Professor Jaejun Yoo and his research team from the Graduate School of Artificial Intelligence at UNIST recently presented their pioneering work on the future of artificial intelligence (AI) technology at the European Conference on Computer Vision (ECCV) 2024, one of the premier international conferences in the computer vision field, held biennially. ECCV serves as a gathering place for researchers from around the world to share their research results, exchange information, and discuss the future of computer vision industries and technologies. At this prestigious forum, the team showcased three significant research papers that highlight innovative achievements in enhancing AI performance, reducing model sizes, and automating design processes using multimodal AI techniques.
① Compact AI Models: Maintaining Performance Even When Reduced by 323 Times
Figure 1-1. A schematic overview of the two innovative techniques, DiME and NICKEL.
One of the major accomplishments involves the compression of Generative Adversarial Networks (GANs) for image generation by an astounding factor of 323, all while maintaining performance quality. By employing knowledge distillation techniques, the researchers demonstrated the potential for efficient AI utilization even on edge devices or low-power computers, eliminating the need for high-performance computing resources.
Figure 1-2. Comparison of stability of their and state-of-the-art compression methods.
Professor Yoo remarked, "Our research has proven that a GAN compressed by 323 times smaller can still generate high-quality images comparable to existing models. This breakthrough paves the way for deploying high-performance AI in edge computing environments and on low-power devices." The first author, Yeo Sang-yeop, added, "We aim to significantly broaden the scope of AI applications by enabling the implementation of high-performance AI capabilities with limited resources."
The team introduced two innovative techniques, the Distribution Matching for Efficient compression (DiME) and the Network Interactive Compression via Knowledge Exchange and Learning (NICKEL), designed to enhance model stability by comparing distributions rather than evaluating images individually. The NICKEL approach optimizes the interaction between the generator and the classifier, enabling the maintenance of high performance in a lightweight model. The combination of these techniques allowed the compressed GAN model to continue producing high-quality images similar to those generated by larger counterparts.
② High-Resolution Video Generation Without High-Performance Computing
Figure 2-1. Visualization of 3D wavelet transform. The volume of video is decomposed into eight subband (xlll, . . . , xhhh) including low and high frequency components.
In another significant advancement, Professor Yoo and his team developed a hybrid video generation model, HVDM, capable of efficiently producing high-resolution videos even in environments with limited computational resources. By integrating a 2D triple-lane representation with a 3D wavelet transformation, HVDM adeptly processes both global context and intricate details within images.
Figure 2-2. Visualization of 3D wavelet transform. The volume of video is decomposed into eight subband (xlll, . . . , xhhh) including low and high frequency components.
While existing video generation models have relied heavily on high-performance computing resources, HVDM successfully implements natural, high-quality images, overcoming the limitations associated with traditional CNN-based autoencoder methods.
Figure 2-3. Qualitative reconstruction results on SkyTimelapse dataset.
The researchers validated HVDM's superiority through rigorous testing on benchmark video datasets, including UCF-101, SkyTimelapse, and Tai Chi, where HVDM consistently demonstrated higher quality videos and realistic details.
Figure 2-4. Generated video sample by their HVDM on UCF-101, SkyTimelapse, and TaiChi datasets.
Professor Yoo emphasized, "HVDM represents a transformative model that can efficiently generate high-resolution videos, even in resource-constrained environments, with applications extending widely across industries such as video production and simulation."
