Forest fire season has officially begun in Canada. Across the country's north and west, well over 100 wildfires have been reported. Towns like Fort McMurray, devastated by fire in 2016, have been partially evacuated and air quality alerts are being issued in Canada and part of the United States.
The key to containing the damage caused by climate-change-driven fires is early detection: the sooner these fires are spotted, the quicker and easier they can be extinguished. Most early-detection systems use a cocktail of satellite imagery, ground sensors and helicopter-based observation. But researchers at Concordia's Gina Cody School of Engineering and Computer Science are developing an entirely automated system instead. It combines deep neural networks, image processing and unmanned aerial vehicles (UAVs or drones) to spot and track fires before they blaze out of control.
In a paper published in IEEE Transactions on Industrial Electronics, the authors propose their method that employs UAV-based visual-infrared camera aerial images in an integrated design.
"The drones act as a mobile sensor, which makes them more convenient," says Youmin Zhang, a professor in the Department of Mechanical, Industrial and Aerospace Engineering and the paper's corresponding author.
"They allow for faster action at a lower cost. With visual and infrared cameras and the embedded artificial intelligence (AI) algorithms, we can move toward autonomous operations of forest fire detection and control."
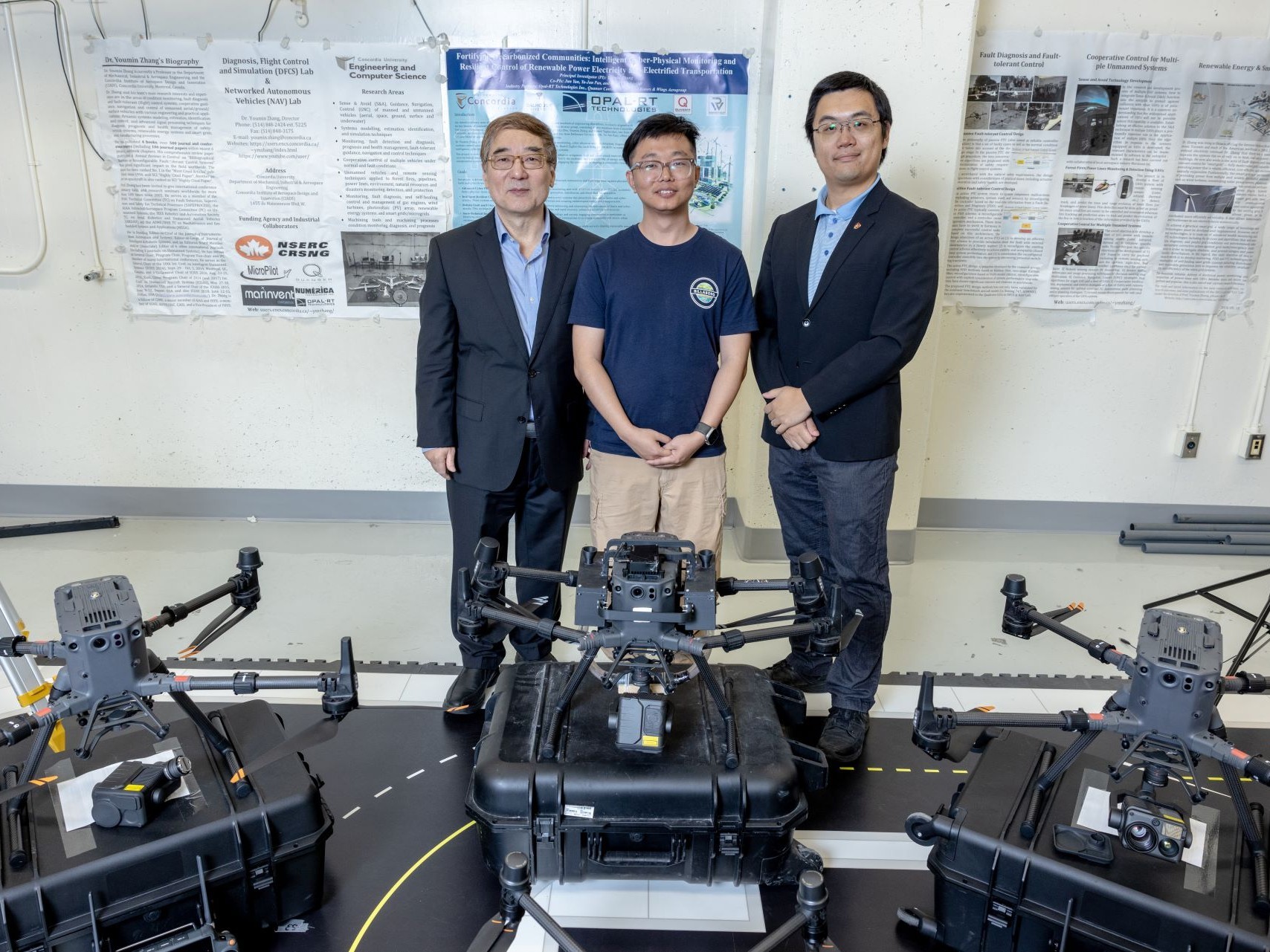
An affordable, autonomous solution
The system designed by the researchers is made up of three main modules.
The first uses a type of image-analysis neural network called an attention-gate U-Net, which focuses on specific and relevant parts of an image in a highly detailed and structured way. This helps identify smoke and suspected flame segmentation while decreasing the risks of false alarms.
The second module determines the location of the UAV and fires. The system relies on a monocular simultaneous localisation and mapping (SLAM) algorithm, a technique used in computer vision to track and map a camera's environment. This is applied alongside wildfire spot distance estimation algorithms, which predict the spread of the forest fire's embers using a sophisticated model based on variables such as wind, ember size, topography and heat, along with the UAV's own GPS system to geolocate the suspected wildfire spots.
Last, a transformation matrix is used to register visual and infrared images. This helps avoid false detection of forest fires and presents a complete picture without any key details or segments left out.
The researchers point out that the visual cameras used in their research are common and affordable, and they are capable of capturing images of rising smoke. But because forest fires usually start under the tree canopy and are undetectable by conventional visual cameras, the infrared camera can detect a new fire's radiation emissions and heat signals, thus enabling the system to accurately geoposition it before it grows into an inferno.
While field testing proved that their method worked on a small scale, Zhang and his co-authors say much more work needs to be done before their method can be employed by fire-fighting agencies. Capacity and computer resources are obstacles they must still overcome. But he is confident their approach is heading in a promising direction.
"In some senses, our research is leading in the investigation and verification of this technique, and in developing a theory toward a real application in the future."
PhD student Linhan Qiao and Shun Li, MSc 23, are co-first authors. Associate professor and Concordia University Research Chair (Tier 2) in Artificial Intelligence in Cyber Security and Resilience Jun Yan also contributed to this work as co-supervisor to Li.
The research was supported by the Natural Sciences and Engineering Research Council of Canada (NSERC) and by the Consortium for Research and Innovation in Aerospace in Québec project called AirTanker Visual Intelligent Tracking & Airborne Guidance System (AVITAGS).
Read the cited paper: "Early Wildfire Detection and Distance Estimation Using Aerial Visible-Infrared Images."