Snow measurement has never been so fast and accurate: ETH Zurich researchers have developed an artificial intelligence capable of determining snow depth across Switzerland using satellite images.
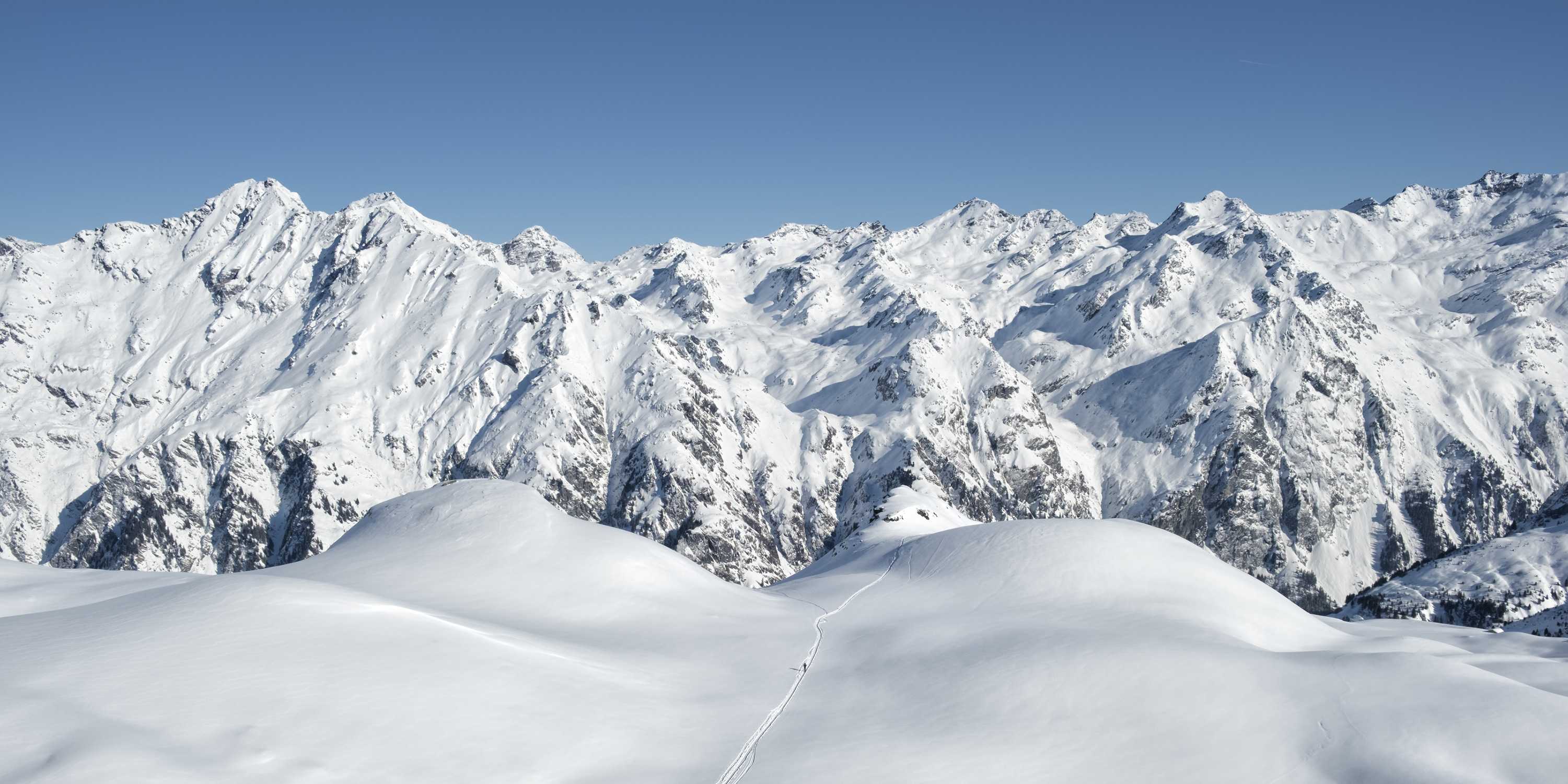
In brief
- ETH Zurich researchers and the Swiss company ExoLabs have developed an AI-assisted snow measurement system capable of determining the daily snow depth more accurately than ever before.
- The use of satellite images means that snow depths can even be updated without always needing to gather new measurement data on the ground.
