The models aim to remove 'hallucinations' from AI-generated language.
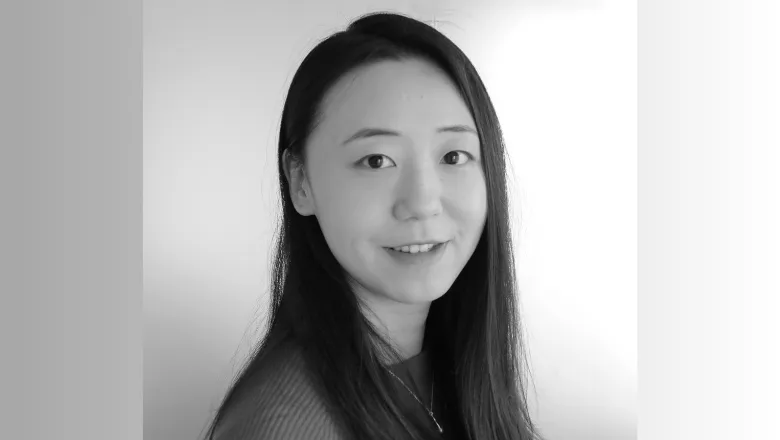
Dr Zheng Yuan has signed a multi-year gift agreement with NetMind AI to develop systems to filter out non-factual or irrelevant information in natural language generation systems.
The Department of Informatics researcher aims to develop the next generation of algorithms that integrate robust fact-checking protocols and effective hallucination mitigation strategies. An artificial intelligence hallucination is when a response generated by AI contains false or misleading information presented as fact.
This work will support natural language generation systems, such as chatbots, to produce text that appears both natural and remains verifiably true.
The research will be funded by a gift agreement with Net Mind AI - a start-up Dr Yuan has been working with since 2022. Based in London, Net Mind AI aims at making AI more accessible and affordable by creating distributed computing platforms and AI ecosystems.
They company also supports the growing AI research community to address the most critical challenges and opportunities in AI. The gift funding will support Dr Yuan and her group of researchers, including PhD students.
Dr Zheng Yuan, "The public has access to amazing tools with generative AI, and they can use these tools to perform various tasks including generating text. People use AI for everything from checking grammar to polishing academic writing and business emails. This is the power of natural language processing - however we notice they tend to 'hallucinate'".
"The world is evolving and there's new information coming out every day from different sources. We're trying to build an external knowledge base that can detect whether that information is both factual and relevant to the user."
Natural language generation hallucinations tend to 'slip through the cracks' because all traditional evaluation systems were built or proposed before the systems existed.
The first step towards targeting this issue is to identify better evaluation systems that can systematically evaluate which natural language generation model is better at preserving the facts. From there, the group will use the evaluation system to feed their model training.
"We're trying to build a better system where end users have more control and generative AI can better meet their requirements."
Dr Zheng Yuan
"The evaluation system will detect if a hallucination is factual or non-factual. Factual hallucinations occur when information is true but irrelevant or unexpected. Once the type of hallucination is identified, we can propose different mitigation strategies depending on the level of hallucination," Dr Yuan said.
The new evaluation system would filter out non-factual hallucinations entirely or propose corrections; while factual hallucinations would be identified as true but allow the user to keep the information or filter it out.
"We're trying to build a better system where end users have more control and generative AI can better meet their requirements," Dr Yuan said.
Applications include better language translation - where the meaning in the original language is retained rather than a direct translation of the words; and more accurate summarisation abilities, which stick closely to the source information.