The quadrupedal robot ANYmal went back to school and has learned a lot. ETH Zurich researchers used machine learning to teach it new skills: the robot can now climb over obstacles and successfully negotiate pitfalls.
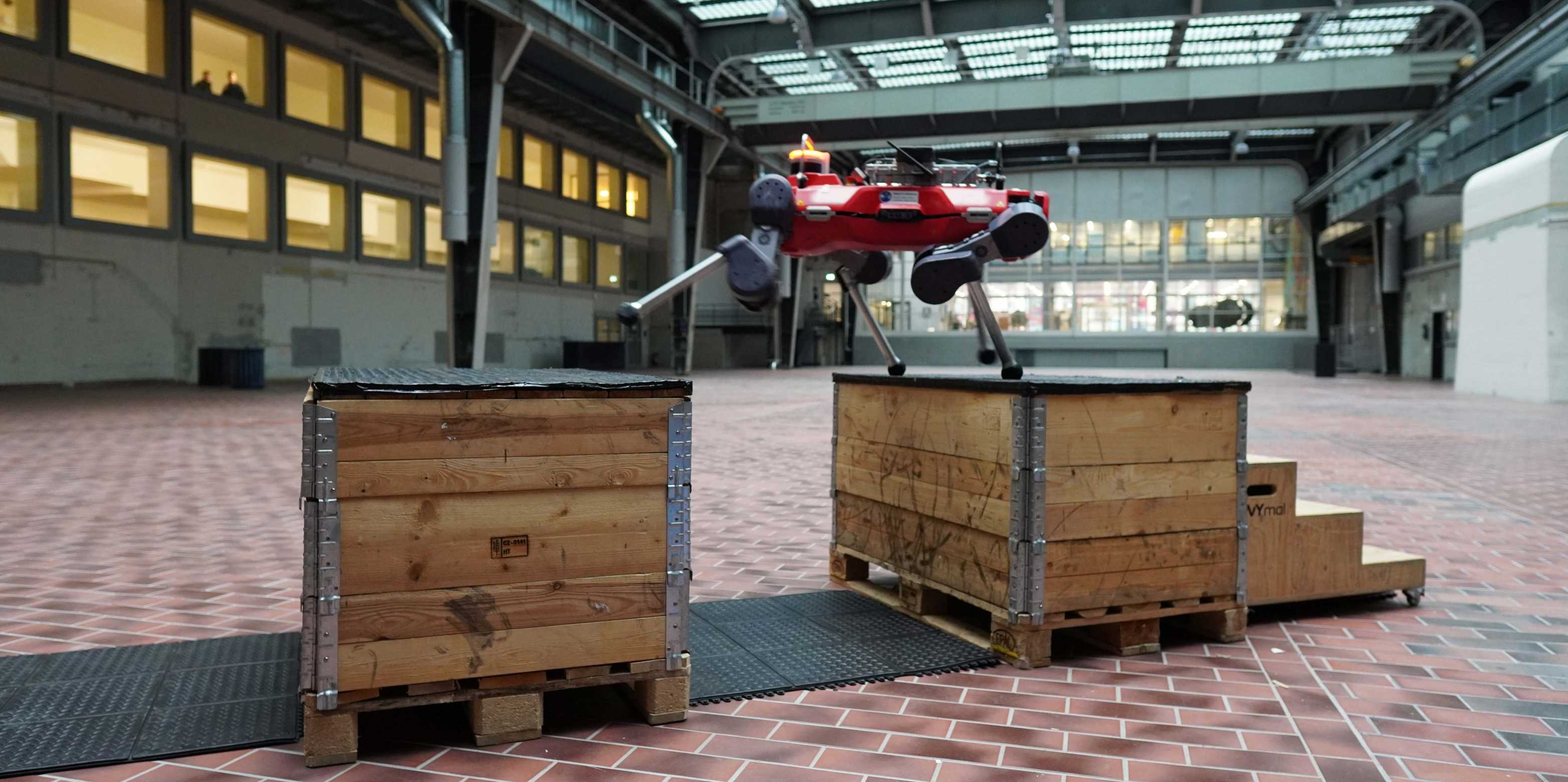
In brief
- Using machine learning, quadrupedal robot ANYmal learns like a child by means of trial and error. In this way it taught itself to do parkour.
- With the aid of a camera and an artificial neural network, it detects and overcomes obstacles.
- Through the combination with classic model-based control, the robot can negotiate difficult terrain.
ANYmal has for some time had no problem coping with the stony terrain of Swiss hiking trails. Now researchers at ETH Zurich have taught this quadrupedal robot some new skills: it is proving rather adept at parkour, a sport based on using athletic manoeuvres to smoothly negotiate obstacles in an urban environment, which has become very popular. ANYmal is also proficient at dealing with the tricky terrain commonly found on building sites or in disaster areas.
To teach ANYmal these new skills, two teams, both from the group led by ETH Professor Marco Hutter of the Department of Mechanical and Process Engineering, followed different approaches.
Exhausting the mechanical options
Working in one of the teams is ETH doctoral student Nikita Rudin, who does parkour in his free time. "Before the project started, several of my researcher colleagues thought that legged robots had already reached the limits of their development potential," he says, "but I had a different opinion. In fact, I was sure that a lot more could be done with the mechanics of legged robots."
With his own parkour experience in mind, Rudin set out to further push the boundaries of what ANYmal could do. And he succeeded, by using machine learning to teach the quadrupedal robot new skills. ANYmal can now scale obstacles and perform dynamic manoeuvres to jump back down from them.
