Machine learning and multidisciplinary collaboration map 'constellations' of molecules, which may help customise medical treatments for blood cancer
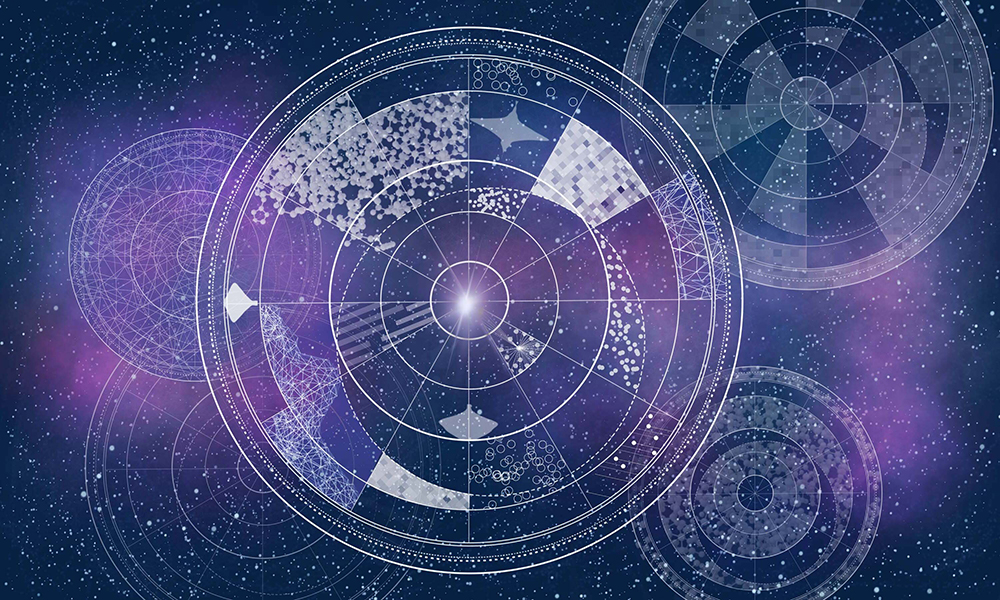
Much like how the earliest astronomers created constellations to make sense of a night sky full of twinkling lights, scientists are mapping the constellations of DNA, proteins, and other molecules in tumours, potentially navigating a way to better customised cancer treatments.
Researchers have long used various kinds of omics technologies to understand the components that biological systems are made of: genomics (sequencing genomes), proteomics (studying the collection of proteins in the tissues of an organism), transcriptomics (studying the RNA), and metabolomics (studying metabolites).
Now, having collected data produced by all these approaches, computational biologists at EMBL have worked closely with biomedical scientists and physicians from the University of Zurich, the University of Heidelberg, the German Cancer Research Centre (DKFZ), and elsewhere to build a machine-learning approach that could help us better understand blood cancer and consequently devise individualised drug treatment plans.
The challenge and opportunity of a 'model' disease
Chronic lymphocytic leukaemia is the most common kind of blood cancer in the western world and generally strikes people in their 60s or older. Its impact varies considerably - even at the cellular level. In some people, the disease is quite aggressive; in others, it can be managed by medication, allowing patients to live a long time.
The most effective medication for any given type of cancer is generally not the same for everyone, which has created a puzzle for physicians who may have to guess which drug might work best in each case. Unfortunately, that means subjecting patients, some of whom are already immunocompromised and frail, to treatments with uncertain outcomes that may also have serious side effects.
That said, the high prevalence of this particular leukaemia and the relative ease of obtaining blood samples makes it a suitable testbed for molecular medicine. It is also one of the most studied leukaemias, which means researchers have significant clinical data to build upon.
EMBL Group Leader and Senior Scientist Wolfgang Huber and his collaborators took this opportunity to create a comprehensive multi-omics survey of this disease. Huber compares their approach to using different kinds of telescopes aimed at the same astronomical object - because they operate with different types of light, they provide different types of information about the object, thus giving a more complete picture.
"We had samples from hundreds of patients, allowing us to see the heterogeneity in the molecular landscape," Huber said. "This allowed us then to map out the constellations of the molecular makeup. The key, however, was to find a 'North Star' - a point that looks the same from all different angles we're looking at it, and enables us to piece the complete picture together."
Multi-omics constellations lead to novel biomarker 'North Star'
And, in fact, this astronomers' approach soon found a common axis line in the otherwise seemingly chaotic molecular universe. By linking data points that trended in the same direction across the multiple omics views, the researchers honed in on a new biomarker that seems to indicate which tumours are more likely to be aggressive.
"We first checked that our algorithm was doing something useful. When we saw that it rediscovered a couple of known things, it gave us confidence that the new effects it found were real," Huber explained. He noted that the multi-omics data allowed the researchers to see something that was previously hidden in the individual omics datasets.
"When you find something like this in a single dataset, it is often not clear whether it's worth following up, as it just may be something too convoluted or a fluke in the measurement. But, in our case, because we could see it from different angles, it was more likely to be something of biological consequence," he added.
A year later, after more detailed experiments by the team of Thorsten Zenz at the University Hospital Zurich, the researchers were sufficiently confident in the meaning of their discovery.
"The next step was boiling down this discovery into a smaller, more practical marker," Huber said. "Once you know what you are looking for, you can build a specific assay that is rapid and cheap enough to deploy in a clinical setting."
It is these accomplishments - discovering and then understanding the biological meaning of this biomarker - that were the focus of a recent paper in Nature Cancer.
"I think one of the most unique and exciting aspects of this project is that we used a machine-learning method to integrate these omics datasets to identify new biology, but in a long-studied and seemingly well-characterised disease," said Junyan Lu, a machine-learning expert in the Huber Group and the lead author of the paper.
"Currently, much machine learning in biomedical research focuses on mining single omics data, like just genomics or transcriptomics, alone," he added. "Multi-omics machine learning enabled us to amplify meaningful and clinically related signals among noisy biological data. This is because important biological changes usually affect several molecular layers and are visible in multiple omics datasets."
Lu performed most of the computational analyses of the omics data, ultimately providing hypotheses for medical collaborators to explore further. "Without the interdisciplinary collaboration with Thorsten Zenz's group, I would not have had the data from patient samples to work with," Lu said. "The input from the clinicians and experimental biologists was crucial for interpreting results and refining our hypothesis."