In the agricultural and food industry, determining the chemical composition of raw materials is important for production efficiency, application, and price. Traditional laboratory testing is time-consuming, complicated, and expensive. New research from the University of Illinois Urbana-Champaign demonstrates that near-infrared (NIR) spectroscopy and machine learning can provide quick, accurate, and cost-effective product analysis.
- Marianne Stein
In two studies, the researchers explore the use of NIR spectroscopy for analyzing characteristics of corn kernels and sorghum biomass.
"NIR spectroscopy has many advantages over traditional methods. It is fast, accurate, and inexpensive. Unlike lab analysis, it does not require the use of chemicals, so it's more environmentally sustainable. It does not destroy the samples, and you can analyze multiple features at the same time. Once the system is set up, anyone can run it with minimal training," said Mohammed Kamruzzaman, assistant professor in the Department of Agricultural and Biological Engineering (ABE), part of the College of Agricultural, Consumer and Environmental Sciences and The Grainger College of Engineering at U. of I. He is a co-author on both papers.
In the first study, the researchers created a global model for corn kernel analysis. Moisture and protein content impact nutritional value, processing efficiency, and price of corn, so the information is crucial for the grain processing industry.
NIR and other spectroscopic techniques are indirect methods. They measure how a material absorbs or emits light at different wavelengths, then construct a unique spectrum that is translated into product characteristics with machine learning models. Many food and agricultural processing facilities already have NIR equipment, but models need to be trained for specific purposes.
"Corn grown in different locations varies because of soil, environment, management, and other factors. If you train the model with corn from one location, it will not be accurate elsewhere," Kamruzzaman said.
To address this issue and develop a model that applies in many different locations, the researchers collected corn samples from seven countries - Argentina, Brazil, India, Indonesia, Serbia, Tunisia, and the USA.
"To analyze moisture and protein in the corn kernels, we combined gradient-boosting machines with partial least squares regression. This is a novel approach that yields accurate, reliable results," said Runyu Zheng, a doctoral student in ABE and lead author on the first study.
While the model is not 100% global, it provides considerable variability in the data and will work in many locations. If needed, it can be updated with additional samples from new locations, Kamruzzaman noted.
In the second study, the researchers focused on sorghum biomass, which can serve as a renewable, cost-effective, and high-yield feedstock for biofuel.
Biomass conversion into biofuels depends on chemical composition, so a rapid and efficient method of sorghum biomass characterization could assist biofuel, breeding, and other relevant industries, the researchers explained.
Using sorghum from the University of Illinois Energy Farm, they were able to accurately and reliably predict moisture, ash, lignin, and other features.
"We first scanned the samples and obtained NIR spectra as an output. This is like a fingerprint that is unique to different chemical compositions and structural properties. Then we used chemometrics - a mathematical-statistical approach - to develop the prediction models and applications," said Md Wadud Ahmed, a doctoral student in ABE and lead author on the second paper.
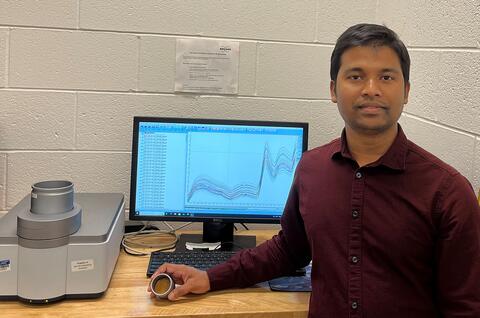
While NIR spectroscopy is not as accurate as lab analysis, it is more than sufficient for practical purposes and can provide fast, efficient screening methods for industrial use, Kamruzzaman said.
"A major advantage of this technology is that you don't need to remove and destroy products. You can simply take samples for measurement, scan them, and then return them to the production stream. In some cases, you can even scan the samples directly in the production line. NIR spectroscopy provides a lot of flexibility for industrial usage," he concluded.
The first paper, "Optimizing feature selection with gradient boosting machines in PLS regression for predicting moisture and protein in multi-country corn kernels via NIR spectroscopy," is published in Food Chemistry [DOI: 10.1016/j.foodchem.2024.140062].
The second paper, "Rapid and high-throughput determination of sorghum (Sorghum bicolor) biomass composition using near infrared spectroscopy and chemometrics," is published in Biomass and Bioenergy [DOI:10.1016/j.biombioe.2024.107276].
This work was funded by the DOE Center for Advanced Bioenergy and Bioproducts Innovation (U.S. Department of Energy, Office of Science, Biological and Environmental Research Program under Award Number DE-SC0018420).