Abstract
Recognizing binding sites of DNA-binding proteins is a key factor for elucidating transcriptional regulation in organisms. ChIP-exo enables researchers to delineate genome-wide binding landscapes of DNA-binding proteins with near single base-pair resolution. However, the peak calling step hinders ChIP-exo application since the published algorithms tend to generate false-positive and false-negative predictions. Here, we report the development of DEOCSU (DEep-learning Optimized ChIP-exo peak calling SUite), a novel machine learning-based ChIP-exo peak calling suite. DEOCSU entails the deep convolutional neural network model which was trained with curated ChIP-exo peak data to distinguish the visualized data of bona fide peaks from false ones. Performance validation of the trained deep-learning model indicated its high accuracy, high precision and high recall of over 95%. Applying the new suite to both in-house and publicly available ChIP-exo datasets obtained from bacteria, eukaryotes and archaea revealed an accurate prediction of peaks containing canonical motifs, highlighting the versatility and efficiency of DEOCSU. Furthermore, DEOCSU can be executed on a cloud computing platform or the local environment. With visualization software included in the suite, adjustable options such as the threshold of peak probability, and iterable updating of the pre-trained model, DEOCSU can be optimized for users' specific needs.
Chromatin immunoprecipitation (ChIP) has been widely employed by researchers to determine the in vivo location of DNA binding sites on the genome for a particular protein of interest. In particular, ChIP-exo enables researchers to delineate genome-wide binding landscapes of DNA-binding proteins with near single base-pair resolution. However, the peak calling step hinders ChIP-exo application because the published algorithms tend to have higher false-positive and false-negative prediction rates.
To solve this, a research team, led by Professor Donghyuk Kim from the Department of Energy and Chemical Engineering at UNIST has introduced a novel machine learning-based ChIP-exo peak calling suite, also known as DEOCSU (DEep-learning Optimized ChIP-exo peak calling SUite).
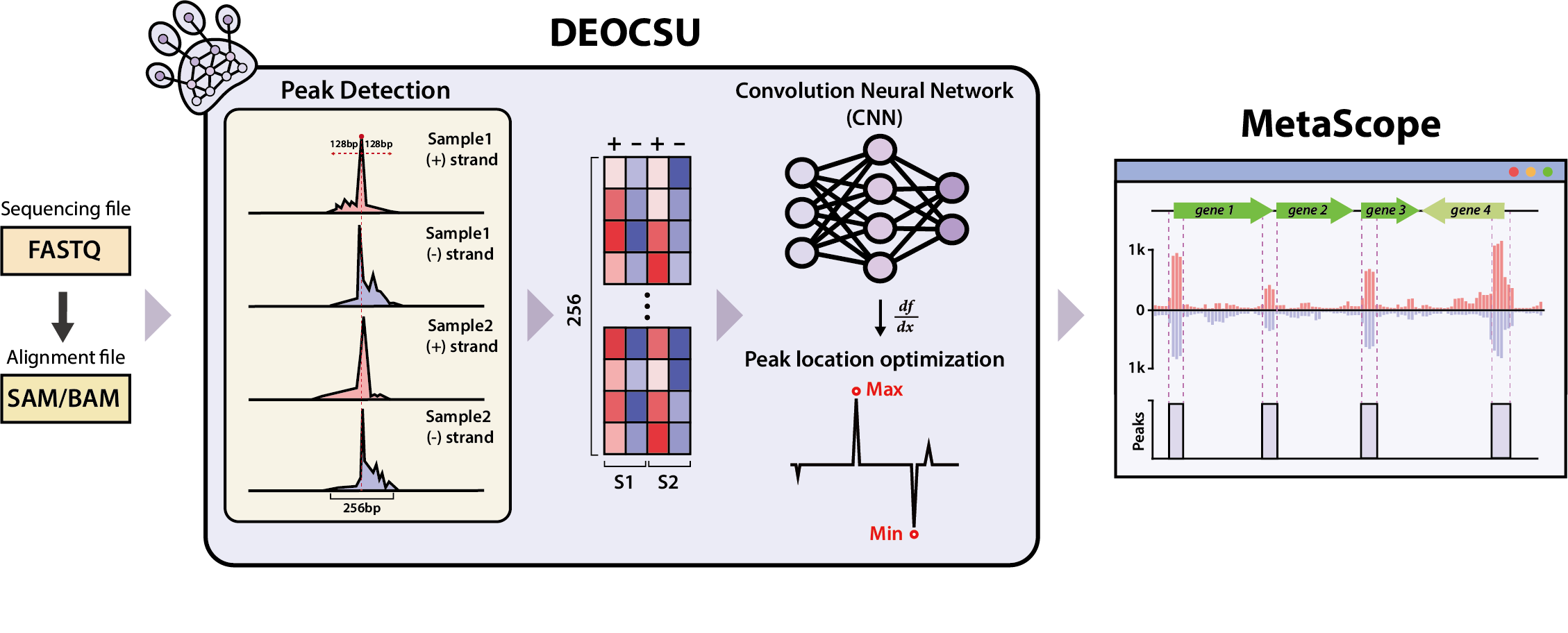
Figure 1. Overview of DEOCSU
/Public Release. This material from the originating organization/author(s) might be of the point-in-time nature, and edited for clarity, style and length. Mirage.News does not take institutional positions or sides, and all views, positions, and conclusions expressed herein are solely those of the author(s).View in full
here.