A researcher at EPFL working at the crossroads of neuroscience and computational science has developed an algorithm that can predict how surprise and novelty affect behavior.
Surprising and unexpected events - a villain in a film who suddenly makes a compassionate gesture, a presidential candidate who wins even though his friends thought he'd lose, or a long-lost friend you bump into on vacation in a distant country - trigger natural responses in our bodies. These responses are a combination of thoughts and behaviors, and could, in these examples, mean gaining a better appreciation of the film director, rethinking our social circle or rekindling an old friendship.
Such responses are what scientists at EPFL's Laboratory of Computational Neuroscience, headed by Wulfram Gerstner, are studying. Although much research has been done in neuroscience and psychology on how the brain responds to surprise and novelty and the attendant effects on behavior, many questions remain. For instance, it's not clear exactly what is meant by "surprise" and "novelty" and how these situations impact certain brain functions.
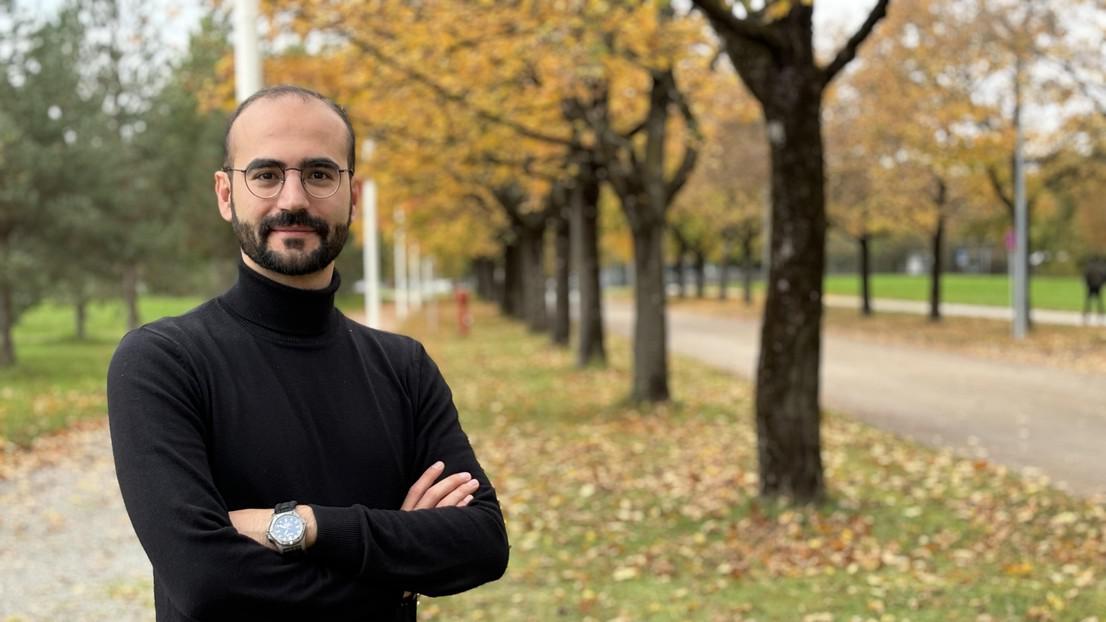
Alireza Modirshanechi, currently a postdoc at Helmholtz Munich in Germany, conducted his PhD thesis research at Gerstner's lab, examining these issues with the help of artificial intelligence (AI). He compiled various definitions of surprise to develop an algorithm that can predict how humans will respond to surprising and novel situations. The algorithm essentially describes an intelligent agent that mimics a human being and that can perform various tasks (in experiments run by the researchers) with the same results as those obtained by human participants. "By understanding the algorithm, we can gain insight into how human brain and cognition work," says Modirshanechi. This paves the way to a better understanding of learning, memorization and decision-making processes. His PhD research earned him the EPFL Dimitris N. Chorafas Foundation Award for 2024.
Our goal isn't to create an AI system that works better than humans, but rather to design one that's more faithful to human behavior in order to improve our understanding of the brain
18 mathematical definitions of surprise and novelty
The first challenge Modirshanechi faced was finding a way to define "surprise" for an intelligent agent. The underlying idea is that since the agent is designed to mimic humans, it should be surprised in situations when humans are surprised. Drawing on classic experiments in behavioral studies, Modirshanechi developed a taxonomy of 18 mathematical definitions of surprise and novelty that he programmed into the intelligent agent. In some of these experiments, human participants were asked to read sentences containing words that had been classified as either expected or unexpected; in others, participants listened to a series of repetitive sounds that were suddenly interrupted by a strange noise. And in yet others, the logical predictions made by participants were confounded - they expected X to occur, but Y did instead. Modirshanechi's taxonomy of mathematical definitions serves to evaluate how an intelligent agent feels "surprised" in these kinds of situations - information that can eventually help scientists better understand how humans react to surprising and novel events.
Modirshanechi used mathematical methods to investigate the similarities and differences between the 18 definitions and the conditions that make them indistinguishable. He then outlined a unified framework for the systematic comparison of different approaches to quantifying surprise and how this emotion influences the brain. His algorithm distinguishes between "surprise" as a modulator of learning speed and "novelty" as a driver of goal-directed exploration.
