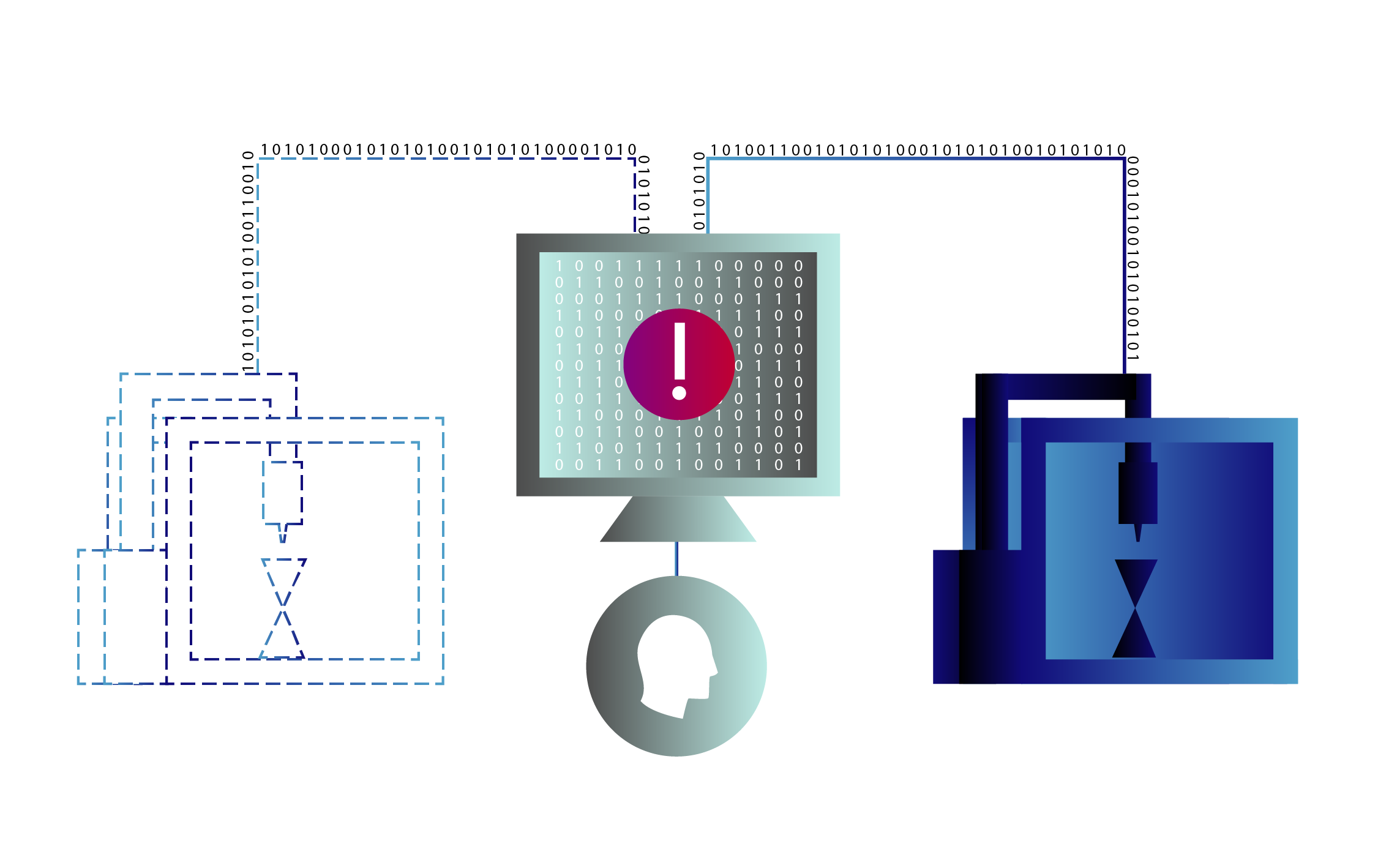
N. Hanacek/NIST
Detailed virtual copies of physical objects, called digital twins, are opening doors for better products across automotive, health care, aerospace and other industries. According to a new study, cybersecurity may also fit neatly into the digital twin portfolio.
As more robots and other manufacturing equipment become remotely accessible, new entry points for malicious cyberattacks are created. To keep pace with the growing cyber threat, a team of researchers at the National Institute of Standards and Technology (NIST) and the University of Michigan devised a cybersecurity framework that brings digital twin technology together with machine learning and human expertise to flag indicators of cyberattacks.
In a paper published in IEEE Transactions on Automation Science and Engineering, the NIST and University of Michigan researchers demonstrated the feasibility of their strategy by detecting cyberattacks aimed at a 3D printer in their lab. They also note that the framework could be applied to a broad range of manufacturing technologies.