In the process, ANYmal learned like a child would - through trial and error. Now, when presented with an obstacle, ANYmal uses its camera and artificial neural network to determine what kind of impediment it's dealing with. It then performs movements that seem likely to succeed based on its previous training.
Is that the full extent of what's technically possible? Rudin suggests that this is largely the case for each individual new skill. But he adds that this still leaves plenty of potential improvements. These include allowing the robot to move beyond solving predefined problems and instead asking it to negotiate difficult terrain like rubble-strewn disaster areas.
Combining new and traditional technologies
Getting ANYmal ready for precisely that kind of application was the goal of the other project, conducted by Rudin's colleague and fellow ETH doctoral student Fabian Jenelten. But rather than relying on machine learning alone, Jenelten combined it with a tried-and-tested approach used in control engineering known as model-based control. This provides an easier way of teaching the robot accurate manoeuvres, such as how to recognise and get past gaps and recesses in piles of rubble. In turn, machine learning helps the robot master movement patterns that it can then flexibly apply in unexpected situations. "Combining both approaches lets us get the most out of ANYmal," Jenelten says.
As a result, the quadrupedal robot is now better at gaining a sure footing on slippery surfaces or unstable boulders. ANYmal is soon also to be deployed on building sites or anywhere that is too dangerous for people - for instance to inspect a collapsed house in a disaster area.
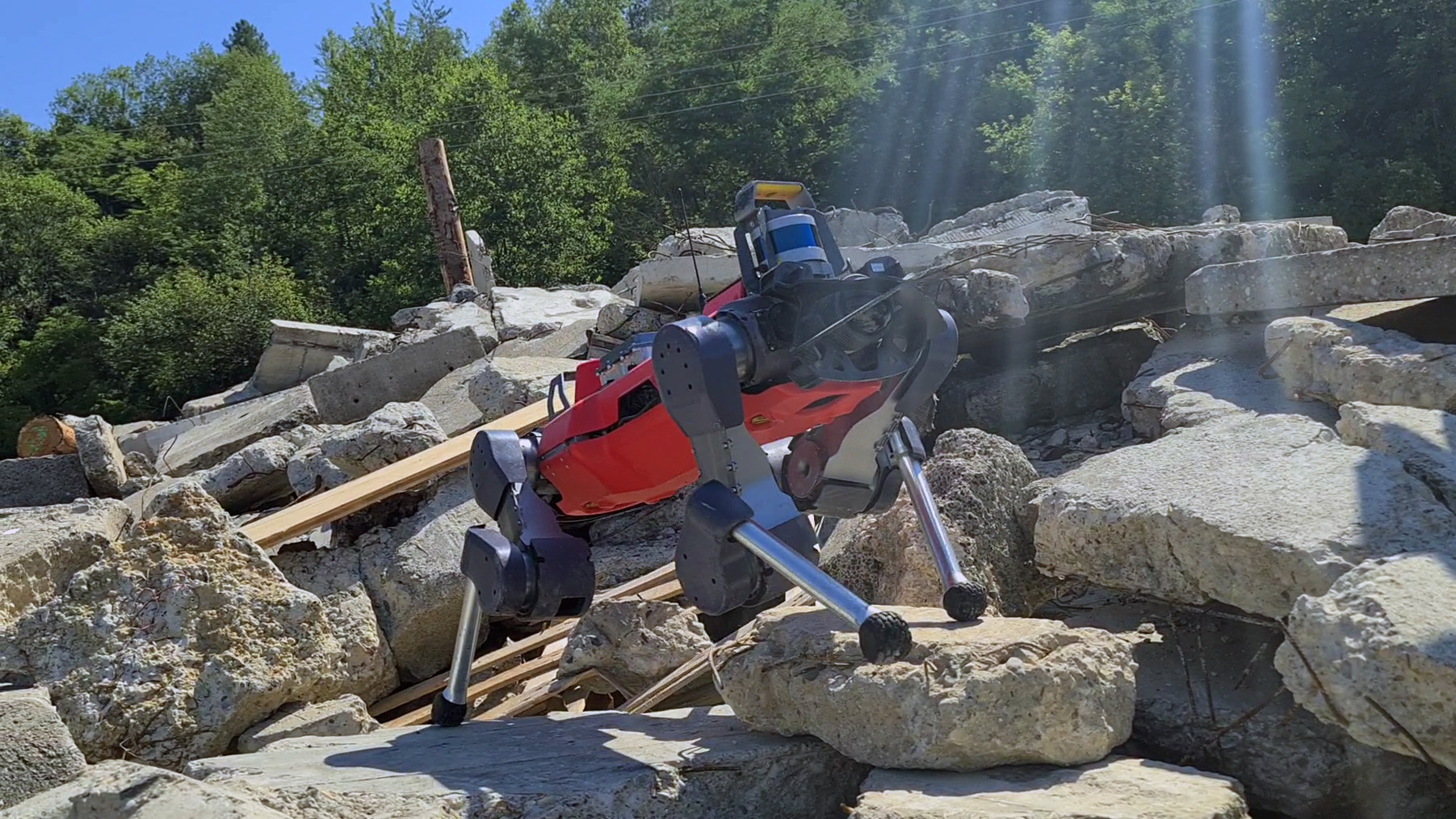
References
Hoeller D, Rudin N, Sako D, Hutter M: ANYmal Parkour: Learning Agile Navigation for Quadrupedal Robots, Science Robotics, 13 March 2024, doi: external page10.1126/scirobotics.adi7566
Jenelten F, He J, Farshidian F, Hutter M: DTC: Deep Tracking Control. Science Robotics 2024, 17: eadh5401, doi: external page10.1126/scirobotics.adh5401