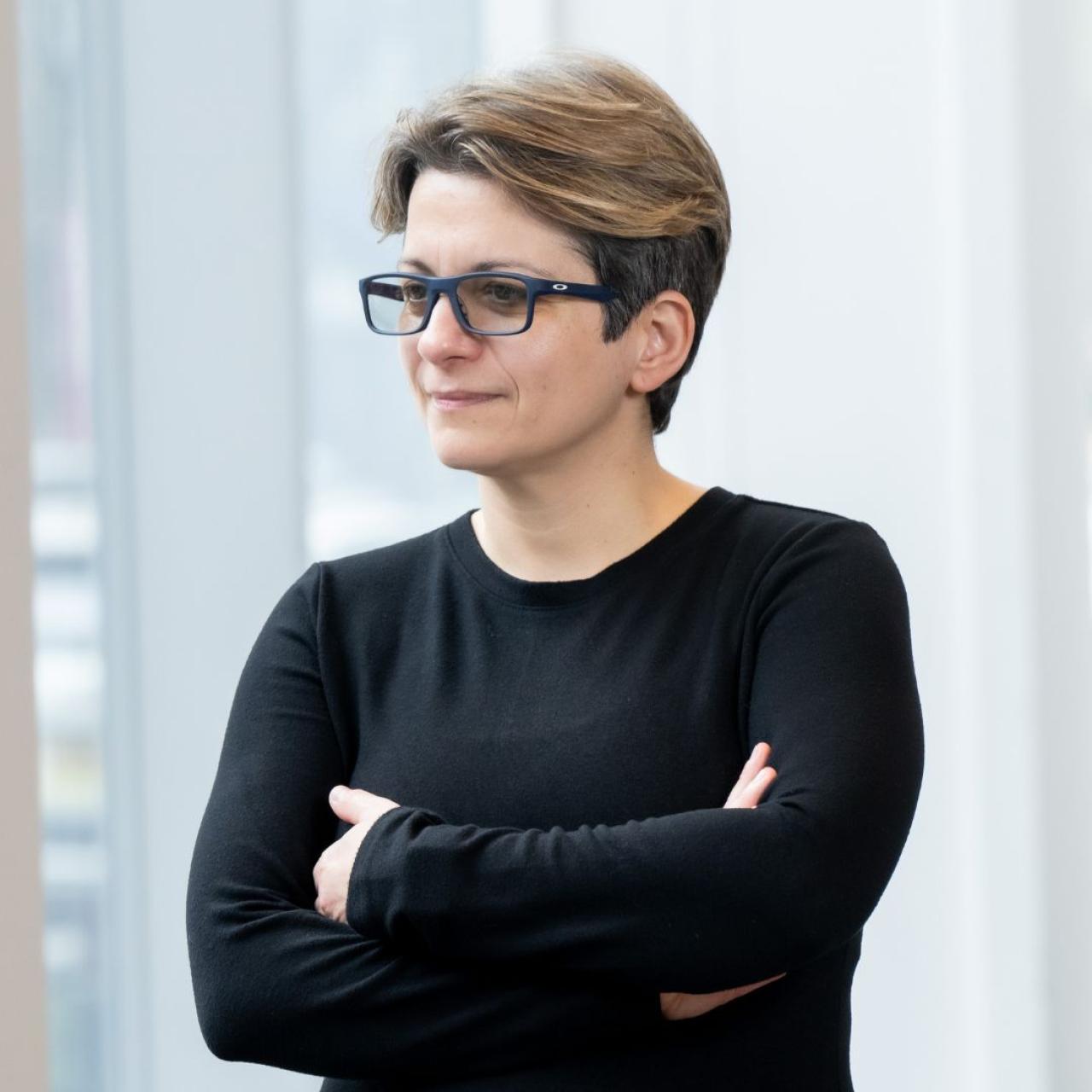
Despina Kontos, PhD is a leading researcher in artificial intelligence and data science. She joined CUIMC to spearhead the Center for Innovation in Imaging Biomarkers and Integrated Diagnostics (CIMBID), aiming to elevate it into a major hub for the clinical translation of AI tools.
