QUT researchers who took an Artificial Intelligence (AI) system on a south-east Queensland road trip have identified the key role high-definition annotated maps will likely play in autonomous driving on Australian roads.
Professor Michael Milford, deputy director of QUT's Centre for Robotics, led the research team which took an electric car fitted with high-tech sensors on a 1200km road trip over three months last year.
"The primary goal of the study was to consider how current advances in robotic vision and machine learning – the backbone of AI – could enable the research car platform to see and make sense of everyday road signage and markings that we, as humans, take for granted," Professor Milford said.
The research findings highlight the limitations camera-vision systems have in correctly identifying and interpreting signs, traffic lights and lines on the roads.
It also highlights potential infrastructure issues for automated vehicles, such as obstructed signs, inclement weather, road works and driving at night.
Professor Milford said the study had found the most critical factor in improving the effectiveness of cameras with autonomous vehicle navigation systems was the implementation of "prior maps", which are high-definition maps annotated with details such as exact lane locations, traffic signs, and other data about the rules of the road.
Without a map, the camera vision systems only detected about 40 per cent of signs such as speed, give way, no right turn, pedestrian crossing and speed hump, with that accuracy rate even lower on rural roads and motorways.
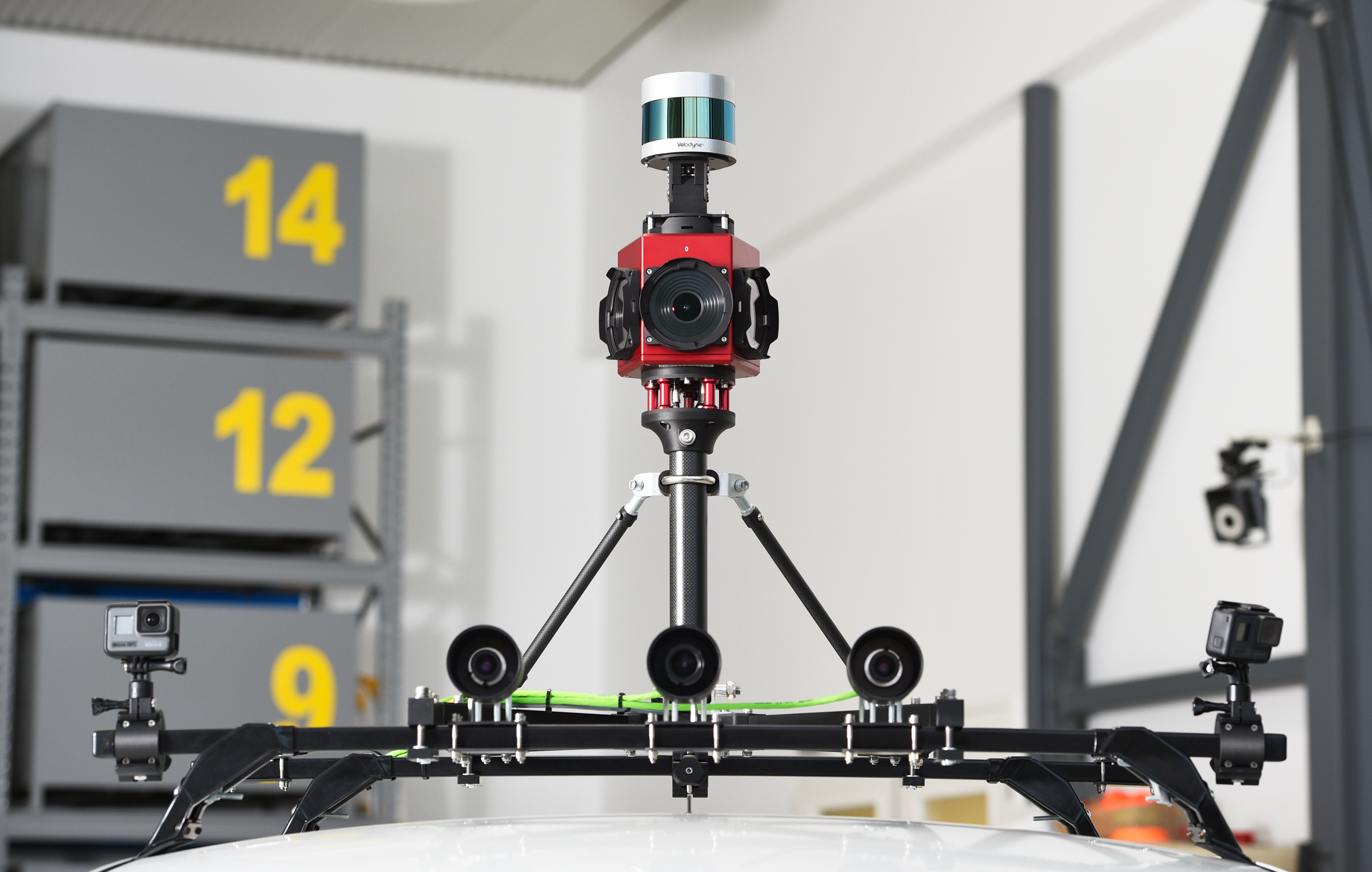
The camera vision systems also falsely detected many signs when there were none, or wrongly identified them, struggled to identify lane markings or cracked or wet roads.
When the navigation system used a combination of imagery from the cameras and high-definition maps, the accuracy rate for correctly identifying signs jumped to more than 97 per cent.