- Another benefit of the new technology is that it also provides users such as winter sport enthusiasts or operators of hydropower plants with an indication of how certain they can be about the estimate.
How much snow is there up in the mountains, and where exactly is it? The answer is of just as much interest to the winter tourism industry and operators of hydropower plants as it is to winter sport enthusiasts looking to gauge the risk of avalanches. But measuring snow depth is a challenging task for a host of reasons: it can change fast depending on the weather, it varies greatly according to terrain and is not immediately discernible on overhead-images.
Today, snow monitoring in Switzerland is based mainly on data provided by meteorological stations. But as there are only around 400 of these in the entire country, snow data for many places is rather imprecise. This is now set to change: ETH researchers led by Konrad Schindler, Professor of Photogrammetry and Remote Sensing at ETH Zurich, teamed up with the Swiss company ExoLabs, a University of Zurich spin-off, to develop a technology that uses satellite images and artificial intelligence to determine snow depth faster and more accurately than before.
"While the best snow maps currently available for Switzerland have an effective resolution of around 250 by 250 metres, our maps allow the viewer to zoom in to 10 by 10 metres to read the snow depth," Schindler says. Moreover, providing regular updates of snow depth no longer hinges on gathering new measurement data on the ground - in fair weather, publicly available satellite images are sufficient.
Satellite data provided by the European Space Agency
Schindler's research group has plenty of experience with satellite images. It uses them to predict population density in crisis areas, to determine war damage to buildings in Ukraine and to measure the height of forests around the world. But how does an artificial intelligence read snow depth off satellite images?
First, it needs millions of examples: for their technology, the researchers used optical and infrared images taken by Sentinel-2 satellites operated by the European Space Agency (ESA). These satellites capture every location on Earth every five days with a resolution of up to 10 by 10 metres per pixel, making them the most detailed images currently available free of charge and accessible by everyone. By examining these images, the AI can tell when and where Switzerland has snow on the ground and how the snow line changes from week to week.
But that alone is not enough, Schindler says: "Just looking at the white bits on the satellite images doesn't immediately tell us how deep the snow is. For that, we need more data."
Learning by reality comparisons
In addition to the satellite images, the researchers therefore also fed their AI data on Switzerland's terrain. This is because more snow will melt on a steep south-facing hillside than in a shady hollow. Detailed terrain data of this kind is readily available from the public data published by swisstopo, the Swiss Federal Office of Topography.
The researchers trained their AI system to determine the snow depth based on this combination of satellite and terrain data. This involved asking the system to estimate the depth of the snow and then comparing the results with real measurements. "For each grid point, we note how far off the AI estimate was and gradually tweake the system so that the errors became smaller," Schindler explains. The technical term for this is supervised learning.
For the first round of training, the ETH researchers used snow maps provided by ExoLabs, which matched up very well with the snow data from the Swiss meteorological stations. These maps use images not only from Sentinel-2 satellites but also from other satellite missions - which offer lower spatial accuracy but do provide daily images. Using the snow maps from ExoLabs, the AI was able to learn the detailed snow distribution patterns that cannot be captured by the rather loose-knit network of meteorological stations.
Fine-tuning using data from Dischma
The AI was then fine-tuned using extremely detailed snow data, which the Swiss Federal Institute for Forest, Snow and Landscape Research WSL collects only in the Dischma valley in eastern Switzerland. From this data, the AI learned how snow depths can change within just a few metres depending on the terrain. It can now apply these spatial relationships across Switzerland and produce accurate snow depth estimates even for those places where no detailed measurement data is available.
Another benefit of the new technology is that it also provides users with an indication of how certain they can be about the estimate. For example, if the weather has been overcast for a while and new satellite images provide no usable data, the uncertainty of the estimate increases.
New standard for measuring snow depth
The ETH researchers have already successfully tested their AI-assisted snow depth measurement system during two winters. "We expect that this will set a new standard for measuring snow depths in Switzerland," Schindler says.
ExoLabs is taking care of marketing the technology. The Swiss start-up offers high-resolution snow maps through various apps, including those of Outdooractive, Strava, Skitourenguru, Hüttenbuch and swisstopo. Reik Leiterer, CEO of ExoLabs, believes that in the future, enhanced snow maps will also be available for regions other than the Alps, such as Scandinavia, the Pyrenees and North and South America.