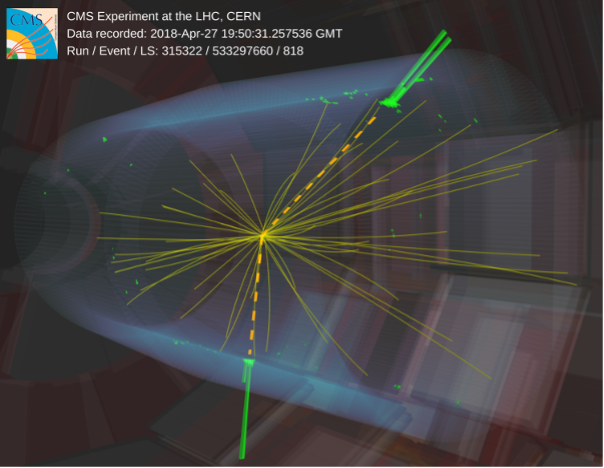
As part of their quest to understand the building blocks of matter, physicists search for evidence of new particles that could confirm the existence of physics beyond the Standard Model (SM). Many of these beyond-SM theories postulate the need for additional partner particles to the Higgs boson. These partners would behave similarly to the SM Higgs boson, for example in terms of their "spin", but would have a different mass.
To search for Higgs partner particles, scientists at the CMS collaboration look for the signatures of these particles in the data collected by the detector. One such signature is when the particles decay from a heavy Higgs partner (X) particle to two lighter partner particles (φ), which in turn each decay into collimated pairs of photons. Photon signatures are ideal to search for particles with unknown masses as they provide a clean, well-understood signature. However, if the φ is very light, the two photons will significantly overlap with each other and the tools usually applied for the photon identification fall apart.
This is where artificial intelligence (AI) comes in. It is well known that machine learning computer vision techniques can differentiate between many faces, and now such AI methodologies are becoming useful tools in particle physics.
The CMS experiment searched for the X and φ partners of the Higgs boson using the hypothetical process X→φφ, with both φ decaying to collimated photon pairs. To do this, they trained two AI algorithms to distinguish the overlapping pairs of photons from noise, as well as to precisely determine the mass of the particle from which they originated. A wide range of masses was explored. No evidence for such new particles was seen, enabling them to set upper limits on the production rate of this process. The result is the most sensitive search yet performed for such Higgs-like particles in this final state.
How can the scientists test the AI's effectiveness? It is not as easy as verifying AI facial differentiation, where you can simply check by looking. Thankfully, the SM has well-understood processes, which CMS physicists used to validate and control the AI techniques. For example, the η meson, which also decays to two photons, provided an ideal test bench. Scientists at CMS were able to cleanly identify and reconstruct the η meson when searching for its decay into photons when they applied these AI techniques.
This analysis clearly shows that AI algorithms can be used to cleanly identify two-photon signatures from the noise and to search for new massive particles. These machine learning techniques are continuously improving and will continue to be used in unique analyses of LHC data, extending CMS searches to even more challenging cases.