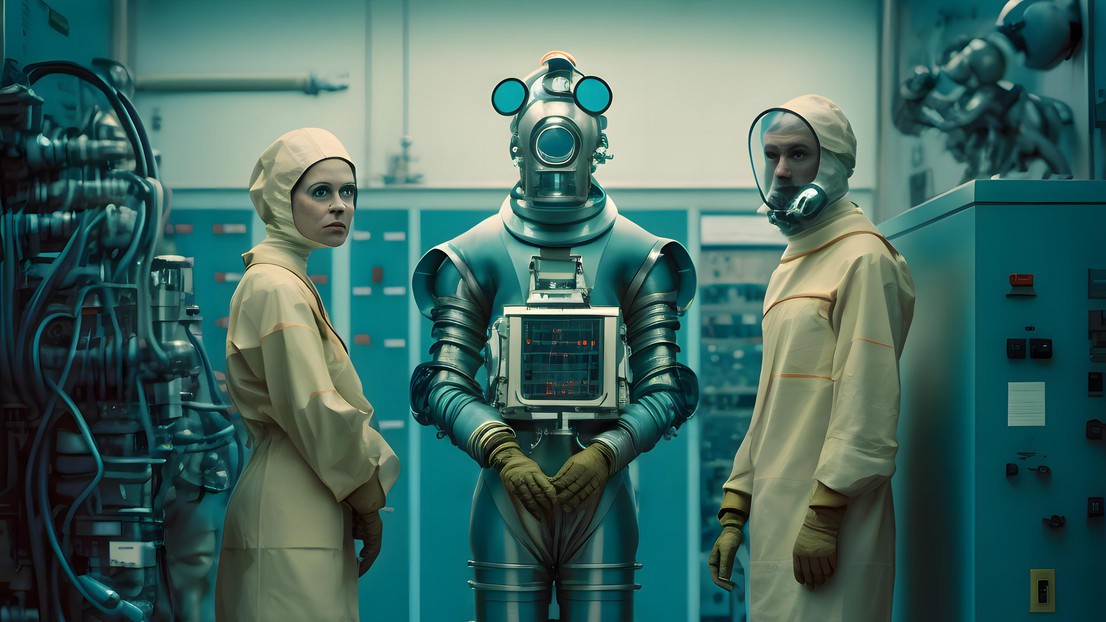
© Illustration created with "Midjourney" by Alexandre Sadeghi
AI chatbot ChatGPT exploded onto the scene in November 2022. It can write essays, WordPress plugins and even pass an MBA exam - yet scientists still don't fully understand how these general artificial intelligence programs work. New EPFL research aims to change this paradigm.
This article is an excerpt from : Artificial intelligence: friend or foe?
Within a few weeks of Open AI's ChatGPT being unleashed on the world, it reached 100 million users, making it the fastest-growing consumer application in history. Two months later, Google announced the release of its own Bard A.I. The day after, Microsoft said it would incorporate a new version of GPT into Bing. These powerful general artificial intelligence programs will affect everything from education to people's jobs - leading to Open AI CEO Sam Altman's claim that general artificial intelligence will lead to the downfall of capitalism.
So, is this a lot of marketing hype or is it the beginning of AI changing the world as we know it? "It's unclear what this means right now for humanity, for the world of work and for our personal interactions," says EPFL Assistant Professor Robert West in the School of Computer and Communications Sciences.
West's work is in the realm of natural language processing, done with neural networks, which he describes as the substrate on which all of these general artificial intelligence programs run. In the past few years, industry focus has been on making these bigger and bigger with exponential growth. GPT-3 (ChatGPT's core language model) has 175 billion neural network parameters, while Google's PaLM has 540 billion. It's rumored that GPT-4 will have still more parameters.
The size of these neural networks means that they are now able to do things that were previously entirely inconceivable. Yet in addition to the ethical and societal implications of these models, training such massive programs also entails major financial and environmental impacts. In 2020, Danish researchers estimated that the training of GPT-3, for example, required the amount of energy equivalent to the yearly consumption of 126 Danish homes, creating a carbon footprint the same as driving 700,000 kilometers by car.
