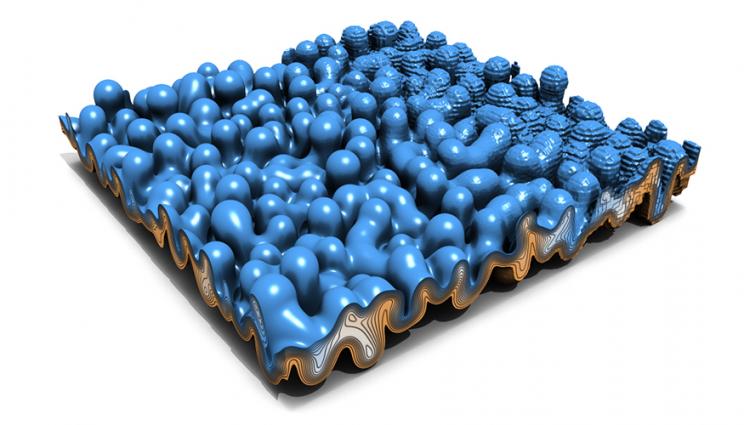
This illustration, showing the density field from a Rayleigh-Taylor instability simulation, depicts how lossy compression affects data quality. Using the zfp open source software, researchers can vary the compression ratio to any desired setting, from 10:1 (shown on the left) to 250:1 (on the right), where compression errors become apparent. Image courtesy of Peter Lindstrom.
A Lawrence Livermore National Laboratory-led effort in data compression was one of nine projects recently funded by the U.S. Department of Energy (DOE) for research aimed at shrinking the amount of data needed to advance scientific discovery.
LLNL was among five DOE national laboratories to receive awards totaling $13.7 million for data reduction in scientific applications, where massive datasets produced by high-fidelity simulations and upgraded supercomputers is beginning to exceed scientists' abilities to effectively collect, store and analyze it.
Under the project - ComPRESS: Compression and Progressive Retrieval for Exascale Simulations and Sensors - LLNL scientists will seek better understanding of data-compression errors, develop models to increase trust in data compression for science and design a new hardware and software framework to drastically improve performance of compressed data. The team anticipates the improvements will enable higher-fidelity science runs and experiments, particularly on emerging exascale supercomputers capable of at least one quintillion operations per second.
Led by principal investigator Peter Lindstrom and co-investigators at LLNL, Alyson Fox and Maya Gokhale, the ComPRESS team will develop capabilities allowing for sufficient data extraction while storing only compressed representations of data. They plan to develop error models and other techniques for accurate error distributions and new hardware compression designs to improve input/output performance. The work will build on zfp, a Lab-developed, open-source, versatile high-speed data compressor capable of dramatically reducing the amount of data for storage or transfer.
"Scientific progress increasingly relies on acquiring, analyzing, transferring and archiving huge amounts of numerical data generated in computer simulations, observations and experiments," Lindstrom said. "Our project aims to tackle the challenges associated with this data deluge by developing next-generation data compression algorithms that reduce or even discard unimportant information in a controlled manner without adverse impact on scientific conclusions."
In addition to greatly reducing storage and transfer costs, data compression should allow important scientific questions to be answered through the acquisition of higher-resolution data, which, without compression, would vastly exceed current storage and bandwidth capacities, Lindstrom added.
The team anticipates the framework will reduce the amount of data required by 2-3 orders of magnitude and plan to demonstrate the techniques on scientific applications including climate science, seismology, turbulence and fusion.
Managed by the Advanced Scientific Computing Research program within the DOE Office of Science, the newly funded data-reduction projects were evaluated for efficiency, effectiveness and trustworthiness, according to DOE. They cover a wide range of promising data-reduction topics and techniques, including advanced machine learning, large-scale statistical calculations and novel hardware accelerators.