Neglected tropical diseases refer to a group of ailments that affect millions of people globally, often in poverty-stricken areas of the world, and yet don't receive the scientific attention they deserve. Schistosomiasis is one such disease – a persistent parasitic infection that affects an estimated 250 million people in 78 countries, particularly in Africa and Latin America.
Because schistosomiasis is understudied, improving diagnostic tools and treatments is often an afterthought. The current diagnostics available to test and treat for schistosomiasis don't always detect the infection in its early stages or when the infection is mild. And blood tests often cannot distinguish between active and past infections. Undiagnosed and left untreated, schistosomiasis can lead to serious bladder or liver complications.
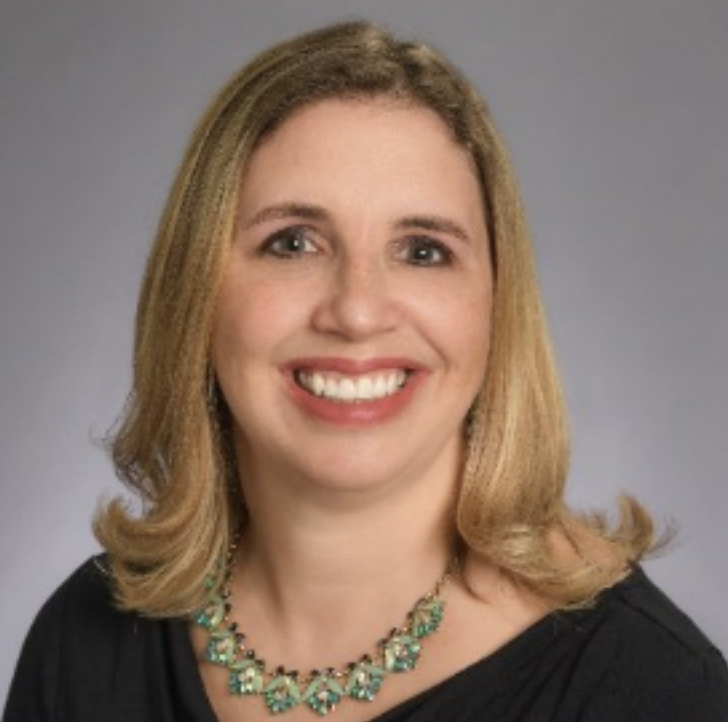
Prof. Jessica Fairley
Now, a team of researchers, including Jessica Fairley, an infectious disease specialist and associate professor in Emory University's School of Medicine, have found ways to detect schistosomiasis when other less sensitive tests cannot, leading to earlier treatment that can improve long term outcomes. Their findings, reported in Science Translational Medicine, show promise for developing a clinical antibody test that could quickly and easily catch even low levels of the infection. The research team used interpretable machine learning to separate individuals with active infection from those with past infection. Comparing healthy and infected individuals across two human cohorts from Brazil and Kenya revealed previously uncharacterized signatures of active disease, which can be used for more precise diagnosis.
A partnership between science and engineering
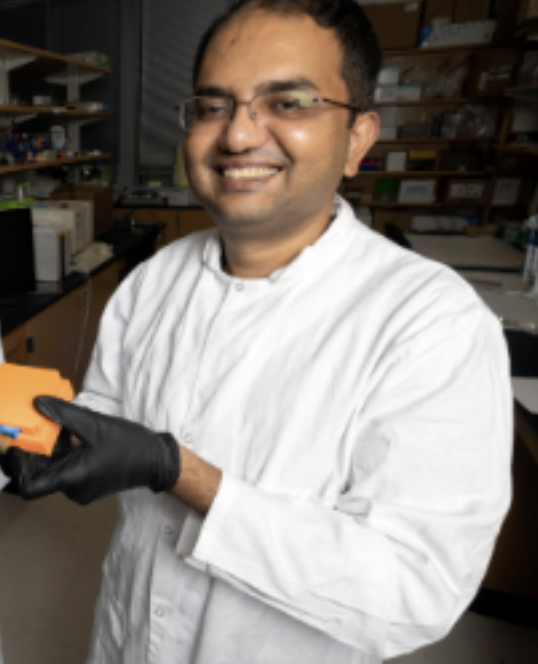
Prof. Aniruddh Sarkar
Aniruddh Sarkar, co-author on the paper and a biomedical engineer at Emory and the Georgia Institute of Technology, primarily focuses his research on fluid behavior at micro- and nano-scale on electronic chips. Since all biology takes place in fluids, and electronic chips function at the same microscopic scale at which cells and biomolecules exist, Sarkar partnered with Fairley to adapt his expertise "to probe biological processes at a very basic level," as he puts it. Fairley and Sarkar combined their knowledge of infectious disease and biological data analytics in a new and previously unrecognized way to diagnose schistosomiasis.
"The traditional gold standard is microscopic visualization of the eggs of the schistosoma parasite," Fairley says. "You look under a microscope, and it's very time intensive. It also can miss infection." By collaborating with Sarkar and machine learning specialist Jishnu Das at the University of Pittsburgh, another co-author on the paper, they were able to develop a machine learning platform that could identify groups of biomarkers for schistosomiasis that held the most insights into how the disease developed in particular patients. Sarkar says basing the diagnosis on qualities of groups of antibodies rather than quantity of a single marker will make it possible to do a better job of reliably detecting the disease early.
"When you look at these hundreds of measurements with just the human eye, even if you plot it with bar charts or pie charts, finding patterns can be challenging," Sarkar says. "It will seem like a jumble. That's where these technologies come in. It brings into focus the part of the data that really matters."
The researchers' eventual goal is to scale up the antibody test to the point where it could replace many other diagnostic techniques and be used quickly and easily in rural locations where schistosomiasis is often most prevalent.
Fairley is optimistic that the partnership between infectious disease knowledge and machine-aided data analysis can make a much larger contribution to public health.
"Introducing Jishnu and Aniruddh to the world of neglected infectious diseases allowed us to leverage their analytical expertise to create new diagnostics," says Fairley. "And this interdisciplinary partnership shows that we can give understudied diseases like schistosomiasis the attention they deserve and move the needle on improving global public health."
CITATION: "Deep humoral profiling coupled to interpretable machine learning unveils diagnostic markers and pathophysiology of schistosomiasis." Science Translational Medicine. 18 Sep 2024. Vol 16, Issue 765 DOI: 10.1126/scitranslmed.adk7832