"This finding is consistent with the majority of approaches that use prior maps, with a few notable exceptions," Professor Milford said.
"It is likely that these map systems will need to have real-time updates on temporary obstructions and changes to signs and roads, and to ensure the navigation systems used by autonomous vehicles receive those updates instantly."
The project, which is part of the Cooperative and Highly Automated Driving (CHAD) Pilot run in partnership with Queensland Department of Transport and Main Roads and iMOVE Cooperative Research Centre, involved a driver taking an electric Renault fitted with high-tech sensors and computers on a wide range of road and driving conditions.
Transport and Main Roads Minister Mark Bailey said the road trip in an electric Renault ZOE assessed Queensland's road infrastructure in preparation for automated vehicles, results of which have now been published online.
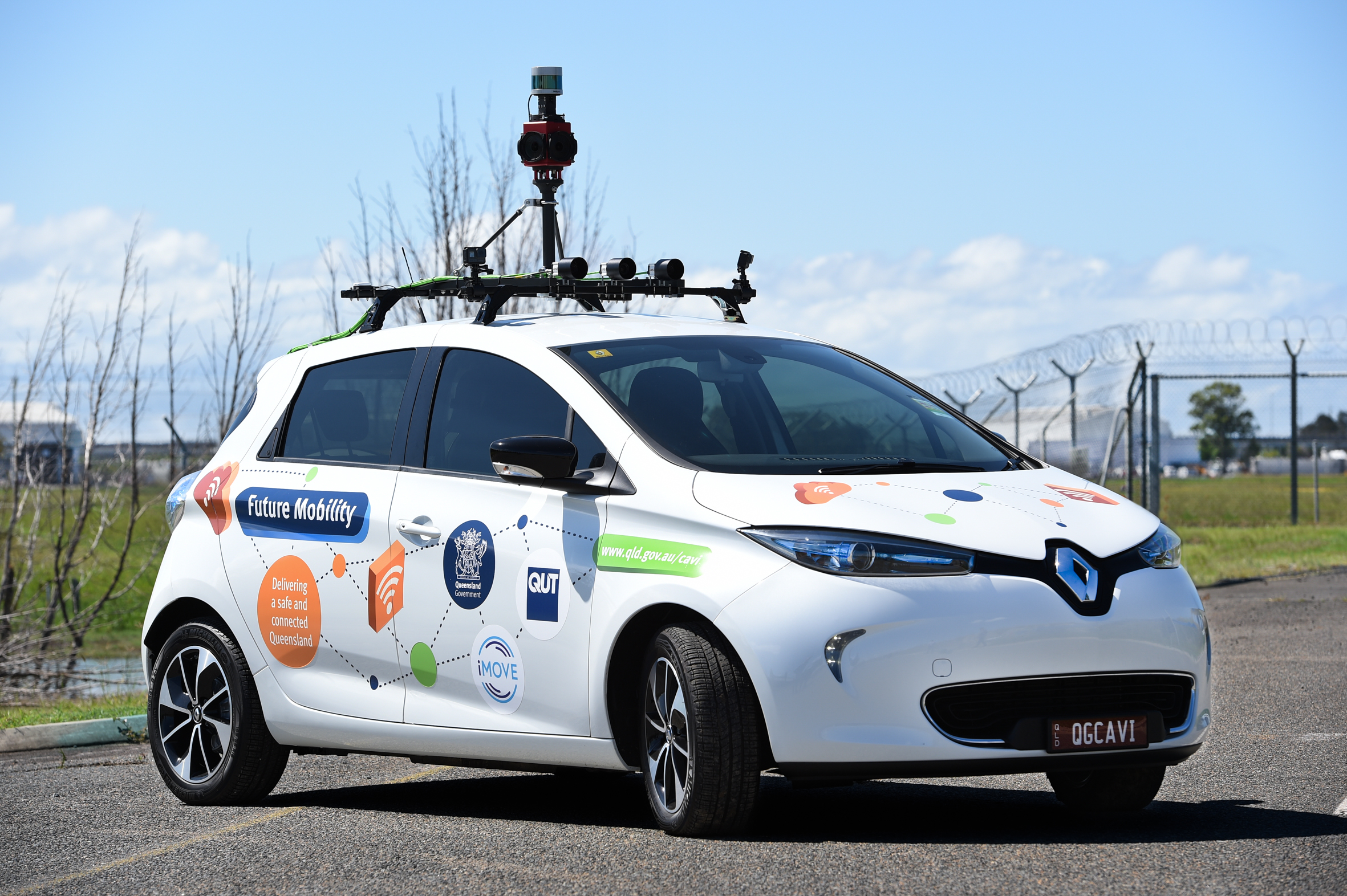
"This study was the first step in understanding infrastructure requirements of our vast and varied road network for new vehicle technologies," Mr Bailey said.
"As researchers drove the car across South East Queensland, onboard sensors collected some 20 terabytes of raw data which was used to train and refine AI algorithms.
"Artificial Intelligence technology and smart road infrastructure have the potential to transform the way we travel in Queensland and reduce road trauma.
"The study results demonstrate that we need more research like this to ensure we have the right systems in place to meet the coming demand for different and emerging transport options."
Professor Milford is also a Chief Investigator at the Australian Research Council Centre of Excellence for Robotic Vision, headquartered at QUT.