Artificial intelligence (AI) is already changing the way medicine is practiced, enhancing and augmenting how clinicians work. One of the most promising areas for AI in healthcare is cancer imaging. Clinical opportunities for imaging AI span the patient's entire cancer journey, from risk assessment and cancer screening to disease detection and treatment response prediction.
"Eighty percent of the AI tools cleared for clinical use by the U.S. Food and Drug Administration are for imaging," said Despina Kontos, PhD, member of the Herbert Irving Comprehensive Cancer Center (HICCC) and vice chair of artificial intelligence and data science research in the Department of Radiology at Columbia University Irving Medical Center (CUIMC). "Radiology is leading the way on the translation of AI tools into the clinic, and here at Columbia, we're trying to capitalize on that momentum to really make an impact."
A computer scientist by training, Kontos pioneered the use of AI-based methods for breast cancer risk assessment with digital breast tomosynthesis, a 3D mammogram that takes multiple images of the breast from various angles. In January, she became founding director of the Center for Innovation in Imaging Biomarkers and Integrated Diagnostics (CIMBID), a new multidisciplinary center at Columbia dedicated to developing and integrating quantitative imaging and non-imaging biomarkers for disease prediction, particularly in cancer.
Enhanced screening protocols for breast cancer, such as shorter screening intervals and the addition of ultrasound or MRI to traditional mammography, are implemented in theory to detect the disease earlier. However, they also require additional resources, potentially overburdening the health system, and may result in higher false-positive rates, which impacts patients' wellbeing. One possible solution is switching from the universal screening recommendations used today to personalized screening regimens that are tailored to an individual patient's cancer risk.
Kontos envisions a world where physicians routinely incorporate image-derived data, such as digital mammograms, into risk assessment algorithms. For example, her work has found that AI-based analysis of mammographic parenchymal complexity - patterns formed by the distribution of fatty, fibroglandular, and stromal breast tissues - can predict breast cancer risk. The resulting risk profile, along with demographics and genetic information, will then be used to optimize screening frequency for each patient.
"I think cancer screening is going to be the first area that we're going to see some of these medical applications of AI," said Kontos, who also serves as director of biomarker imaging at NewYork-Presbyterian Hospital. "Down the road, AI in medical imaging is probably going to play an important role in the areas of prognostic assessment and therapy response evaluation for cancer."
She aims to leverage her new leadership role at the CIMBID to push forward the scientific front in cancer biomarkers for disease prediction. Through the vibrant ecosystem at Columbia that spans across departments and schools, Kontos plans to grow the center into a major hub for the clinical translation of AI tools.
However, to fully harness the power of AI in medicine, researchers and institutions must overcome considerable challenges involving infrastructure and data sharing. AI projects require high-performance computing infrastructure to work with and shuffle around massive amounts of data, all of which costs time, effort, and money.
"We have the ideas, in principle, and the population data, but it takes forever to figure out how to organize the data, load them on a computing environment, store them, analyze them, and share them between institutions," said Kontos. "That's what stops us from making the breakthrough contributions that can lead to change in patient care."
One idea for streamlining part of this process is federated learning with multi-institutional datasets. Instead of bringing the data to the algorithm through data sharing, which can be incredibly time- and resource-consuming, federated learning focuses on bringing the algorithm to the data. The algorithm is trained locally at each institution, and then the results are aggregated into a single model. For example, a 2022 study by Kontos and her colleagues on quantitative mammographic breast density estimation with neural networks demonstrated that federated learning can improve algorithm generalizability while maintaining patient privacy.
As the inaugural Chief Research Information Officer for CUIMC, Kontos will oversee the strategic planning and direction of resources devoted to research informatics, technology, and computing (RITC) across the medical center. With AI applications in mind, she hopes to upgrade CUIMC's RITC infrastructure to provide greater access to high-performance computing, data resources, and support services.
Kontos notes just how rapidly AI is advancing, and how academic centers such as Columbia will need to adapt to continue to be a part of the conversation and drive change for the greater good.
"In academia, we will never be Amazon or Google," she said. "I think we need to recognize that and be more open-minded about how we partner with industry. We both bring complementary expertise, and if we come together, we can make the breakthroughs that we need to push the field forward."