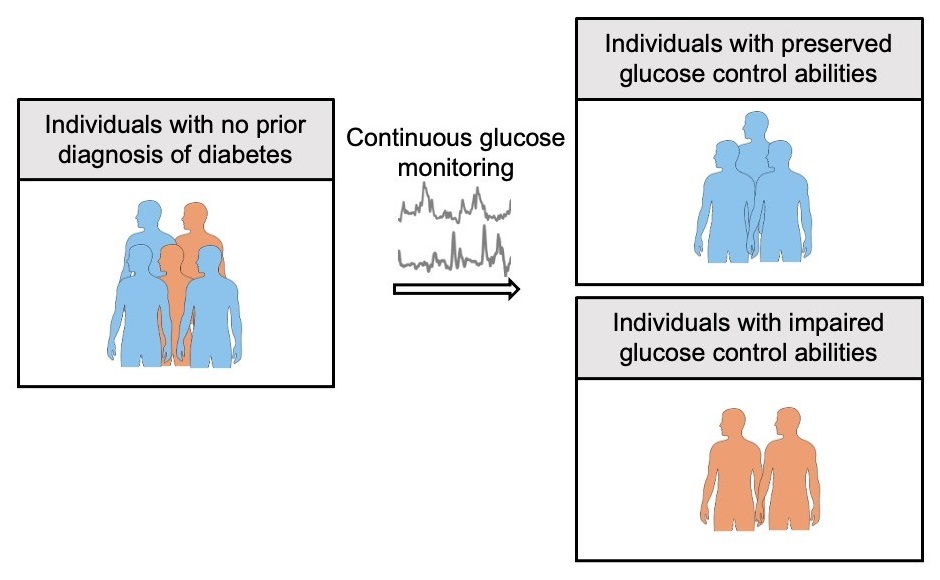
The highs and lows of blood glucose aren't just an energy rollercoaster; they could be a key to detecting diabetes risk early and spare you a needle prick or two.
Researchers at the University of Tokyo have identified a simple, noninvasive method for assessing blood glucose regulation — an essential factor in diabetes risk. Their approach, based on continuous glucose monitoring (CGM) data, could improve early detection and risk assessment for diabetes without relying on blood samples and expensive or complex procedures.
The study is published in Communications Medicine.
Often called a "silent epidemic," diabetes is an increasingly prevalent global health concern, with significant health and economic consequences. Early detection of impaired glucose regulation — an intermediate stage between normal blood glucose levels and diabetes — is essential for preventing or delaying the onset of Type 2 diabetes. Conventional diagnostic tools, however, often miss early signs because they rely on periodic blood samples rather than continuous monitoring.
"Traditional diabetes tests, while useful, do not capture the dynamic nature of glucose regulation under physiological conditions," said Shinya Kuroda, a professor at the University of Tokyo's Graduate School of Science and co-author of the current study.
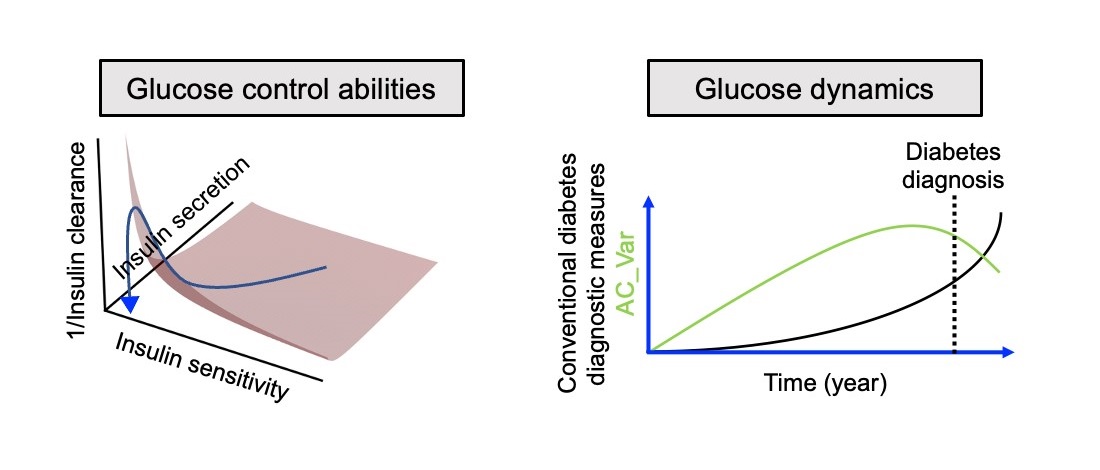
To find a more practical alternative, the team turned to CGM, a wearable technology that continuously tracks glucose levels in real time, providing a clearer picture of blood glucose fluctuations in everyday life. Their goal was to identify a CGM-based method for estimating glucose handling capacity, which maintains stable levels, without invasive procedures.
The team analyzed 64 individuals without a prior diabetes diagnosis, using a CGM device, oral glucose tolerance tests (OGTT), and clamp tests that are used to assess insulin sensitivity and glucose metabolism. They then validated their findings with an independent dataset and mathematical simulations.
Their analysis showed that AC_Var, a measure of glucose-level fluctuations, strongly correlates with the disposition index, a well-established predictor of future diabetes risk. Moreover, the researchers' model, which combines AC_Var with glucose standard deviation, outperformed traditional diabetes markers — such as fasting blood glucose, HbA1c and OGTT results — in predicting the disposition index.
"By analyzing CGM data with our new algorithm, we identified individuals with impaired glycemic control — even when standard diagnostic tests classified them as 'normal,'" said Kuroda. "This means we can potentially detect issues much earlier, creating an opportunity for preventive interventions before diabetes is diagnosed."
The team also showed that the method was more accurate than conventional diagnostic indicators in predicting diabetes complications such as coronary artery disease. To facilitate broader access to this approach, the research team has developed a web application that allows individuals and health care providers to easily calculate these CGM-based indices.
"Our ultimate goal is to provide a practical, accessible tool for widespread diabetes screening," Kuroda said. "By enabling early detection of glucose regulation abnormalities, we hope to prevent or delay disease onset and reduce long-term complications."