AI systems already work their magic in many areas of biomedical science, helping to solve protein structure, discover hidden patterns in the genome and process massive amounts of biological data. Now, an AI-assisted technology developed at the Weizmann Institute of Science and described in Nature Biotechnology may grant researchers and physicians an unprecedented means of peering deep into the body's tissues by making it possible to simultaneously view more proteins than ever before, in a tissue sample.
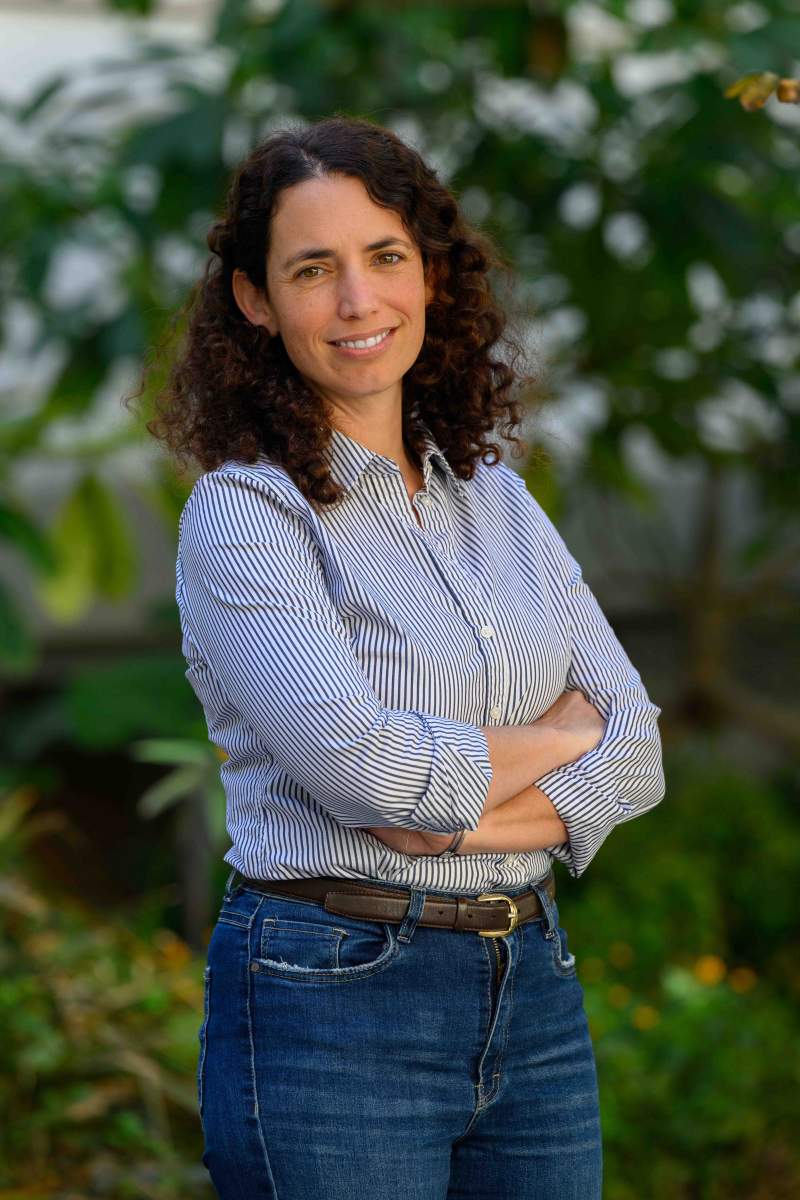
"To understand how any particular tissue works, it's crucial to measure lots of its proteins at the same time," says Dr. Leeat Keren of Weizmann's Molecular Cell Biology Department, who headed the research team. "This gives us an idea of which cells are present in the tissue and how they communicate and interact with one another."
Keren explains that this knowledge is vital to the study of disease processes. Cancerous growths, for example, contain, in addition to tumor cells, various other cell types, including healthy cells of the tissue the tumor is growing on and of the immune system. The cellular makeup of the tumor and how those cell types interact with one another can determine the effectiveness of therapies or be used to predict which patients have a better prognosis and which are likely to develop metastases. Such findings, in turn, can lead to improved personalized treatments.
This new Weizmann Institute technology, called CombPlex, offers a significant leap forward in the study of tissues' cellular composition. Unlike conventional methods that capture data from just three or four proteins at a time, CombPlex, in its proof-of-concept stage, has already demonstrated the ability to simultaneously image and quantify nearly two dozen proteins within individual cells, with the potential to scale up this ability to hundreds of proteins in the future. The technology requires no additional instruments, making it highly accessible.