Cyberattacks can be incredibly subtle and thus difficult to detect or differentiate from other, sometimes more routine, system anomalies. Operational data describing what is occurring within machines - sensor data, error signals, digital commands being issued or executed, for instance - could support cyberattack detection. However, directly accessing this kind of data in near real time from operational technology (OT) devices, such as a 3D printer, could put the performance and safety of the process on the factory floor at risk.
"Typically, I have observed that manufacturing cybersecurity strategies rely on copies of network traffic that do not always help us see what is occurring inside a piece of machinery or process," said NIST mechanical engineer Michael Pease, a co-author of the study. "As a result, some OT cybersecurity strategies seem analogous to observing the operations from the outside through a window; however, adversaries might have found a way onto the floor."
Without looking under the hood of the hardware, cybersecurity professionals may be leaving room for malicious actors to operate undetected.
Taking a Look in the Digital Mirror
Digital twins aren't your run-of-the-mill computer models. They are closely tied to their physical counterparts, from which they extract data and run alongside in near real time. So, when it's not possible to inspect a physical machine while it's in operation, its digital twin is the next best thing.
In recent years, digital twins of manufacturing machinery have armed engineers with an abundance of operational data, helping them accomplish a variety of feats (without impacting performance or safety), including predicting when parts will start to break down and require maintenance.
In addition to spotting routine indicators of wear and tear, digital twins could help find something more within manufacturing data, the authors of the study say.
"Because manufacturing processes produce such rich data sets - temperature, voltage, current - and they are so repetitive, there are opportunities to detect anomalies that stick out, including cyberattacks," said Dawn Tilbury, a professor of mechanical engineering at the University of Michigan and study co-author.
To seize the opportunity presented by digital twins for tighter cybersecurity, the researchers developed a framework entailing a new strategy, which they tested out on an off-the-shelf 3D printer.
The team built a digital twin to emulate the 3D printing process and provided it with information from the real printer. As the printer built a part (a plastic hourglass in this case), computer programs monitored and analyzed continuous data streams including both measured temperatures from the physical printing head and the simulated temperatures being computed in real time by the digital twin.
The researchers launched waves of disturbances at the printer. Some were innocent anomalies, such as an external fan causing the printer to cool, but others, some of which caused the printer to incorrectly report its temperature readings, represented something more nefarious.
So, even with the wealth of information at hand, how did the team's computer programs distinguish a cyberattack from something more routine? The framework's answer is to use a process of elimination.
The programs analyzing both the real and digital printers were pattern-recognizing machine learning models trained on normal operating data, which is included in the paper, in bulk. In other words, the models were adept at recognizing what the printer looked like under normal conditions, also meaning they could tell when things were out of the ordinary.
If these models detected an irregularity, they passed the baton off to other computer models that checked whether the strange signals were consistent with anything in a library of known issues, such as the printer's fan cooling its printing head more than expected. Then the system categorized the irregularity as an expected anomaly or a potential cyber threat.
In the last step, a human expert is meant to interpret the system's finding and then make a decision.
"The framework provides tools to systematically formalize the subject matter expert's knowledge on anomaly detection. If the framework hasn't seen a certain anomaly before, a subject matter expert can analyze the collected data to provide further insights to be integrated into and improve the system," said lead-author Efe Balta, a former mechanical engineering graduate student at the University of Michigan and now a postdoctoral researcher at ETH Zurich.
Generally speaking, the expert would either confirm the cybersecurity system's suspicions or teach it a new anomaly to store in the database. And then as time goes on, the models in the system would theoretically learn more and more, and the human expert would need to teach them less and less.
In the case of the 3D printer, the team checked its cybersecurity system's work and found it was able to correctly sort the cyberattacks from normal anomalies by analyzing physical and emulated data.
But despite the promising showing, the researchers plan to study how the framework responds to more varied and aggressive attacks in the future, ensuring the strategy is reliable and scalable. Their next steps will likely also include applying the strategy to a fleet of printers at once, to see if the expanded coverage either hurts or helps their detection capabilities.
"With further research, this framework could potentially be a huge win-win for both maintenance as well as monitoring for indications of compromised OT systems," Pease said.
Paper: E. C. Balta, M. Pease, J. Moyne, K. Barton and D. M. Tilbury. Cyber-Attack Detection Digital Twins for Cyber-Physical Manufacturing Systems. IEEE Transactions on Automation Science and Engineering. Published online Feb. 22, 2023. DOI: 10.1109/TASE.2023.3243147