Here is the ultimate vision of systems biology: an entire living cell modeled on a computer. Type in a genetic mutation or the chemical formula for an experimental drug, and the virtual cell immediately shifts its biology in response, giving researchers valuable insight into what might occur in the human body and replacing slow, tedious lab experiments.
Columbia's Mohammed AlQuraishi says such an AI-powered cell simulation might be less than 15 years away, and he is working to make it a reality. Today, AlQuraishi's lab focuses mostly on using machine learning approaches to predict how proteins fold into complex structures-a challenge which stymied biologists for decades. But as he refines tools to do this, he looks toward the future.

Mohammed AlQuraishi says the use of AI to predict biology is moving at the speed of light. Photo provided by Columbia University Herbert Irving Comprehensive Cancer Center.
"If we can predict the structure of molecules, then we can next predict how molecular machines assemble. Next, we predict the motion and function of those machines, and we keep building our way up until we've captured the entire complexity of the cell," says AlQuraishi, assistant professor of systems biology at the Vagelos College of Physicians and Surgeons, and a member of Columbia's Program for Mathematical Genomics. "This would completely change how we study disease and design drugs."
Cellular programming
As an undergraduate at Santa Clara University, AlQuraishi intended to launch a career in computer science; he had always loved programming. But a few biology classes showed him that, like computers, living cells contained codes that executed logic and programs.
"What really attracted me to biology was this idea that you have a code written in another language that nobody fully understands," says AlQuraishi. "Protein structure, in particular, struck me as this prism through which to view all of biology."
So he changed his plans, earning a second bachelors degree in biology after he had graduated with a computer science degree, and then studying genetics at Stanford. In graduate school there, he modeled how protein and DNA molecules interact with each other. In the mid-2010s, as a systems biology fellow at Harvard Medical School, AlQuraishi returned to the question that had first drawn him in to biology: can we predict how a protein folds?
A newly formed protein is like a long, straight strand of spaghetti which must fold, origami-like, into a complex molecule. Predicting a protein's final shape, based only on the sequence of building blocks that composed it, was a long-time challenge.
Protein folding for the masses
Most researchers were trying to create detailed rule books of the physics of protein folding and use those rules to write long, complex computer programs that could simulate the process. The technique was slow-going at best.
AlQuraishi took another approach: he turned to an emerging type of artificial intelligence known as deep learning. With deep learning models-such as today's popular ChatGPT-a program is given massive amounts of data and finds it own patterns.
"At this time, most biologists had no idea what deep learning was, but it was pretty clear to me that this was uniquely suited to the problem of protein folding prediction," AlQuraishi says.
AlQuraishi and his Harvard colleagues developed an early AI-powered protein folding predictor, but it was quickly supplanted by Google's AlphaFold, first released in 2018 with later versions in 2020 and 2024. As powerful as AlphaFold was (the team that developed it won the 2024 Nobel Prize in Chemistry), AlQuraishi still saw room for his own continued research and development.
