The recently completed AGROS validation trial is taking researchers another step closer to the realisation of a fully autonomous greenhouse. In the trial, an Artificial Intelligence algorithm based on Reinforcement Learning controlled the climate in a semi-commercial greenhouse resulting in a productive cucumber crop. Another milestone was marked by the successful deployment of a Digital Twin, which controlled greenhouse climate, irrigation strategy, and crop management. Anja Dieleman, AGROS project leader and researcher at Wageningen University & Research's business unit Greenhouse Horticulture, shares a summary of the successful validation trial and its results.
"In the past two years, we worked on the building blocks for autonomously controlled cultivation of cucumbers. We determined the plant traits essential to decision-making for crop management and climate control, and chose the sensors to measure said traits. To control the greenhouse autonomously, we developed algorithms based on two approaches: a mechanistic model-based Digital Twin and a machine learning algorithm based on Reinforcement Learning," explains Anja. Early this year saw the start of the validation trial: these two approaches and a growers reference were applied in a greenhouse trial with real cucumber crops to evaluate their performance at the WUR research facilities in Bleiswijk (the Netherlands).
- Unfortunately, your cookie settings do not allow videos to be displayed. - check your settings
The objective was to reach the highest possible net profit, which was determined by the balance between variable costs (electricity, natural gas, CO2) and benefits (number of harvested cucumbers, depending on fruit weight). On May 11, the last cucumbers were harvested in the greenhouse compartments of the AGROS validation trial.
Production in growers compartment matches
prediction
The climate control and crop management in the 'growers' compartment was based on current grower knowledge and best practices, with a pre-defined cultivation and irrigation strategy which aimed to realise balanced crop growth and production. The basis of the strategy was the crop's demand for assimilates, provided by sunlight, additional LED lighting, and the temperature and CO2 supply strategy. The irrigation strategy resulted in a crop with high growth at the start of cultivation. When the first flowers appeared, the fruit pruning strategy was determined based on the number of newly formed leaves and the expected light levels. The experts' concept was to grow a vigorous crop, with the assumption that its production would compensate for the costs of electricity, heat, and CO2. "The number of fruits harvested very closely matched the predicted yield, which was based on the cultivation plan that was made at the start of the trial. It shows the robustness of the plan. This control strategy proved to be favourable: the net profit in the growers compartment was the highest," says Anja.
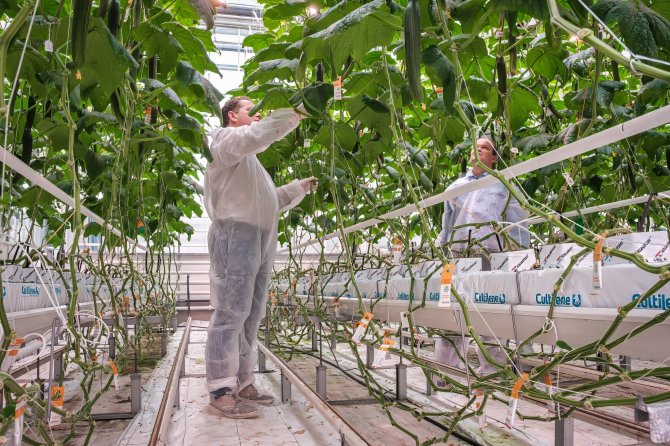
Closest to autonomous cultivation in commercial practice
The Digital Twin that controlled the second compartment was generated by the combined crop and climate model of the business unit Greenhouse Horticulture. In this close-to-real environment, the Digital Twin determined the ideal control strategy based on the responses of the simulated climate and virtual cucumber crop. It used real-time data from climate sensors and manual crop measurements to 'self-calibrate' and improve its control strategy during the trial. The Digital Twin realised an objective control strategy, in which it balanced actual costs against expected benefits: the cucumbers that would be harvested in the next two weeks. Although the number of harvested cucumbers was lower, the Digital Twin made optimal use of variations in electricity prices, resulting in the lowest price per kWh of electricity used. Anja: "Looking at the near future, the Digital Twin is closest to the application of autonomous cultivation in commercial practice. It can take decisions objectively in complex greenhouse production systems, balancing variable resource costs and product prices."
Pioneering fully autonomous greenhouse control with Reinforcement Learning
In the third greenhouse compartment, the climate was controlled by a Reinforcement Learning (RL) algorithm that was trained on virtual data sets of cucumber crops and climate. "AI application in greenhouse horticulture is still in its infancy, and this was one of the first times a greenhouse was controlled fully autonomously by a Reinforcement Learning algorithm," says Anja. The model could control actuators like lighting, screen use, CO2 concentration, and heating, but was not trained to control irrigation and fruit pruning. In the first three weeks of cultivation, control was copied from the 'growers' compartment, and when the first fruits were set, the RL took over. This 'black box' model resulted in a greenhouse climate that differed considerably from the other two compartments, but the crop proved to be able to cope well with the larger fluctuations in temperature. The result was good fruit production, even with the limitations of a pre-set fruit pruning strategy.
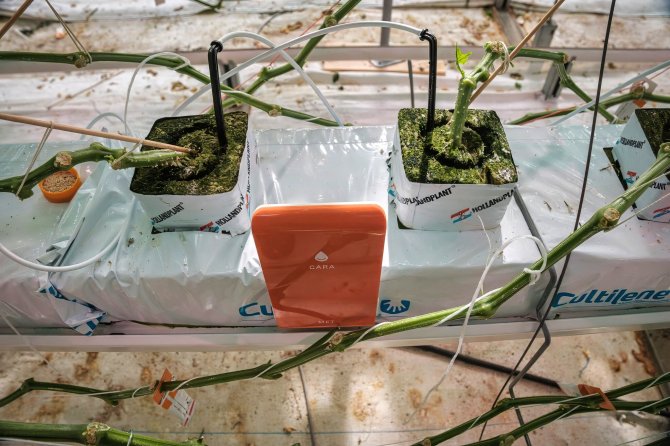
Incorporating sensors into the control loop
"Ideally, all controls would have relied on climate and crop sensors providing continuous, automated, and objective data. However, in this validation trial, we still based control on manual measurements to ensure that the control systems would not be hampered by system failures. The next step in the development of autonomous greenhouse control is to incorporate sensors into the control loop, which would require robust sensors, supported by soft sensor-based solutions," concludes Anja.
Greenhouse horticulture plays an important role in the year-round production of fresh and healthy fruit and vegetables. Nowadays, the horticultural sector faces a number of challenges. A major one is the limited availability of highly qualified and experienced staff that can oversee all aspects of the production system. Other challenges are the rising costs of resources, such as electricity, natural gas, CO2, and fertilisers. The increasing complexity of the production system necessitates the step towards a more data-driven approach. In the public-private partnership project AGROS, we work on the realisation of an 'autonomous greenhouse' in which cultivation is controlled remotely by intelligent algorithms, based on data from non-invasive crop, climate and substrate sensors.
'AGROS: towards an autonomous greenhouse' is a public-private partnership project and a cooperation of the business unit Greenhouse Horticulture of Wageningen University & Research with 2Grow, BASF Vegetable Seeds, Delphy, Gennovation, Greenport West Holland, Hortilux, IMEC/One Planet, Mechatronix, Philips, Ridder, Roullier, Saint-Gobain Cultilene, Signify, Stichting Kennis in je Kas, and Van der Hoeven. Funding is provided by the government "Top Sector" program Horticulture & Starting Materials.