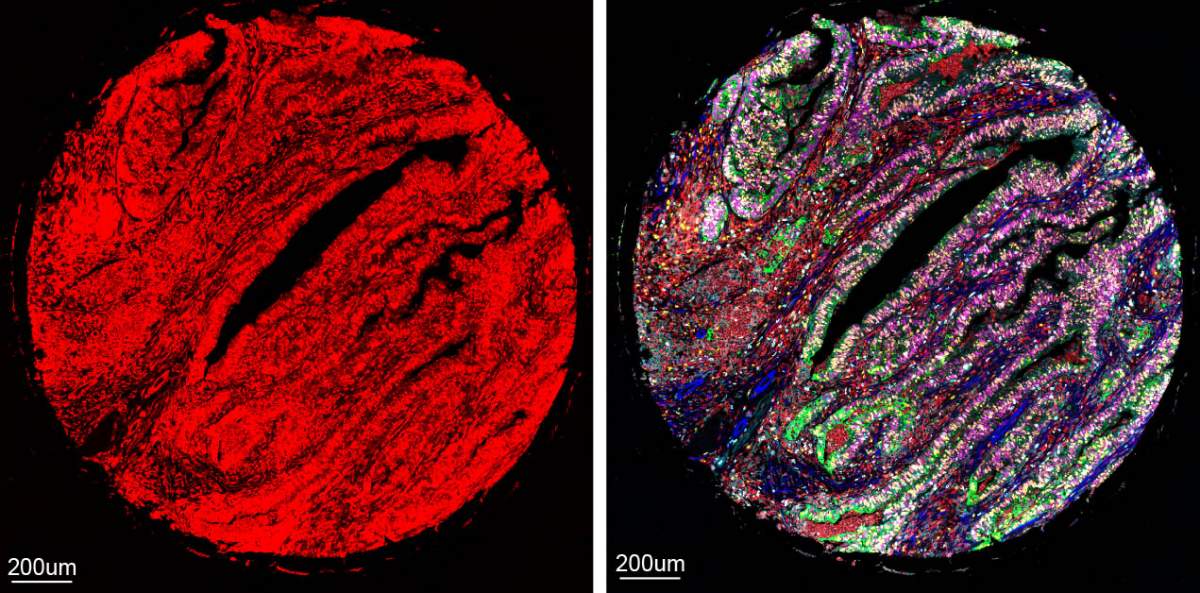
In search of the one-snap photo
The limitations of traditional methods stem from the optics of light. The fluorescent probes used to tag proteins have advanced protein studies enormously in the past few decades, but when several proteins in the same tissue sample are tagged with different-colored probes, the cellophane-like colors can overlap, muddling the microscope image. One way around this is to repeat the imaging several times, each time washing off the existing tags and placing new ones. But this approach, known as cyclic fluorescence, is time-consuming, and it makes it possible to eventually image only several dozen proteins at most - out of the myriad protein versions that can be produced by the 20,000 or so protein-coding genes in the human genome.
"We wanted to develop an imaging method that would capture more of them at once, so that we could eventually image them all," Keren says.
She offers a picture-taking metaphor to describe the essence of her mission. "Imagine that you wanted to get a snapshot of a room but were limited to photographing three or four objects at a time, say just tables, chairs and TV screens to start. By the time you got around to windows, rugs and standing lamps, you'd have taken dozens of photos and would then need to assemble them to get an approximate image of the room." Keren's goal is to provide a means of photographing the entire room, with the full complement of objects it contains, in a single shot.
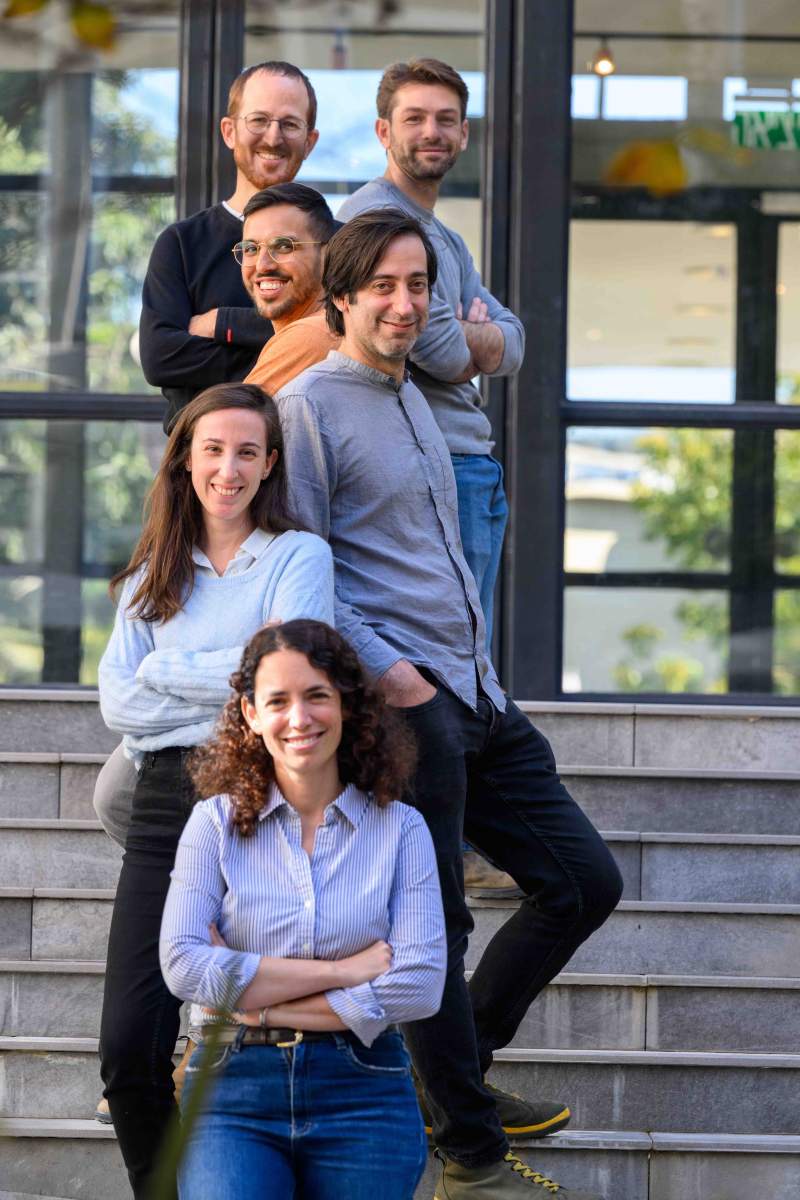
Reading overlapping proteins
Keren and her team decided to rely on what is known as a combinatorial approach: labeling each protein by attaching to it several fluorescent tags, so that each molecule is identified by a unique combination of fluorescent tags that create a barcode of sorts.