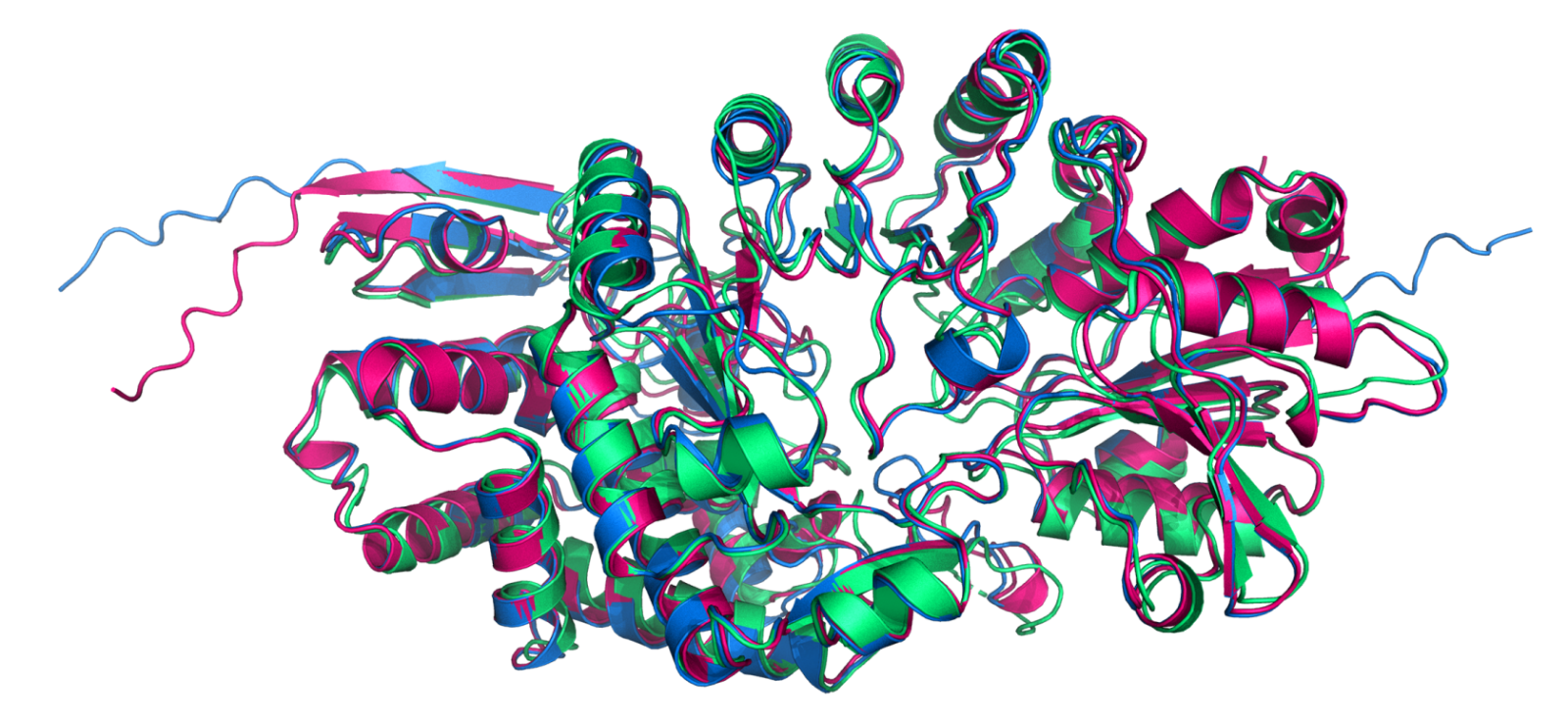
A newly formed protein is like a long, straight strand of spaghetti which must fold, origami-like, into a complex molecule. Mohammed AlQuraishi's group has created OpenFold, which uses AI to predict a protein's final shape based on its sequence of building blocks. This image compares the performance of OpenFold (pink) and AlphaFold (blue) to the protein's experimentally determined structure (green). Image provided by Mohammed AlQuraishi.
"AlphaFold did well for individual proteins but it worked less well for protein complexes, larger assemblies, and mutant proteins," said AlQuraishi. "At the same time, there are technical and legal limitations that mean individual labs can't train it on new data or add new functionality."
In response, AlQuraishi's group developed OpenFold-an open-access program that he says has with the same performance as AlphaFold 2 but not the same limitations. Researchers around the globe quickly flocked to OpenFold and began using it in new and diverse ways, such as adding different types of experimental results to strengthen the predictions of the program. Today, AlQuraishi and his collaborators are working on a new version of OpenFold to match the capabilities of AlphaFold3.
The power of AI
As new types of data are integrated into OpenFold and its successors-data on how proteins interact with each other, for instance-AlQuraishi believes that it will gain the ability to make increasingly complex types of predictions. That's where his vision for an entire simulated cell emerges from.
"I think we can't ever be certain that we understand all the essential features of a living cell unless we can model it," he says. "And then suddenly, we will have this incredible tool that we can probe in all sorts of ways."
He recently adjusted his estimate of when this kind of tool might debut; for years, he estimated 2050. Now, he guesses 2040.
"This field is moving at the speed of light," he said. "I encourage people to step back and look at how far we've come in the last few years and try to imagine what things will look like five or 10 years from now. Because I think many people don't appreciate how quickly and massively things are evolving."