Radiologists are beginning to use AI-based computer vision models to help speed up the laborious process of parsing medical scans. However, these models require large amounts of carefully labeled training data to achieve consistent and accurate results, meaning radiologists must still dedicate significant time to annotating medical images.
An international team led by Johns Hopkins Bloomberg Distinguished Professor Alan Yuille has a solution: AbdomenAtlas, the largest abdominal CT dataset to date, featuring over 45,000 3D CT scans of 142 annotated anatomical structures from 145 hospitals worldwide—over 36 times larger than its closest competitor, TotalSegmentator V2. The dataset and its implementations appear in a recent issue of Medical Image Analysis.
Key Takeaways
- Computer scientists used AI to create the largest abdominal organ dataset yet in under two years-a task that would have taken two millennia for humans alone
- The dataset will help researchers around the world train AI algorithms to identify cancer and other diseases without overburdening radiologists
Previous abdominal organ datasets were compiled by radiologists manually identifying and labeling individual organs in CT scans, requiring thousands of hours of human labor.
"Annotating 45,000 CT scans with 6 million anatomical shapes would require an expert radiologist to have started working around 420 BCE—the era of Hippocrates—to complete the task by 2025," says lead author Zongwei Zhou, an assistant research scientist in the Whiting School of Engineering's Department of Computer Science.
Addressing this monumental challenge, the Hopkins-led team used AI algorithms to dramatically accelerate this organ-labeling task. Working with 12 expert radiologists and additional medical trainees, they completed in under two years a project that would have taken humans alone over two millennia.
The researchers' method combines three AI models trained on public datasets of labeled abdominal scans to predict annotations for unlabeled datasets. Using color-coded attention maps to highlight areas needing refinement, the method identifies the most critical sections of the models' predictions for manual review by radiologists. By repeating this process—AI prediction followed by human review—they significantly accelerate the annotation process, achieving a 10-fold speedup for tumors and 500-fold for organs, the researchers say.
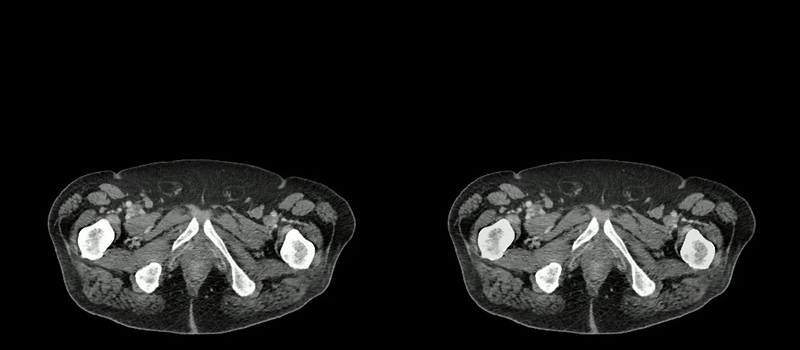
Image caption: Two series of abdominal CT scan slices, standard on the left and AbdomenAtlas' organ segmentation on the right
Image credit: Johns Hopkins University
This approach enables the team to expand the scope, scale, and precision of their dataset without overburdening radiologists, resulting in what the team says is the largest fully annotated abdominal organ dataset in existence. They continue to add more scans, organs, and both real and artificial tumors to help train new and existing AI models to identify cancerous growths, diagnose diseases, and even create digital twins of real-life patients.
"By enabling AI models to learn more about related anatomical structures before training on data-limited domains—such as in tumor identification—we have made AI perform similar to the average radiologists in some tumor detection tasks," reports first author Wenxuan Li, a graduate student of computer science advised by Yuille.
AbdomenAtlas also serves as a benchmark that allows other research groups to evaluate the accuracy of their medical segmentation algorithms. The more data that's used to test these algorithms, the better their reliability and performance can be guaranteed in complex clinical scenarios, the Hopkins researchers say.
The team has committed to eventually releasing AbdomenAtlas to the public and posing new medical segmentation challenges using it, such as the BodyMaps challenge at the 27th International Conference on Medical Image Computing and Computer Assisted Intervention last October. This challenge aimed to encourage AI algorithms that not only perform well theoretically but also those that are practically efficient and reliable in clinical settings.
Despite the advancements made possible by AbdomenAtlas, its creators note that the dataset only accounts for 0.05% of the CT scans annually acquired in the United States—and call upon other institutions to help fill in the gaps.
"Cross-institutional collaboration is crucial for accelerating data sharing, annotation, and AI development," the researchers write. "We hope our AbdomenAtlas can set the stage for larger-scale clinical trials and offer exceptional opportunities to practitioners in the medical imaging community."