Researchers used a chemical synthesis robot and computationally cost effective A.I. model to successfully predict and validate highly selective catalysts.
Artificial intelligence (A.I.) has made headlines recently with the advent of ChatGPT's language processing capabilities. Creating a similarly powerful tool for chemical reaction design remains a significant challenge, especially for complex catalytic reactions. To help address this challenge, researchers at the Institute for Chemical Reaction Design and Discovery and the Max Planck Institut für Kohlenforschung have demonstrated a machine learning method that utilizes advanced yet efficient 2D chemical descriptors to accurately predict highly selective asymmetric catalysts-without the need for quantum chemical computations.
"There have been several advanced technologies which can "predict" catalyst structures, but those methods often required large investments of calculation resources and time; yet their accuracy was still limited," said joint first author Nobuya Tsuji. "In this project, we have developed a predictive model which you can run even with an everyday laptop PC."
For a computer to learn chemical information, molecules are usually represented as a collection of descriptors, which often consist of small parts, or fragments, of those molecules. These are easier for A.I. to process and can be arranged and rearranged to construct different molecules, much like Lego pieces can be arranged and connected in different ways to construct different structures.
However, computationally cheaper 2D descriptors have struggled to accurately represent complex catalyst structures, leading to inaccurate predictions. To improve this issue, researchers developed new Circular Substructure (CircuS) 2D descriptors that explicitly represent cyclic and branched hydrocarbon structures, which are common in catalysis. Training data for the A.I. was obtained through experiments via a streamlined, semi-automatic process utilizing a synthesis robot. This experimental data was then converted into descriptors and used to train the A.I. model
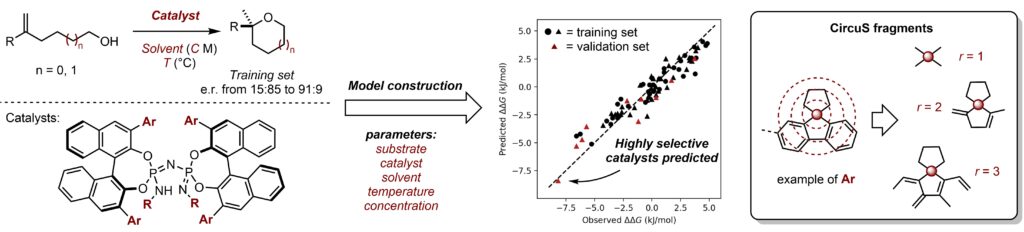
Fast and robust predictive models using 2D descriptors particularly suited for asymmetric catalysis. Highly selective catalysts were predicted and validated using training data with only moderate selectivities (Nobuya Tsuji, Pavel Sidorov, et al. Angewandte Chemie International Edition. January 23, 2023)
Researchers used the fully trained model to virtually test 190 catalysts not part of the training data. In this set, the A.I. model was able to predict highly selective catalysts after only having been trained on the data of catalysts with moderate selectivity, showing an ability to extrapolate beyond the training data. The catalyst predicted to have the highest selectivity was then tested experimentally, exhibiting a selectivity nearly identical to that predicted by the A.I. model. Obtaining high selectivity is especially crucial for the design of new medicines, and this technique provides chemists with a powerful framework for optimizing selectivity that is efficient in both computational and labor cost.
"Often, to predict new selective catalysts chemists would use models based on quantum chemical calculations. However, such models are computationally costly, and when the number of compounds and the size of molecules increases, their application becomes limited," commented joint first author Pavel Sidorov. "Models based on 2D structures are much cheaper and therefore can process hundreds and thousands of molecules in seconds. This allows chemists to filter out the compounds they may not be interested in much more quickly."
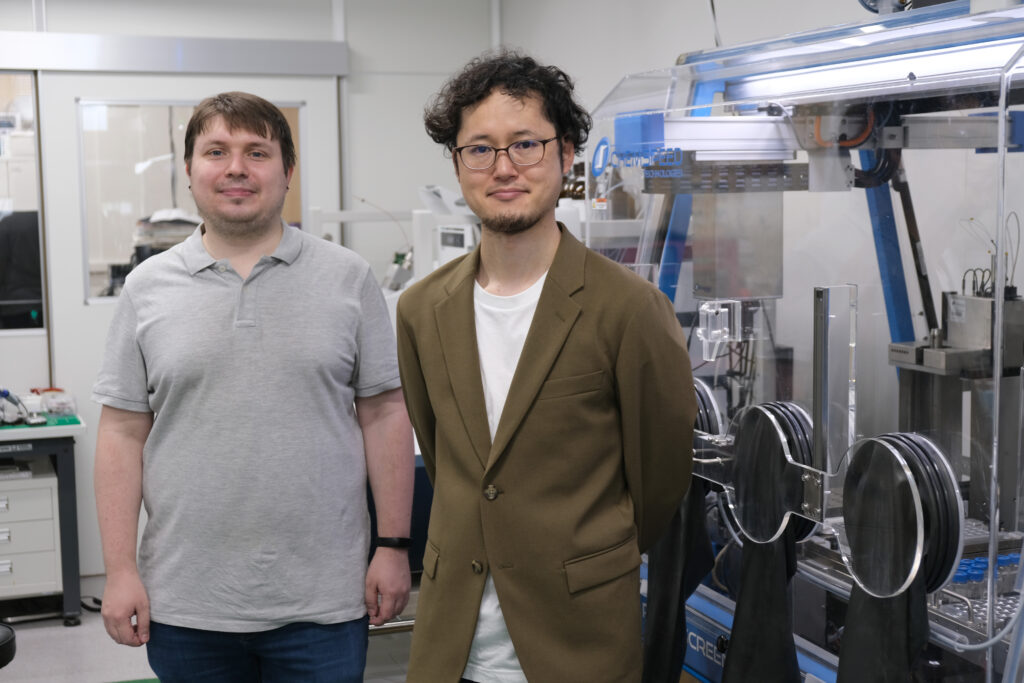
Pavel Sidorov (left) and Nobuya Tsuji (right) of the research team at the Institute for Chemical Reaction Design and Discovery (WPI-ICReDD), Hokkaido University, pictured next to the automated synthesis robot used in this study. (Photo Credit: ICReDD)
Original Article:
Nobuya Tsuji, Pavel Sidorov, Chendan Zhu, Yuuya Nagata, Timur Gimadiev, Alexandre Varnek, Benjamin List. Predicting Highly Enantioselective Catalysts Using Tunable Fragment Descriptors. Angewandte Chemie International Edition. January 23, 2023.
DOI: 10.1002/anie.202218659
Funding:
This work was financially supported by the Max Planck Society, the Deutsche Forschungsgemeinschaft (DFG, German Research Foundation, Leibniz Award to B.L.) and Germany's Excellence Strategy (EXC 2033-390677874-RESOLV), the European Research Council (ERC, European Union's Horizon 2020 research and innovation program "C−H Acids for Organic Synthesis, CHAOS," Advanced Grant Agreement No. 694228). This work was also financially supported by the Institute for Chemical Reaction Design and Discovery (ICReDD), which was established by the World Premier International Research Initiative (WPI), MEXT, Japan, and by the Japan Society for the Promotion of Science (JSPS) KAKENHI Grants 21H01925, 22K14672, 21H01924 and the Japan Science and Technology Agency Exploratory Research for Advanced Technology (JST-ERATO) grant JPMJER1903.