7 February 2025
Interview with Stefan Kesselheim and Jan Ebert from the Jülich Supercomputing Centre
At the end of January, the Chinese startup DeepSeek published a model for artificial intelligence called R1 - and sent shockwaves through AI world. The model achieves performance comparable to the AI models of the largest US tech companies. And yet, until recently, DeepSeek was a little-known enterprise. The model is said to have cost less than $ 6 million.
Prof. Stefan Kesselheim heads Simulation and Data Lab Applied Machine Learning at the Jülich Supercomputing Centre. He is also head of the Helmholtz AI Consultant team, which supports science and industry in developing customized approaches for machine learning. Together with his colleague and AI expert Jan Ebert, he explains what is so special about the DeepSeek AI model and what makes it different to previous models.
What makes R1 so efficient?
Stefan Kesselheim: DeepSeek-R1 is not an efficient model in itself. The basic model DeepSeek-V3 was released in December 2024. It has 671 billion parameters, making it quite large compared to other models. Good engineering made it possible to train a large model efficiently, but there is not one single outstanding feature.
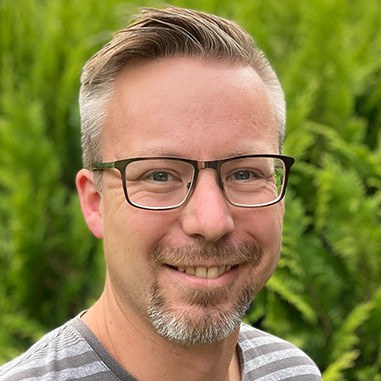
The R1 model published in January builds on V3. The model uses a technique known as reasoning - similar to OpenAI's o1 model. The model uses numerous intermediate steps and outputs characters that are not intended for the user. This is similar to the human thought process, which is why these steps are called chains of thought. This technique makes usage considerably more complex, essentially considerably less efficient, but it improves the results considerably depending on the task. Up to now, only OpenAI and Google were known to have found a comparable solution for this.
Jan Ebert: To train DeepSeek-R1, the DeepSeek-V3 model was used as a basis. The conventional part of training is in DeepSeek-V3. DeepSeek-R1 is basically DeepSeek-V3 taken further in that it was subsequently taught the reasoning techniques Stefan talked about, and learned how to generate a "thought process". When we talk about efficiency, we cannot just talk about R1 alone, we must also include the basic architecture of V3.
Although V3 has a very large number of parameters, a comparatively small number of parameters are actively used to predict individual words (tokens). Parts of the model are automatically selected to generate the best prediction in each case. This technique is known as a "mixture of experts". The community assumes that GPT-4 uses the same technology; other providers are also known to use it. DeepSeek put a lot of effort into this to make it as efficient as possible. Another efficiency improvement underlying V3 is a more efficient comparison between individual words (tokens). However, none of these technologies are new; they were already implemented in earlier DeepSeek models.
To come back to the engineering point raised by Stefan: the DeepSeek-V3 model - and presumably R1 as well - was trained to a lower numerical accuracy than usual. Catastrophic rounding errors therefore had to be avoided on the way to finding a solution. As far as I know, no one else had dared to do this before, or could get this approach to work without the model imploding at some point during the learning process.
In general, comparisons are difficult with models that are kept behind closed doors, such as those of OpenAI or Google, as too little is known about them.
How could DeepSeek develop its AI so quickly and cost-effectively?
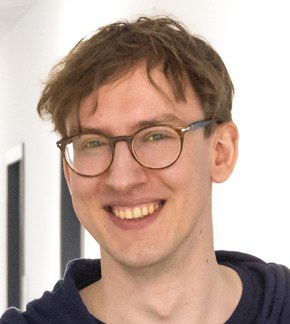
Stefan Kesselheim: DeepSeek published a broad outline of the basic technique for training reasoning in February 2024 when they released DeepSeekMath. The technique is known as Group Relative Policy Optimization and makes it possible to refine AI models - even without using data provided by humans. We are very impressed that this conceptually simple approach represented such a breakthrough. This breakthrough is what made it possible to develop this model in less than a year. The basic model DeepSeekV3 was a natural evolution of its predecessor. Excellent engineering work has been done here.
Jan Ebert: It is also important to mention that DeepSeek has invested a lot of time and money into researching scaling laws. This allowed the team to predict pretty accurately how they would need to scale up the model and data set to achieve the maximum potential. The research on AI models for mathematics that Stefan cited will have laid many important building blocks for the code, which R1 will also have used to automatically evaluate its answers.
Are there fundamental differences between the R1 and European and US models?
Stefan Kesselheim: Based on what we know about DeepSeek-R1, a direct path has been taken here to a strong model, and decisive parts have been made openly available. At this point in time, the DeepSeek-R1 model is comparable to OpenAI's o1 model. Other providers will now also do their utmost to refine their models in a similar way. We expect to see the French company Mistral AI do this for its models, for example. With DeepSeek-R1, however, explicit care was taken to ensure that the model presents certain aspects of Chinese politics and history in a certain way. Such targeted interventions are not currently known in US and European models.
Jan Ebert: That being said, OpenAI is currently facing criticism for training its models to consider human rights issues relating to Palestine separately. Of course, you have to be careful here, because this could also involve automatically learned answers, taken from the gigantic "unmoderated" data set used for training. As an aside, censorship on certain points is prescribed, as far as I understand it, by the Chinese state in an AI law.
The big difference between DeepSeek-R1 and the other models, which we have only implicitly described here, is the disclosure of the training process and the appreciation of and focus on research and innovation. Mistral, for example, occasionally publishes trained models for free use, but the architecture of these models is still very conventional to a large extent. DeepSeek has upped the pace here, and has been doing so for over a year now. With the release of R1, all the differences in DeepSeek's models and training processes have now gained the visibility they deserve.
What can we do to catch up here?
Stefan Kesselheim: DeepSeek has a large team of AI engineers, whose ideas often stand out from the mainstream. The development of Group Relative Policy Optimization most certainly involved many hurdles and probably did not work right away. This explorative way of thinking, which does not focus on immediate commercial success, should inspire AI science more than ever before.
Jan Ebert: We should dare to innovate more. DeepSeek has done a really great job. A clever idea, a good team, and the courage to try something new is what made the difference here. At Jülich, we too are also trying to make our mark in projects like TrustLLM and help further develop large AI models. We are actively shaping the future towards scientific transparency and open source. By the way, you can download some of the DeepSeek models from our evaluation server Blablador and try them out. Unfortunately, we currently lack the resources for the large R1 model.