Abstract
A new ultra-lightweight artificial intelligence (AI) model has been developed that assists in generating high-quality images without directly sending sensitive data to servers. This technological advancement paves the way for the safe utilization of high-performance generative AI in environments where privacy is paramount, such as in the analysis of patient MRI and CT scans.
A research team, led by Professor Jaejun Yoo from the Graduate School of Artificial Intelligence at UNIST has announced the development of PRISM (PRivacy-preserving Improved Stochastic Masking), a federated learning AI model.
Federated learning (FL) is a technique that allows for the creation of a global AI by compiling results from each device's local AI after conducting learning without needing to upload sensitive information directly to the server.
PRISM serves as an AI model that acts as a mediator connecting local AI with global AI during the federated learning process. This model reduces communication costs by an average of 38% compared to existing models, and its size is reduced to a 1-bit level, which allows it to operate efficiently on the CPUs and memory of small devices such as smartphones and tablets.
Moreover, PRISM accurately assesses which local AI's information to trust and incorporate, even in situations where there is significant variability in data and performance across different local AIs, resulting in high-quality generated outputs.
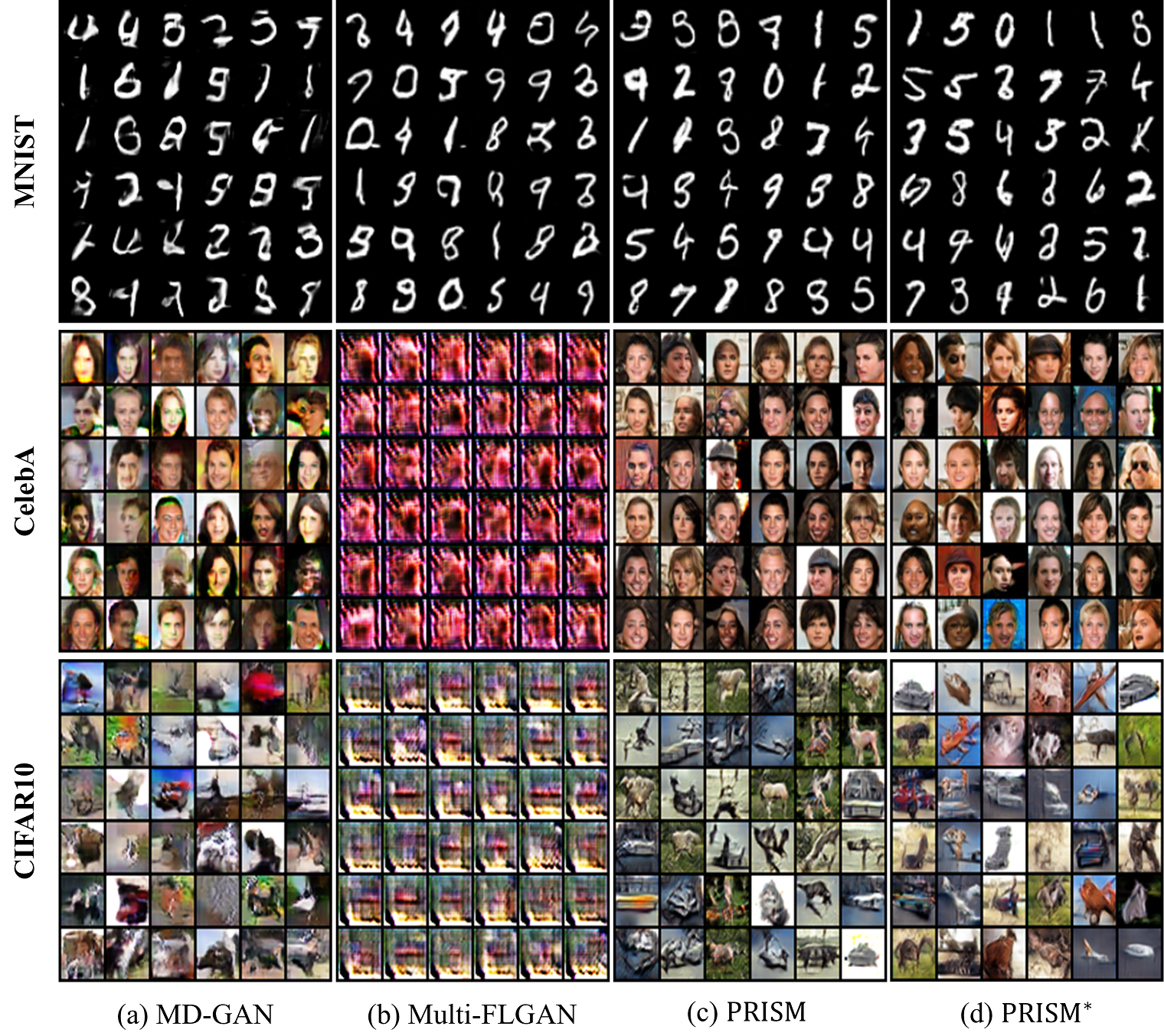
Figure 1. Qualitative results in IID scenario with a privacy budget.
For instance, when transforming a selfie into a Studio Ghibli-style image, previous methods required uploading the photo to a server, raising concerns about potential privacy breaches. With PRISM, all processing occurs on the smartphone, safeguarding personal privacy and enabling rapid results. However, it's important to note that developing the local AI model capable of generating images on the smartphone is a separate requirement.
Experimental results on datasets commonly used for validating AI performance, including MNIST, FMNIST, CelebA, and CIFAR10, demonstrated that PRISM not only reduced communication volume but also produced higher quality image generation compared to traditional methods. Notably, additional experiments using the MNIST dataset confirmed compatibility with diffusion models primarily used for generating Studio Ghibli-style images.
The research team enhanced communication efficiency by employing a stochastic binary mask method that selectively shares only critical information instead of vast parameter sharing. Furthermore, the use of Maximum Mean Discrepancy (MMD) for precise evaluation of generative quality and Mask-Aware Dynamic Aggregation (MADA) strategies that aggregate contributions from each local AI differently helped to mitigate data discrepancies and training instability.
Professor Yoo stated, "Our approach can be applied not only to image generation, but also to text generation, data simulation, and automated documentation, making it an effective and safe solution in fields dealing with sensitive information, such as healthcare and finance."
This research was conducted in collaboration with Professor Dong-Jun Han from Yonsei University, with UNIST researcher Kyeongkook Seo participating as the first author.
The research findings will be presented at the thirteenth International Conference on Learning Representations (ICLR 2025), one of the world's most prestigious conferences dedicated to artificial intelligence research, is scheduled to take place from April 24 to 28 in Singapore. This study has been supported by the National Research Foundation of Korea (NRF), Institute for Information and communication Technology Planning and evaluation (IITP), and UNIST Supercomputing Center.
Journal Reference
Kyeongkook Seo, Dong-Jun Han, Jaejun Yoo, "PRISM: Privacy-Preserving Improved Stochastic Masking for Federated Generative Models," ICLR 2025, (2025).