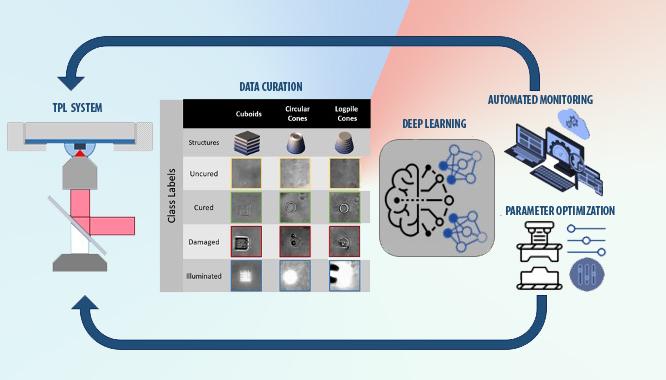
Lawrence Livermore National Laboratory scientists and collaborators are using machine learning to address two key barriers to industrialization of two-photon lithography (TPL): monitoring of part quality during printing and determining the right light dosage for a given material. The team developed a machine learning algorithm trained on thousands of video images of TPL builds to identify the optimal parameters for settings such as exposure and laser intensity and to automatically detect part quality at high accuracy.
Two-photon lithography (TPL) - a widely used 3D nanoprinting technique that uses laser light to create 3D objects - has shown promise in research applications but has yet to achieve widespread industry acceptance due to limitations on large-scale part production and time-intensive setup.
Capable of printing nanoscale features at a very high resolution, TPL uses a laser beam to build parts, focusing an intense beam of light on a precise spot within a liquid photopolymer material. The volumetric pixels, or "voxels," harden the liquid to a solid at each point the beam hits and the uncured liquid is removed, leaving behind a 3D structure. Building a high-quality part with the technique requires walking a fine line: too little light and a part can't form, too much and it causes damage. For operators and engineers, determining the correct light dosage can be a laborious manual process.
Lawrence Livermore National Laboratory (LLNL) scientists and collaborators turned to machine learning to address two key barriers to industrialization of TPL: monitoring of part quality during printing and determining the right light dosage for a given material. The team's machine learning algorithm was trained on thousands of video images of builds labeled as "uncured," "cured" and "damaged," to identify the optimal parameters for settings such as exposure and laser intensity and to automatically detect part quality at high accuracy. The work was recently published in the journal Additive Manufacturing.
"You never know the exact parameters for a given material, so you typically go through this terrible process of loading up the device, printing hundreds of objects and manually sorting through the data," said principal investigator and LLNL engineer Brian Giera. "What we did was run the routine set of experiments and made an algorithm that automatically processes the video to quickly identify what's good and what's bad. And what you get for free out of that process is an algorithm that also operates on real-time quality detection."
The team developed the algorithm and trained it on experimental data collected by Sourabh Saha, a former LLNL research engineer who is now an assistant professor at Georgia Institute of Technology. Saha designed the experiments to clearly show how changes in light dosage affected the transitions among the uncured, cured and damaged builds, and printed a range of objects with two types of photo-curing polymer using a commercially available TPL printer.
"The popularity of TPL lies in its ability to build a variety of arbitrarily complex 3D structures," Saha said. "However, this presents a challenge for traditional automated process monitoring techniques because the cured structures can look radically different from each other - human experts can intuitively identify the transitions. Our goal here was to show that machines can be taught this skill."
The researchers collected more than 1,000 videos of various kinds of parts built under different light dosage conditions. Xian Lee, a graduate student at Iowa State University, manually sifted through each frame of the videos, examining tens of thousands of images to analyze each transition region.
Using the deep learning algorithm, researchers found they could detect part quality at greater than 95 percent accuracy within a few milliseconds, creating an unprecedented monitoring capability for the TPL process. Giera said operators could apply the algorithm to an initial set of experiments and create a pretrained model to accelerate parameter optimization and provide them with a way to oversee the build process and anticipate problems such as unexpected over-curing in the device.
"What this allows for is actual qualitative process monitoring where there wasn't a capability to do that before," Giera said, "Another neat feature is it basically only uses image data. If I had a very large area and I'm building at multiple build locations to then assemble a master part, I could actually record video of all those areas, feed those sub-images into an algorithm and have parallel monitoring."
In the spirit of transparency, the team also described instances where the algorithm made mistakes in predictions, showing an opportunity for improving the model to better recognize dust particles and other particulate matter that could affect build quality. The team released the entire dataset to the public, including the model, training weights and actual data for further innovation by the scientific community.
"Because machine learning is such an evolutionary field, if we put the data out there then this problem can benefit from other people solving it. We've done this starter dataset for the field, and now everyone can move forward," Giera said. "This allows us to benefit from the broader machine learning community, which may not know as much about additive manufacturing as we do but do know more about new techniques they're developing."
The work stemmed from a previous Laboratory Directed Research and Development (LDRD) project on two-photon lithography and was finished under a current LDRD titled "Accelerated Multi-Modal Manufacturing Optimization (AMMO)."
Co-author Soumik Sarkar of Iowa State University also contributed to the work.