Researchers at Tohoku University and the University of California, Santa Barbara, have developed new computing hardware that utilizes a Gaussian probabilistic bit made from a stochastic spintronics device. This innovation is expected to provide an energy-efficient platform for power-hungry generative AI.
As Moore's Law slows down, domain-specific hardware architectures - such as probabilistic computing with naturally stochastic building blocks - are gaining prominence for addressing computationally hard problems. Similar to how quantum computers are suited for problems rooted in quantum mechanics, probabilistic computers are designed to handle inherently probabilistic algorithms. These algorithms have applications in areas like combinatorial optimization and statistical machine learning. Notably, the 2024 Nobel Prize in Physics was awarded to John Hopfield and Geoffrey Hinton for their groundbreaking work in machine learning.
Probabilistic computers have traditionally been limited to binary variables or probabilistic bits (p-bits), making them inefficient for continuous-variable applications. Researchers from the University of California, Santa Barbara, and Tohoku University have now extended the p-bit model by introducing Gaussian probabilistic bits (g-bits). These complement p-bits with the ability to generate Gaussian random numbers. Like p-bits, g-bits serve as a fundamental building block for probabilistic computing, enabling optimization and machine learning with continuous variables.
One machine learning model that benefits from g-bits is the Gaussian-Bernoulli Boltzmann Machine (GBM). g-bits allow GBMs to run efficiently on probabilistic computers, unlocking new opportunities for optimization and learning tasks. For example, present-day generative AI models, such as diffusion models - widely used to create realistic images, videos, and text - rely on computationally expensive iterative processes. g-bits enable probabilistic computers to handle these iterative stages more efficiently, reducing energy consumption and speeding up the generation of high-quality outputs.
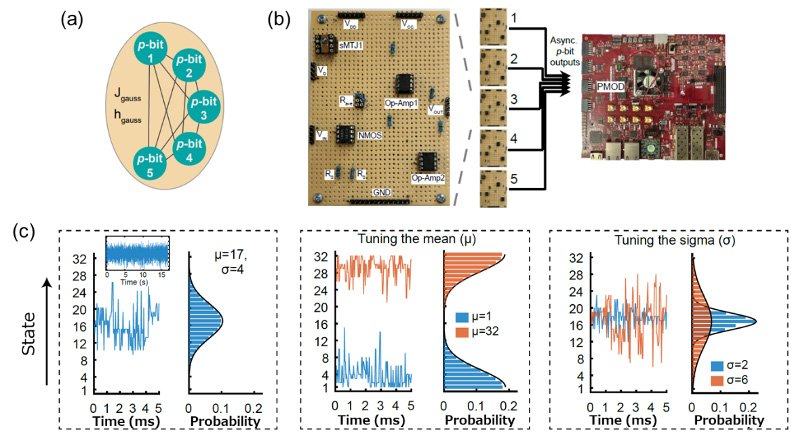
Other potential applications include portfolio optimization and mixed-variable problems, where models must process both binary and continuous variables. Conventional p-bit systems struggled with such tasks because they are inherently discrete and required complex approximations to handle continuous variables, leading to inefficiencies. By combining p-bits and g-bits, these limitations are overcome, enabling probabilistic computers to address a much broader range of problems directly and effectively.
- Publication Details:
Title: Beyond Ising: Mixed Continuous Optimization with Gaussian Probabilistic Bits using Stochastic MTJs
Authors: Nihal Sanjay Singh, Corentin Delacour, Shaila Niazi, Kemal Selcuk, Daniel Golenchenko, Haruna Kaneko, Shun Kanai, Hideo Ohno, Shunsuke Fukami and Kerem Y. Camsari
Conference: 70th Annual IEEE International Electron Devices Meeting