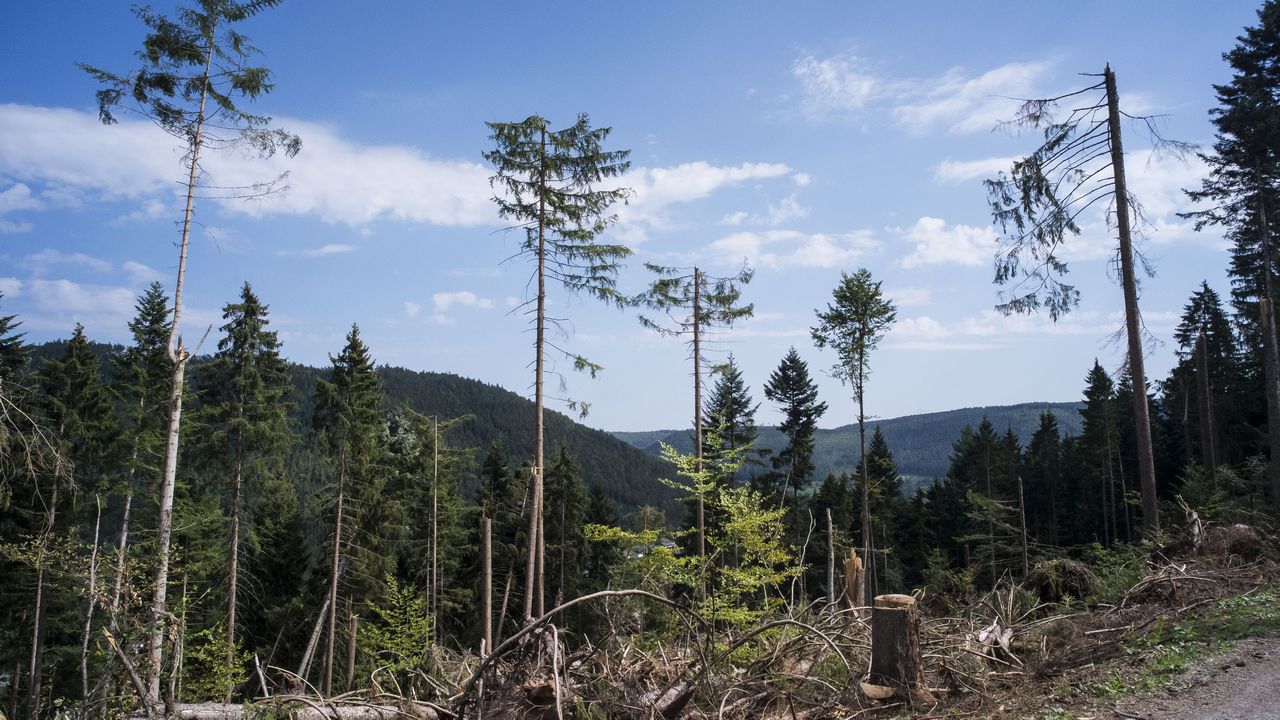
Better protection of humans and the environment requires precise forecasts of extreme weather phenomena, such as winter storms. Researchers of Karlsruhe Institute of Technology (KIT) have now compared methods of statistics and machine learning for forecasts of wind gusts with a view to make the forecasts more accurate and reliable. They found that taking into account geographical information and additional meteorological variables, such as temperature, significantly improves the forecast quality, in particular when using modern AI methods based on neural networks.
Strong wind gusts, such as squalls with a speed of more than 65 kilometers per hour, may cause severe damage and be dangerous for humans, animals, and infrastructure facilities. To issue effective warnings, early and reliable forecasts are required. "Wind gusts are difficult to model, as they are driven by small-scale processes and are locally limited," says Benedikt Schulz, doctoral researcher at KIT's Institute of Stochastics. "Their predictability with the numerical weather forecast models used by weather services is limited and subject to uncertainties."
To better estimate such uncertainties of forecasts, meteorologists make ensemble forecasts. Based on the current state of the atmosphere, they make several model calculations in parallel for slightly different conditions. In this way, various future weather development scenarios are covered. "In spite of continuous improvements, however, such ensemble weather forecasts still have systematic errors, as local temporally varying conditions cannot be considered by the models," Schulz explains. "With the help of artificial intelligence, we want to correct these systematic errors, improve the forecasts, and more reliably predict dangerous weather phenomena."
Geographical Information and Additional Meteorological Variables Improve the Forecast of Wind Gusts
Together with Dr. Sebastian Lerch, Schulz for the first time compared different statistics and AI methods for the post-processing of ensemble forecasts of wind gusts. "We analyzed both existing and novel methods for statistical post-processing of numerical weather forecasts and systematically compared their forecast qualities," Lerch says. He heads the junior research group "AI Methods for Probabilistic Weather Forecasts" funded by the Vector Foundation at KIT's Institute for Economic Policy Research.
All post-processing methods were found to produce reliable forecasts of the speed of wind gusts. "Yet, AI methods are far superior to classical statistical approaches and produce far better results, as they allow for a better consideration of new information sources, such as geographical conditions or other meteorological variables, such as temperature and solar radiation," Lerch summarizes. "AI methods reduce the forecast errors of weather models by about 36 percent on the average," Schulz adds. The researchers analyzed the forecasts made with the weather model of the German Weather Service (DWD) at 175 observation stations in Germany and found that AI methods produced better forecasts at more than 92 percent of the stations. Neural networks can learn complex and non-linear relationships from the big data sets available. This plays a central role when correcting systematic errors of ensemble forecasts. "Analysis of which information is particularly relevant to the methods also allows conclusions to be drawn with respect to meteorological processes," Schulz says.
With their work, the researchers contribute to the development of weather forecast methods at the interface of statistics and AI. "Weather services might use these methods to improve their forecasts," Lerch says. "For this, we are in close contact with the German and other international weather services."
Supraregional Research for Better Weather Forecasts
The above research is embedded in the collaborative research center/transregio 165 "Waves to Weather" (W2W) coordinated by Ludwig-Maximilians-Universität Munich. It cooperates with KIT and Johannes-Gutenberg-Universität to enhance the accuracy and reliability of weather forecasts. W2W addresses the presently biggest challenge in weather forecast: Identifying the limits of forecasts in different situations and making the physically best possible prognosis.
Original Publication
Benedikt Schulz & Sebastian Lerch: Machine Learning Methods for Postprocessing Ensemble Forecasts of Wind Gusts: A Systematic Comparison. Monthly Weather Review (MWR), 2022. DOI: 10.1175/MWR-D-21-0150.1.
More about the KIT Center Mathematics in Sciences, Engineering, and Economics (MathSEE): https://www.mathsee.kit.edu/english/index.php
Being "The Research University in the Helmholtz Association", KIT creates and imparts knowledge for the society and the environment. It is the objective to make significant contributions to the global challenges in the fields of energy, mobility, and information. For this, about 9,800 employees cooperate in a broad range of disciplines in natural sciences, engineering sciences, economics, and the humanities and social sciences. KIT prepares its 22,300 students for responsible tasks in society, industry, and science by offering research-based study programs. Innovation efforts at KIT build a bridge between important scientific findings and their application for the benefit of society, economic prosperity, and the preservation of our natural basis of life. KIT is one of the German universities of excellence.