③ AI for Web UI and Advertising Design: Streamlining the Creation Process
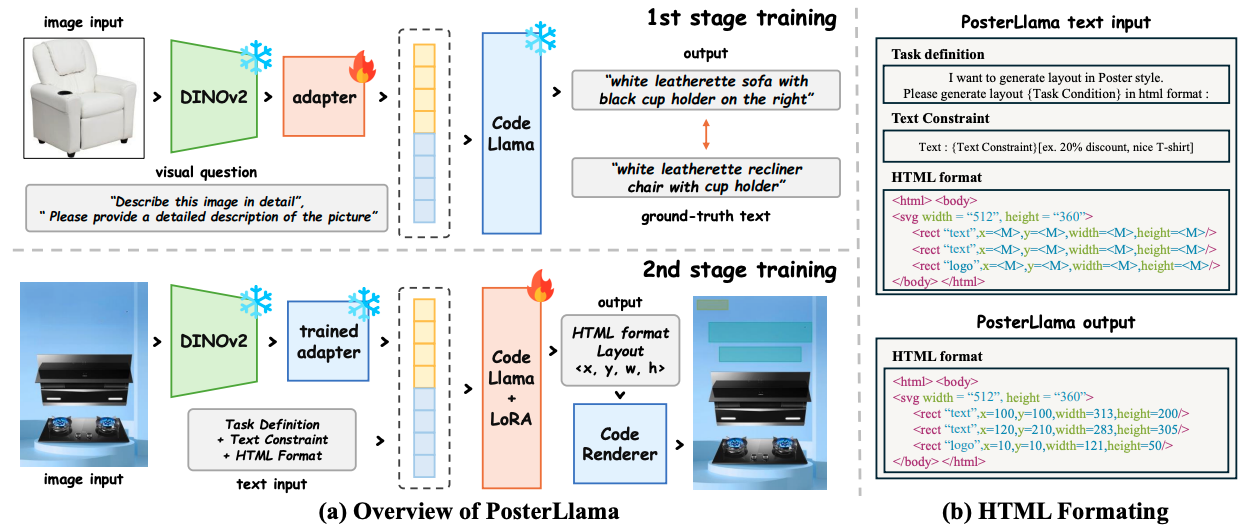
Figure 3-1. The overall training step of PosterLlama.
The research team also introduced a multi-modal layout generation model designed to automate the production of advertising banners and web UI layouts with minimal data input. This model processes images and text simultaneously, generating appropriate layouts based solely on user input.
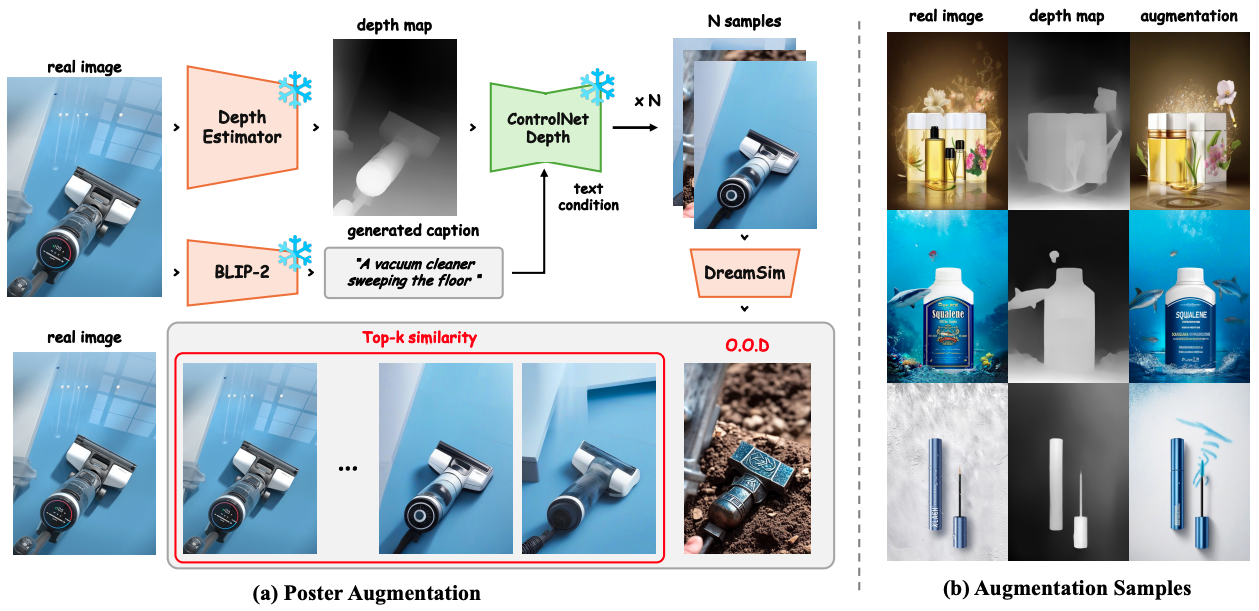
Figure 3-2. The illustration of depth-guided poster augmentation.
Previous models have struggled to adequately integrate text and visual information due to limited data resources. The new model addresses this limitation, significantly enhancing the practicality of advertising design and web UI creation. By maximizing the interaction between text and images, it automatically produces optimized designs that seamlessly reflect both visual and textual elements.