The advantage of combinations, or barcodes, is that they can vastly increase the number of molecules tagged by a small number of colors. If you want to image, for example, seven different molecules, they can be marked using only three colors because these three colors can come in numerous possible combinations. With more tags, the number of potential combinations increases exponentially, unlocking a larger palette of possibilities. However, if viewing multiple individual probes is complex, the problem becomes even more significant when viewing multiple barcodes. As the number of barcodes grows, they quickly start overlapping, making it impossible to distinguish among them.
Keren and her team had the idea that AI might be able to tackle the seemingly intractable problem of overlapping barcodes. They hypothesized that AI could do this by learning the expression properties of different proteins from images of tissues, since some proteins are expressed by only one type of cell, whereas others, by different cells; some are expressed on the cell surface, and some inside the cell; some are ubiquitous, while others are rare.
""To understand how any particular tissue works, it's crucial to measure lots of its proteins at the same time"
Amazingly enough, their hunch proved true. Working in collaboration with Dr. Shai Bagon of the Weizmann Center of Artificial Intelligence, Keren and her team of students - whose backgrounds ranged from biochemistry to bioinformatics to mathematics - set out to design both an experimental method that could measure multiple proteins at once and an AI algorithm that would tease apart images containing numerous protein barcodes. The team trained a deep neural network to tackle the challenge of breaking down combinatorial images into individual protein images. The network was trained on simulated data, generated by bringing together numerous fluorescent images of individual proteins produced in labs worldwide.
The result is CombPlex, an AI-assisted imaging method that accurately measures multiple proteins in a tissue sample on the level of individual cells. It can decompress pictures that appear to a human viewer like a nightmarish fluorescent tangle, sorting the signals into images of individual proteins.
Since CombPlex is compatible with conventional fluorescent microscopes widely used in research and clinics, it holds promise for greatly facilitating the study of tissues in biomedical and medical labs. In addition to providing a fuller picture of the tissue, it can - in a day or two - supply protein imaging that would take weeks to obtain with conventional approaches.
"We hope that CombPlex will one day replace regular pathology methods because it provides a more comprehensive look at tissues and enables more precise insights for clinical decision-making," Keren says.
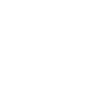
Science Numbers
In theory, CombPlex enables researchers to capture up to 2n-1 proteins using only n tags. This means that 3 tags can capture 7 proteins; 5 tags, 31 proteins; and 10 tags, 1,023 proteins, though real-life challenges can reduce these numbers. In the study, Keren's team was able to measure 22 proteins using barcodes comprising 5 different tags each.
The development of CombPlex was guided and supported by Bina , the Weizmann Institute's translational research unit that identifies early-stage projects with applicative potential. "When we consulted experts in the field, they were all really enthusiastic about this technology," says the head of Bina, Dr. Sharon Fireman.
The research team also included Raz Ben-Uri, Lior Ben Shabat, Dana Shainshein, Yuval Bussi, Noa Maimon, Dr. Tal Keidar Haran, Dr. Idan Milo and Dr. Ofer Elhanani of the Molecular Cell Biology Department; Omer Bar Tal of the Computer Science and Applied Mathematics Department; Drs. Inna Goliand, Yoseph Addadi and Tomer-Meir Salame of the Life Sciences Core Facilities Department; and Dr. Alexander Rochwarger and Prof. Christian M. Schuerch of University Hospital Tuebingen, Germany.
Dr. Leeat Yankielowicz-Keren's research is supported by the Dwek Institute for Cancer Therapy Research; the Schwartz Reisman Collaborative Science Program; the Abisch-Frenkel Foundation for the Promotion of Life Sciences; the Enoch Foundation Research Fund; Sharon Levine and Jon Corzine; the Rising Tide Foundation; and Fundación Alberto Palatchi.
Dr. Yankielowicz-Keren is the incumbent of the Fred and Andrea Fallek President's Development Chair.