Quantum chemistry, the study of chemical properties and processes at the quantum scale, has opened many paths to research and discovery in modern chemistry. Without ever handling a beaker or a test tube, chemists can make predictions about the properties of a given atom or molecule and how it will undergo chemical reactions by studying its electronic structure-how its electrons are arranged in orbitals-and how those electrons interact with those of other compounds or atoms.
However, as powerful as quantum chemistry has shown itself to be, it also has a big drawback: Accurate calculations are resource-intensive and time consuming, with routine chemical studies involving computations that take days or longer.
Now, thanks to a new quantum chemistry tool that uses machine learning, quantum-chemistry calculations can be performed 1,000 times faster than previously possible, allowing accurate quantum chemistry research to be performed faster than ever before.
The tool, called OrbNet, was developed through a partnership between Caltech's Tom Miller, professor of chemistry, and Anima Anandkumar, Bren Professor of Computing and Mathematical Sciences.
"In quantum chemistry, there has been a punishing interplay between the accuracy of the calculation and the amount of time it takes," Miller says. "You would start a calculation, and would say, 'Well, see you on Tuesday.' But now, the calculations can be done interactively."
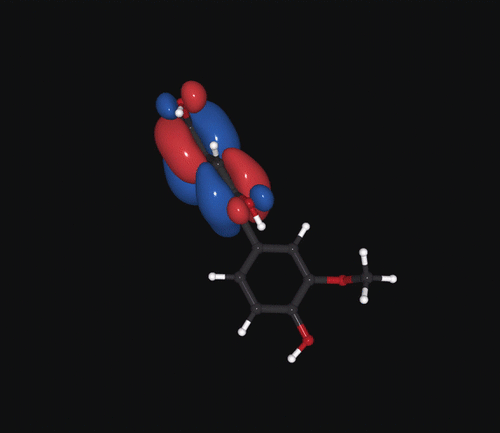
OrbNet processes quantum-chemistry simulations much more quickly than previously possible, allowing real-time manipulation of molecules in the program.
Credit: Caltech
OrbNet uses a graph neural network, a type of machine-learning system that represents information as "nodes," which contain data, and "edges," which represent the ways those chunks of data are related to one another.
Miller says OrbNet works as well as it does because of an innovation in the way atoms and molecules are mapped to the graph neural network that is based around the Schrödinger equation, a piece of mathematics central to quantum mechanics.
"Previous graph architectures had organized the atoms as nodes and the bonds between the atoms as edges, but that's not the way quantum chemistry thinks about it," he says. "So, instead, we built a graph where the nodes are the electron orbitals, and the edges are interactions between the orbitals. That has a much more natural connection to the Schrödinger equation."
Like all machine-learning systems, OrbNet needs to be trained to perform an assigned task, similar to how a person who gets a new job needs to be trained for it. OrbNet learned to predict molecular properties on the basis of accurate reference quantum mechanical calculations. Anandkumar, whose research focuses on machine learning, helped design and optimize the graph neural network implementation.
"Orbnet is a great example of a deep-learning model that uses domain-specific features: in this case, molecular orbitals. This enables the machine-learning model to accurately do the calculations on molecules much larger, as much as 10 times larger, than the molecules present in training data," Anandkumar says. "For a standard deep-learning model, such extrapolation is impossible since it only learns to interpolate on the training data. Leveraging the domain knowledge of molecular orbitals was essential for us to achieve transferability to larger molecules.
Currently, OrbNet has been trained on approximately 100,000 molecules, which Miller says allows it to perform many useful calculations for researchers, but ongoing efforts aim to scale it to larger training data sets.
"The prediction of molecular properties has many practical benefits. For example, OrbNet can be used to predict the structure of molecules, the way in which they will react, whether they are soluble in water, or how they will bind to a protein," he says.
Miller says future work on OrbNet will focus on expanding the range of tasks it can be used for through additional training.
"We have demonstrated it works for a small corner of organic chemistry, but there's nothing that prevents us from expanding the approach to other application domains," he says.
The paper describing OrbNet, titled, "OrbNet: Deep Learning for Quantum Chemistry Using Symmetry-Adapted Atomic-Orbital Features," was published in the Journal of Chemical Physics on September 25 and selected as an Editor's Pick. Additional co-authors are Caltech chemistry graduate student Zhuoran Qiao, and Matthew Welborn and Frederick R. Manby of Entos, Inc.
The research was supported in part by Entos, Inc., a company spun off from Caltech in which Miller holds a financial stake. It was also supported by the Caltech De Logi fund.