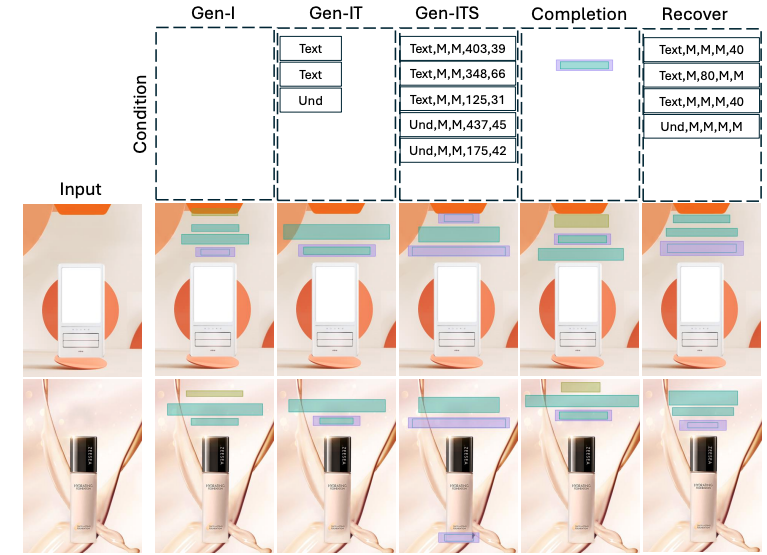
Figure 3-3. Visualization of PosterLlama across five conditional generations, with the conditions for layout generation positioned at the top.
To enable this functionality, the team transformed layout information into HTML code. Leveraging extensive pre-training data from language models, they established an automated generation pipeline that yields exceptional results, even with sparse datasets. Benchmark evaluations revealed performance improvements of up to 2,800% compared to existing methodologies.
In the pre-training process, the team utilized the image caption dataset, combining Depth-Map and ControlNet techniques to enhance performance through data augmentation. This approach significantly improved the quality of layout generation and created natural designs by reducing potential distortions that may occur during data preprocessing.
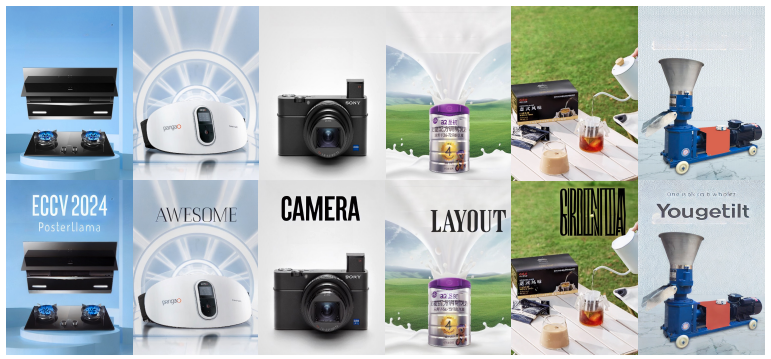
Figure 3-4. The example of proposed poster generation pipeline.
"Our model outperforms existing solutions that require over 60,000 data points, showing effective results with as few as 5,000 samples," noted Professor Yoo. "This innovation is accessible not only to experts but also to everyday users, signaling significant advancements in the automation of advertising banners and web UI design."
This research was conducted with support from the National Research Foundation of Korea (NRF), the Ministry of Science and ICT (MSIT), the Institute of Information and Communication Planning and Evaluation (IITP), and UNIST. The findings are anticipated to expand the applicability of AI across various industries, while maximizing performance and efficiency.
Journal Reference
1. Sangyeop Yeo, Yoojin Jang, Jaejun Yoo, "Nickel and Diming Your GAN: A Dual-Method Approach to Enhancing GAN Efficiency via Knowledge Distillation," arXiv:2405.11614 [cs.CV], (2024).
2. Kihong Kim, Haneol Lee, Jihye Park, et al., "Hybrid Video Diffusion Models with 2D Triplane and 3D Wavelet Representation," arXiv:2402.13729 [cs.CV], (2024).
3. Jaejung Seol, Seojun Kim, Jaejun Yoo, "PosterLlama: Bridging Design Ability of Langauge Model to Contents-Aware Layout Generation," arXiv:2404.00995 [cs.CV], (2024).