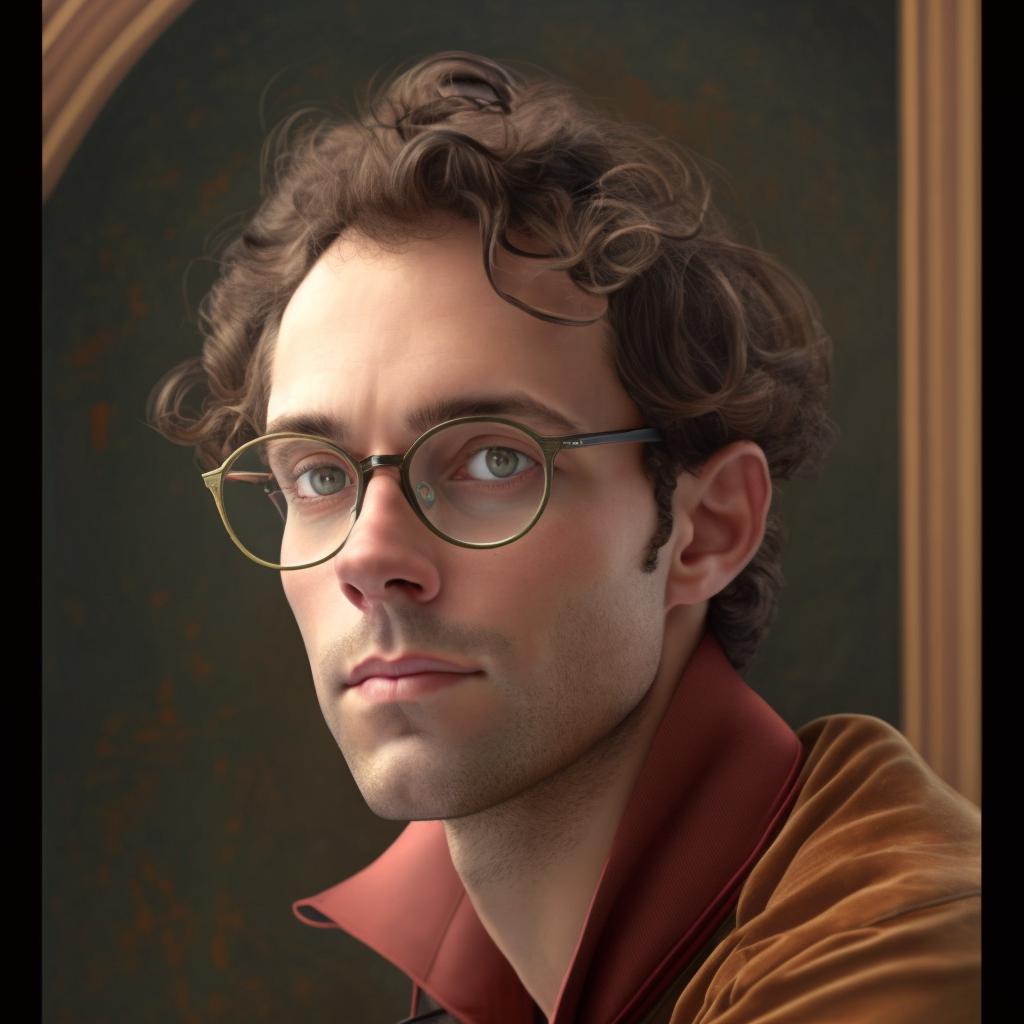
Stirring the whole broth every time
"A cutting-edge model such as GPT-3 needs all its 175 billion neural network parameters just to add up two numbers, say 48 + 76, but with 'only' 6 billion parameters it does a really bad job and in 90% of cases it doesn't get it right," says West.
"The root of this inefficiency is that neural networks are currently what I call a primordial soup. If you look into the models themselves, they're just long lists of numbers, they're not structured in any way like sequences of strings or molecules or DNA. I see these networks as broth brimming with the potential to create structure," he continues.
West's Data Science Lab was recently awarded a CHF 1.8 million Swiss National Science Foundation Starting Grant to do just this. One of the research team's first tasks will be to address the fundamental problem of turning the models' hundreds of billions of unstructured numbers into crisp symbolic representations,using symbolic auto encoding.
"In today's language models like GPT-3, underlying knowledge is spread across its primordial soup of 175 billion parameters. We can't open up the box and access all the facts they have stored, so as humans we don't know what the model knows unless we ask it. We can't therefore easily fix something that's wrong because we don't know what's wrong," West explains. "We will be taking self-supervised natural language processing from text to knowledge and back, where the goal is to propose a new paradigm for an area called neuro-symbolic natural language processing. You can think of this as taming the raw power of neural networks by funneling it through symbolic representations."
On-chip neuroscience
West argues that this approach will unlock many things for next-level AI that are currently lacking, including correctability - if there is a wrong answer it's possible to go into the symbol and change it (in a 175-billion parameter soup it would be difficult to know where to start); fairness - it will be possible to improve the representation of facts about women and minorities because it will be possible to audit information; and, interpretability - the model will be able to explain to humans why it arrived at a certain conclusion because it has explicit representations.
Additionally, such a model will be able to introspect by reasoning and combining facts that it already knows into new facts, something that humans do all the time. It will memorize facts and forget facts by just deleting an incorrect entry from its database, which is currently very difficult.
"When trying to understand current state-of-the-art models like GPT-3 we are basically conducting neuroscience, sticking in virtual probes to try to understand where facts are even represented. When we study something that's out there in nature we are trying to understand something that we didn't build, but these things have never left our computers and we just don't understand how they work."
Revamping Wikipedia
The final part of the research will demonstrate the wide applicability of these new methods - putting them into practice to revolutionize Wikipedia. To support the volunteer editors, West's new model will try to tackle key tasks and automate them, for example, correcting and updating stale information and synchronizing this knowledge across all the platform's 325 languages.
West also sees important financial and environmental benefits from the research work his team is undertaking. "In academia, we don't have the resources of the private sector, so our best bet is to shift the paradigm instead of just scaling up the paradigm that already exists," he explains. "I think this is where we can save computational resources by being smarter about how we use what we have - and this is a win-win. Industry can take our methods and build them into their own models to eventually have more energy efficient models that are cheaper to run."
For better or worse, it's clear that with the public release of ChatGPT, the genie is out of the bottle. However we navigate the very real challenges of the future, with general artificial intelligence models advancing at a pace that comes as a surprise to many, West remains positive and finds his work exciting as he sees it as helping to break communication barriers between humans, but also between humans and machines.
"This is a starting point. It's already technically challenging, but it's really only a stepping stone towards having something that can perpetually self-improve with many other benefits."