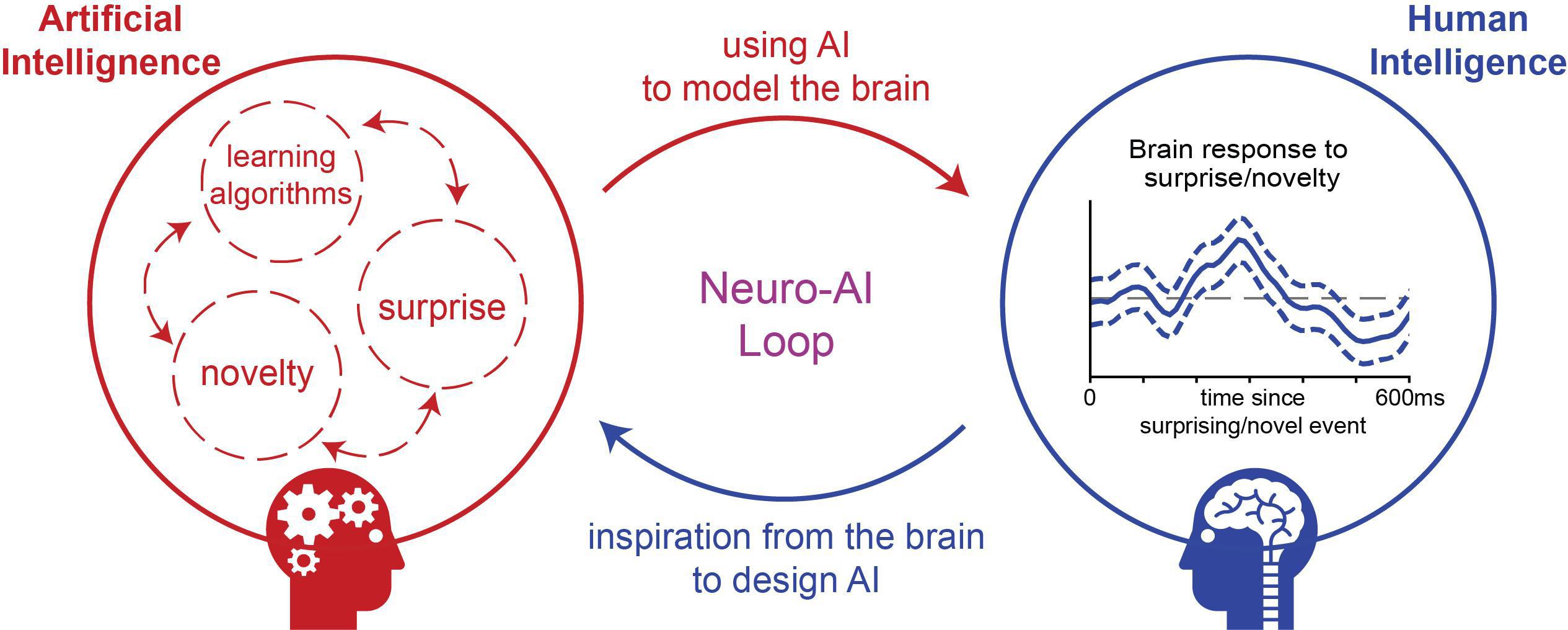
Test the algorithm's predictions on humans
"New things can be surprising, but they can also be expected," says Modirshanechi. "For example, seeing a solar eclipse for the first time is a new experience but it's also an expected one, as you had heard about the eclipse in the news. On the other hand, if your mother who lives in another country suddenly knocks on your door, her visit wouldn't be a new experience but it would be a surprising one. In our research, we quantified this mathematically and drew a distinction between surprise, which accelerates the learning process, and novelty, which drives exploration. We dissociated these signals in the brain."
The next step was to test the algorithm's predictions against the actual behavior of humans. For this, Modirshanechi worked with EPFL's Laboratory of Psychophysics, headed by Michael Herzog, to analyze behavioral and electroencephalography (EEG) data collected during cognitive experiments with human participants. He found that both surprise and novelty were crucial determinants of human behavior in volatile environments with sparse rewards. "The algorithm successfully predicted 60% to 80% of the participants' decisions," says Modirshanechi.
"Our goal isn't to create an AI system that works better than humans, but rather to design one that's more faithful to human behavior in order to improve our understanding of the brain," he explains. "Everyone knows that if you drop an apple, it'll fall to the ground. But Newton found a mathematical formula to describe this process. That's similar to what we're trying to do - we've developed an algorithm that can predict when and by how much humans will be surprised, and we can explain the equation by which humans learn faster when they're surprised."
What's more, Modirshanechi's algorithm provides a basis for further research. "For instance, EEG data suggest that people with schizophrenia perceive surprise differently from people in control groups," he says. "But we don't know to what extent their perception is different. Our mathematical formula can quantify that. It can be used to answer other questions, too: Do schizophrenic patients learn more slowly because they don't feel surprised? Do they seek out information less effectively because they don't experience novelty? With our algorithm, scientists can design experiments to test these kinds of hypotheses."
Applications in other fields
The algorithm has applications in other fields as well. In education, for example, teachers can use it to explore how surprise can reinforce the learning and memorization process.
Modirshanechi's research similarly makes an important contribution to the field of AI. "Most algorithms that exist today are based on stable environments," he says. "But in the real world, things change constantly. Engineers need to be able to incorporate the 'surprise' factor into their models to make them more reliable and secure. I think the time is ripe to address that issue and develop the next generation of AI programs."