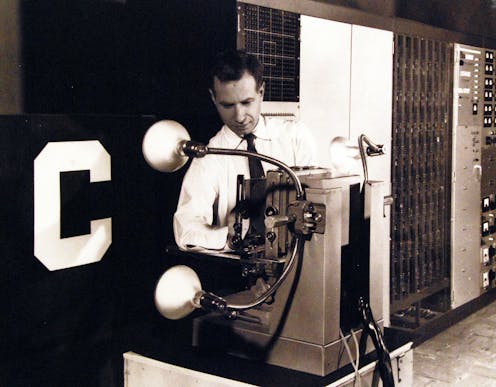
Many people think of psychology as being primarily about mental health, but its story goes far beyond that.
Authors
- Chris Ludlow
Lecturer in Psychology, Swinburne University of Technology
- Armita Zarnegar
Lecturer in Computer Science, Swinburne University of Technology
As the science of the mind, psychology has played a pivotal role in shaping artificial intelligence, offering insights into human cognition, learning and behaviour that have profoundly influenced AI's development.
These contributions not only laid the foundations for AI but also continue to guide its future development. The study of psychology has shaped our understanding of what constitutes intelligence in machines, and how we can address the complex challenges and benefits associated with this technology.
Machines mimicking nature
The origins of modern AI can be traced back to psychology in the mid-20th century. In 1949, psychologist Donald Hebb proposed a model for how the brain learns: connections between brain cells grow stronger when they are active at the same time.
This idea gave a hint of how machines might learn by mimicking nature's approach.
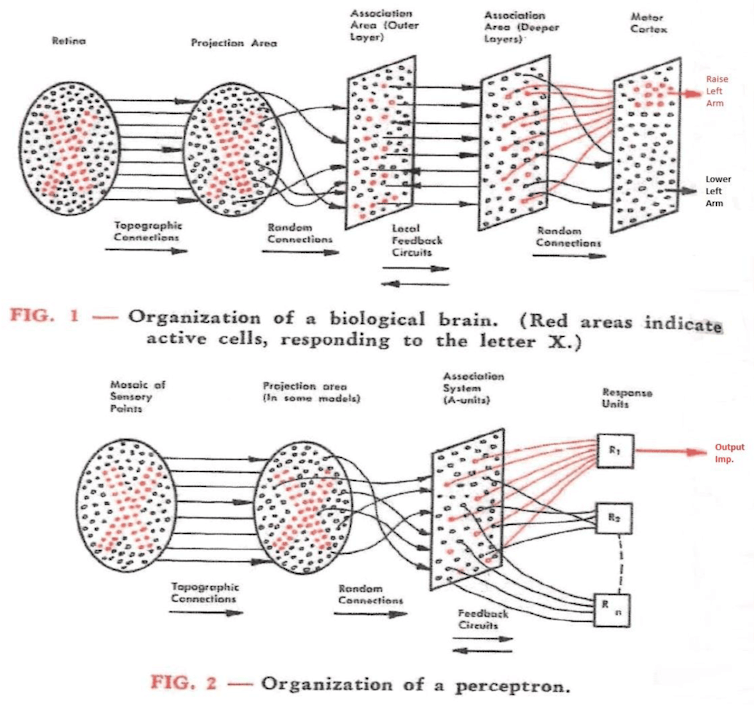
In the 1950s, psychologist Frank Rosenblatt built on Hebb's theory to develop a system called the perceptron .
The perceptron was the first artificial neural network ever made. It ran on the same principle as modern AI systems, in which computers learn by adjusting connections within a network based on data rather than relying on programmed instructions.
A scientific understanding of intelligence
In the 1980s, psychologist David Rumelhart improved on Rosenblatt's perceptron. He applied a method called backpropagation , which uses principles of calculus to help neural networks improve through feedback.
Backpropagation was originally developed by Paul Werbos, who said the technique "opens up the possibility of a scientific understanding of intelligence, as important to psychology and neurophysiology as Newton's concepts were to physics".
Rumelhart's 1986 paper , coauthored with Ronald Williams and Geoffrey Hinton , is often credited with sparking the modern era of artificial neural networks. This work laid the foundation for deep learning innovations such as large language models.
In 2024, the Nobel Prize for Physics was awarded to Hinton and John Hopfield for work on artificial neural networks. Notably, the Nobel committee, in its scientific report , highlighted the crucial role psychologists played in the development of artificial neural networks.
Hinton, who holds a degree in psychology, acknowledged standing on the shoulders of giants such as Rumelhart when receiving his prize.
Self-reflection and understanding
Psychology continues to play an important role in shaping the future of AI. It offers theoretical insights to address some of the field's biggest challenges, including reflective reasoning, intelligence and decision-making.
Microsoft founder Bill Gates recently pointed out a key limitation of today's AI systems. They can't engage in reflective reasoning, or what psychologists call metacognition.
In the 1970s, developmental psychologist John Flavell introduced the idea of metacognition. He used it to explain how children master complex skills by reflecting on and understanding their own thinking.
Decades later, this psychological framework is gaining attention as a potential pathway to advancing AI.
Fluid intelligence
Psychological theory is increasingly being applied to improve AI systems, particularly by enhancing their capacity for solving novel problems.
For instance, computer scientist François Chollet highlights the importance of fluid intelligence , which psychologists define as the ability to solve new problems without prior experience or training.
In a 2019 paper , Chollet introduced a test inspired by principles from cognitive psychology to measure how well AI systems can handle new problems. The test - known as the Abstract and Reasoning Corpus for Artificial General Intelligence (ARC-AGI) - provided a kind of guide for making AI systems think and reason in more human-like ways.
In late 2024, OpenAI's o3 model demonstrated notable success on Chollet's test, showing progress in creating AI systems that can adapt and solve a wider range of problems.
The risk of explanations
Another goal of current research is to make AI systems more able to explain their output. Here, too, psychology offers valuable insights.
Computer scientist Edward Lee has drawn on the work of psychologist Daniel Kahneman to highlight why requiring AI systems to explain themselves might be risky.
Kahneman showed how humans often justify their decisions with explanations created after the fact, which don't reflect their true reasoning. For example, studies have found that judges' rulings fluctuate depending on when they last ate - despite their firm belief in their own impartiality .
Lee cautions that AI systems could produce similarly misleading explanations. Because rationalisations can be deceptive, Lee argues AI research should focus on reliable outcomes instead.
Technology shaping our minds
The science of psychology remains widely misunderstood. In 2020, for example, the Australian government proposed reclassifying it as part of the humanities in universities.
As people increasingly interact with machines, AI, psychology and neuroscience may hold key insights into our future.
Our brains are extremely adaptable, and technology shapes how we think and learn. Research by psychologist and neuroscientist Eleanor Maguire , for example, revealed that the brains of London taxi drivers are physically altered by using a car to navigate a complex city.
As AI advances, future psychological research may reveal how AI systems enhance our abilities and unlock new ways of thinking.
By recognising psychology's role in AI, we can foster a future in which people and technology work together for a better world.
The authors do not work for, consult, own shares in or receive funding from any company or organisation that would benefit from this article, and have disclosed no relevant affiliations beyond their academic appointment.