
The coronavirus has the world in its grip. Finding a cure has never been more important. Unfortunately, the development of new drugs for treatment of the COVID-19 disease caused by the virus and development of a vaccine are complex, lengthy, and above all costly processes. With the help of computer models, this can be accelerated. Researchers at the Center for Computational Life Sciences (CCLS) are researching these models in order to reduce costs and speed up the process of drug development.
Traditional research is expensive
'On average, the development of a drug takes more than ten years and costs more than a billion dollars,' says Gerard van Westen, one of the researchers associated with CCLS. He works on machine learning applied to medicinal chemistry to explore the massive chemical space of potential new medicines. 'A drug is a molecule that has to meet many requirements. On the one hand, it must adhere to the target in the body, and on the other, it must not cause any side effects. It must also have the right characteristics so that it is soluble; is absorbed into the body, and ends up in the right place in the body. In my research we have to balance all these, often conflicting, objectives simultaneously.'
As a result, many drug candidates do not make it past the finish line. This often happens in the final phase in which the drugs are clinically tested by volunteers and patients. With the help of machine learning, or more specifically deep learning, new potential candidate medicines are generated. Van Westen subsequently uses machine learning methods to select the most promising potential medicines. Those that will likely not make it to the finish line can be written off at an early stage. After selection of the molecules they are made in the chemistry lab and tested in biochemical assays, to observe if the molecules behave as the computer predicted. 'The involvement of computers makes the entire process more efficient and can cut costs,' explains Van Westen.
The key that fits the lock
'The molecule must fit the receptor in the cell membrane. So we're actually looking in a pile of keys for the one key that fits the lock,' says Michael Emmerich, who is also connected to CCLS. 'In data we search for this using simulation, or 3-dimensional fitting. The advantage of this is that from the data only molecules that can actually bind to the receptor are identified as possible candidates. 'However, this is inefficient, because each molecule has to be examined separately,' explains Emmerich. 'The solution is machine learning combined with multi-criteria optimization algorithms. Multi-criteria optimisation algorithms make it possible to compare different alternatives or scenarios on the basis of many, often conflicting criteria. 'Based on earlier data we can predict how a molecule will behave and whether it will eventually be able to bind to the receptor. Within the Leiden Institute of Advanced Computer Science (LIACS) we are conducting extensive research into these machine learning models and couple them with multicriteria optimization methods that can search the huge space of all possible molecules for optimal candidate molecules with respect to conflicting criteria.'
Detect side effects in living cells
Once a potential drug has been found, High Throughput Screening (HTS) can provide a solution to identify possible side effects. HTS can rapidly identify active compounds, antibodies or genes, which provide leads for drug design and an understanding of the interaction or role of identified biochemical processes. Lu Cao, university lecturer at LIACS and also affiliated with CCLS, is an expert in this field. 'Computer models can filter candidate drugs based on predictions. But predicting how a drug reacts in a living organism is more difficult. High Throughput Screening can provide insight into this by working with living cells. As a result, any side effects of a potential drug can be discovered before clinical testing, leading to earlier discovery of a potential toxic drug.'
Coronavirus
In the context of the coronavirus, CCLS researchers are trying to support where they can. For example, with Ph.D. Student Patrick Echtenbruck and Professor Boris Naujoks from TH Cologne, Emmerich is looking for promising antiviral molecules that have already been approved as drug compounds. This process is known as drug repurposing. One idea is to bind receptors of lung cells, such as the ACE2 receptor. This receptor is where the SARS-CoV2 virus enters the cell and starts replication. Emmerich developed with his team and people of LACDR a machine learning program to determine whether a molecule is suitable or not with respect to multiple criteria. In a previous study in collaboration with Van Westen and LACDR this method proved to be very successful for other types of drugs.
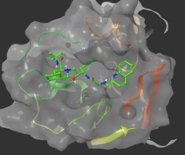
Van Westen has launched a three-dimensional virtual screening campaign ('docking'), looking for small molecules active against SARS-CoV-2 infection. In a similar repurposing approach these molecules include the screening of all approved drugs for affinity towards known viral targets such as the viral protease. They have found some potentially active molecules that warrant follow up screening and biological validation.
In the short term, Emmerich is cautious about expecting to contribute much to drug- and vaccine development for Covid-19 because pharmaceutical companies who are specialized in anti-viral drug- and vaccine development are already doing the same research in cell lines or even in clinical settings. 'Our research can yield improvements in the mid-term and long-term,' says Emmerich. 'Once the first drugs are available, the search for alternative drugs will continue, that is, drugs with less side effects, less costly, or applicable to a broader range of patients. This is where our research into the multi-criteria approach to drug development will be beneficial.'