When older adults fall at home, every second counts - especially when they are alone.
New research from Binghamton University aims to cut reaction times with a human action recognition (HAR) algorithm that uses local computing power to analyze sensor data and detect abnormal movements without transmitting to a processing center offsite.
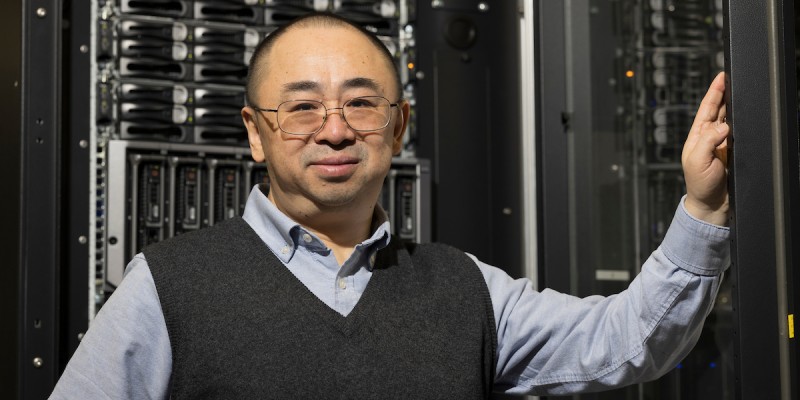
Professor Yu Chen and PhD student Han Sun from the Thomas J. Watson College of Engineering and Applied Science's Department of Electrical and Computer Engineering designed the Rapid Response Elderly Safety Monitoring (RESAM) system to leverage the latest advancements in edge computing.
In a paper recently published in the IEEE Transactions on Neural Systems and Rehabilitation Engineering, they show that the RESAM system can run using a smartphone, smartwatch, laptop or desktop computer with 99% accuracy and a 1.22-second response time, ranking among the most accurate methods available today.
Chen said the research is important for an underserved population: "When many people talk about high tech, they are discussing something cutting edge, like a fancier algorithm, a more powerful assistant to do jobs faster or having more entertainment available. We observed a group of people - senior citizens - who need more help but normally do not have sufficient resources or the opportunity to tell high-tech developers what they need."
By using devices already familiar to older people, rather than a full "smart home" setup, he thinks it gives them a better sense of control over their health. They don't need to learn new technology for the system to be effective.
Also, to protect people's privacy, RESAM reduces the monitored images to skeletons, which still allows analysis of key points such as arms, legs and torso to determine if someone has fallen or suffered a different accident that could lead to injury.
"The most dangerous place for falls is the bathroom, but nobody wants to set up a camera there," Chen said. "People would hate it."
He sees the RESAM system as a cornerstone for a wider concept he's calling "Happy Home," which could include thermal or infrared cameras and other sensors to remotely assess other aspects of a person's environment and well-being.
"Adding more sensors can make our system more powerful, because we are not only monitoring someone's body movements - we can monitor someone's health with one more dimension, so we better predict if something's going to happen before it happens," he said.
Another idea, which Chen is exploring with Associate Professor Shiqi Zhang from the Department of Computer Science, is for the system to include a robot dog or similar "pet" that would keep a closer watch as someone did their daily tasks. Last fall, Zhang demonstrated how a robot dog might guide someone with visual impairment through tugs on a leash.
"You could have a conversation with the robot," Chen said. "For example, when you are heading to the bathroom, the dog may ask you, 'Would you mind if I follow you?' The dog can make a better decision to move closer to monitor your status instead of having only fixed sensors in the room."